1. Introduction
As deep learning of computer vision technology becoming more and more developed and popular, the application of this technology significantly increases. Deep learning simulates our brain, helping systems learn to identify objects and perform complex tasks with increasing accuracy without human intervention. This technology has several great advantages compared to the traditional manual working. Because of the growing computing abilities of computers, computers can achieve much more precision and do extremely intricate work. For example, deep learning in computer vision field plays an important role in solving some problems related to the invention of high-level autonomous driving which puzzled scientists for many years. In my study field, The semantic segmentation is a key concept or a key technique in computer vision deep learning project and thanks to it we can create many useful models to achieve various of functions. Generally speaking, semantic segmentation of characters involves identifying the content, ensuring the location of objects present in the images and classifying them into different groups according to your label criteria. Here is a intuitive example related to the concept of semantic segmentation.
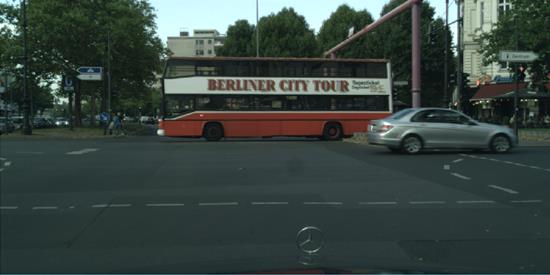
Figure 1. The original image of street image in CityScapes Dataset[2]
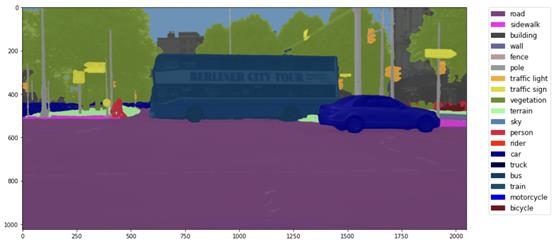
Figure 2. The result of semantic segmentation produced by Segformer model[3]
As we can tell, in the result image, the model classifies different objects with different colors, such as labeling car object with deep blue and labeling those trees with light green. The semantic segmentation model in remote sensing can achieve similar effect and produce similar results as the following example.
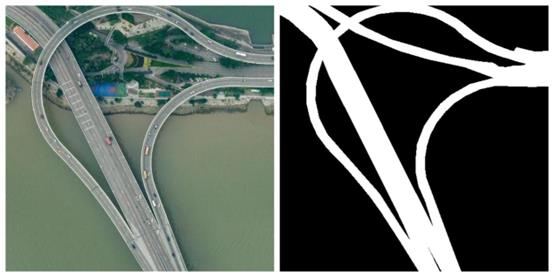
Figure 3. The comparison between original image and label image in road extraction
According to the upper pictures in CHN6 Dataset[4], we can know that an appropriate semantic segmentation model can extract roads with white color based on the studying of original image and the label image and researcher’s goal is to enhance the accuracy of extracting roads as much as possible. Until now, there are many extraordinary scientists manage to create useful model in extracting roads. The models invented by them can be used in a variety of situations, including villages, large cities or harbors. However, for most of those models, according to the following table, the accuracy is about 70 percent.
Table 4. Accuracy of road extraction by using FCN-based Algorithm.
Algorithm |
Methods |
Precision |
Patch-based DCNN |
Mnih et al. |
0.901 |
Patch-based DCNN |
Saito et al. |
0.905 |
Patch-based DCNN |
Ventura et al. |
0.835 |
Patch-based DCNN |
Alshehhi et al. |
0.917 |
FCN-based |
Zhong et al. |
0.710 |
DeconvNet-based |
Wei et al. |
0.606 |
DeconvNet-based |
Panbooyuen et al. |
0.854 |
DeconvNet-based |
Panbooyuen et al. |
0.858 |
DeconvNet-based |
Zhang et al. |
0.919 |
DeconvNet-based |
Gao et al. |
0.851 |
GAN-based |
Costea et al. |
0.841 |
GAN-based |
Shi et al. |
0.883 |
Graph-based |
Ventura et al. |
0.835 |
Graph-based |
Lian et al. |
0.823 |
This accuracy is not high enough in precisely extracting roads from the original images compared to labeling by human, although it will cost less. Many models fail to get high extraction accuracy because under some unconventional and special situations, it is extremely hard to correct classify roads apart from the background.
For this passage, in contrast, the model trained by myself with 40,000 iterations can achieve high accuracy which is about 83 percent under the situations of large cities. I select 234 original Beijing remote sensing images and adjust corresponding labeling images to the appropriate form one by one then train a particular model by utilizing transferring learning based on a effective fully convolutional neural network UNet[1]. Undoubtedly, it will cause over-fitting by only using the images from Beijing. But this model can perform great precision and work pretty well in large cities situations.
2. Methodology
2.1. The basic principle
According to an essay named Road Extraction Methods in High-Resolution Remote Sensing Images: A Comprehensive Review[5], the dataset need to contain a set of original images. Then encoder the original images to the correct labeling format and classify the whole data into two group -- train group and validation group(There is no test group in here because of the small scale of data pictures). The model can gradually learn the traits of road extraction by backward learning those images. The following graph gives an nice example of this process.
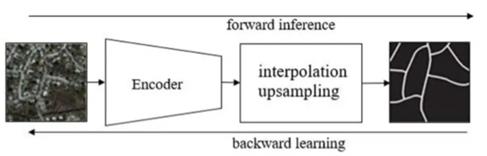
Figure 5. Process of training model related to road extraction by using deep learning5
By using forward inference and backward learning through fully convolutional network, a semantic segmentation model with high accuracy is obtained specifically for extracting roads from remote sensing maps in large cities.
2.2. The pretreatment of the datasets
For the dataset I used, it is called CHN6-CUG Chinese Road Dataset[4]. This dataset mainly covers urban areas in China, including six Chinese cities: Chaoyang District in Beijing, Yangpu District in Shanghai, Wuhan City Center, Nanshan District in Shenzhen, Sha Tin in Hong Kong, and Macau. I randomly select 234 images from city Beijing in order to train my Beijing-oriented road extraction model. Those images are originally RGB mode and they need to be modified to gray-scale mode. Besides, in the coding part, the road color (255,255,255) corresponds to the subscript 1 of the category list. Those data images are changed to the proper form by using a high-quality software named photoshop. The photoshop can automatically identify the white region of the image and change its color to annotation format precisely. Thus, a series of high precision annotation images can be summarized which contributes to the high accuracy of the model significantly.
2.3. Trained process and adjust parameters
I rented a 3090ti graphics card on the FEATURIZE platform for training and used Jupyterlab to code and train the model. For the coding part, I referenced a professional master’s code and adjust some important parts based on the original code. Data set path was linked to the program, own segmentation category and corresponding RGB colors were added in the program, the corresponding pipeline file was generated, and train.py program was called in the terminal. Before the training, the original images and labeling images were randomly cropped and rotated in order to increase model’s robustness. During the training process, the program will output some important variables corresponding to the accuracy of model estimation, such as mIoU and the loss. A table which contains mIoU, accuracy, precision and other factors will be printed for each 500 times.
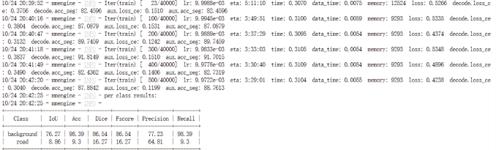
Figure 6. Some output variables during the training process
For the parameters, the total iterations is 40,000 times. The batch_size is 4 and the model I used it UNet. After completing the training process, a JSON log file will be saved in a specific directory. This JSON log file will contains changes information of various parameters during the training process, such as mIoU, Precision, and Loss. The trend of changes in these parameters can help us judge the performance of the model and better adjust parameters to improve the model. After each time, I adjusted the batch_size until the graphics card reaches its ultimate capacity. Due to the limitation of performance and quantity of the graphics card, the maximum batch_size I can use is about 4. Under the batch_size of 4, the model reaches its highest accuracy and lowest loss.
2.4. Visualize training results
An diagram related the loss value to the number of iterations has been created by using the matplotlib. The program will also create a diagram related the value of accuracy to the number of iterations and two diagrams related the accuracy of extraction of each subject to the number of iterations. Those diagrams will be shown in the result part of this article.
3. Result and discussion
3.1. Changing in loss value during the train process
A diagram related the loss value to the number of iterations was created by the matplotlib base on the corresponding json log file. By studying this diagram, we can know how well the model gradually understand the traits of original images to do the road extraction.
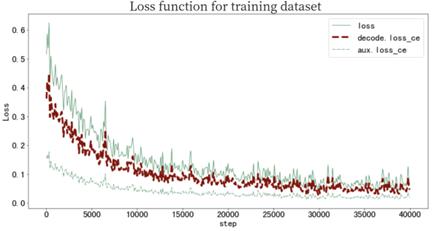
Figure 7. Diagram related loss value to the number of iterations
The green line which is above the red line represents the loss value during the training process. By focusing on the green line, we can argue that when the number of iterations is within 1 to 20,000, the loss value of the model continuously and significantly decreases; When the number of iterations is between 20000 and 40000, the loss value of the model remains stable and become more compacted. This trend shows that the model is understanding the traits of extracting roads gradually thus the loss is getting smaller and smaller. Besides, in the end of the training, the value of loss doesn’t grow up again. That means there is no overfitting problem in this training part. In conclusion, because the loss value is thoroughly decreasing throughout the training process which means the difference between model output and actual results is decreasing, the training was successful and the model started to know the traits of road extraction from the dataset I uploaded.
3.2. Changing in accuracy value during the training process
A diagram related the accuracy of extracting roads to the number of iterations was also created by the matplotlib based on the json log file of the training process. Based of this diagram, we can get a better understanding to judge whether the model successfully learn something new about extracting roads from its basic abilities. Here is the diagram.
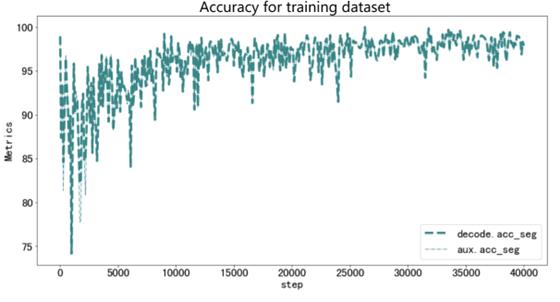
Figure 8. Diagram related the accuracy to the number of iterations
As we can see through this diagram, when the number of iterations is within 1-25000, the accuracy of the model on the training set continues to increase and significantly improves; When the number of iterations is between 25000 and 40000, the accuracy of the model on the training set does not significantly increase, but remains stable. The increasing period shows that the model definitely learned something new about the road extraction since the accuracy is increasing significantly. The stable period indicates that the model had fully learned the traits of extracting roads through the dataset. Both of them show that the model get a pretty well understanding on extracting roads and the final accuracy can reach about 97% which is extremely high. All in all, we can imply that the training was successfully and the model indeed learned something new about the road extraction based on its foundation abilities.
3.3. Evaluation variables for road subject
To further understand the model’s performance on extracting roads from the background on the original images on the testing dataset, a diagram related some evaluative variables to the number of iterations on road subject was created based on the json log file. By researching this diagram, we can test the model’s performance after the model was training by the train dataset. It is just like the application of the model to some more difficult and realistic situations and within that process we can evaluate the performance of the model.
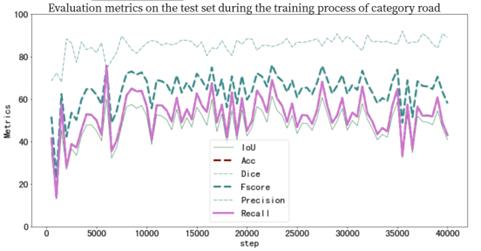
Figure 9. Diagram related some evaluation variables to the iterations on testing dataset
Through this diagram, when the number of iterations is between 1-15000, all evaluation variables (IoU, Acc, Dice, Fscore, Precision, Recall) of the category road on the testing set increases slightly. At 15000 to 40000 times, the semantic segmentation accuracy of the model on the testing set tends to stabilize at around 85%. Those data show that the model gets a better understanding of road extraction during the first 15000 times and reaches its ultimate and get a stable performance after 15,000 iterations. However, all evaluation variable except precision experienced a decreasing around 35,000 iterations. This may caused by a overfitting, showing that the model had already fully understood the dataset and occurred a little bit overfitting.
3.4. Compared with the common FCN-based model’s precision
The precision of the common fully convolutional network accuracy is about 71%. However, for the model I created, the precision on the testing dataset can reach about 83% which is greatly higher than 71%. For the training dataset, the accuracy can reach about 95% which is extremely high. This model can provide a high-accuracy road extraction image based on the original images under the large cities situations. Unless the model is used in environment instead of large cities, it will give a perfect performance which can help the map-maker to extract roads in large cities.
4. Conclusion
4.1. Brief summary and achievement
Compare to the results and analysis, we can generate conclusions. In this scientific research, a model which can extract roads in large cities environment with pretty high accuracy was trained through transfer learning based on UNet[1] with remote sensing original images and labeling images from Beijing. It solves the research gap which is the lack of high-accuracy road extracting model and can promote further research to further enhance its accuracy. The accuracy of this model is about 83% in testing dataset and 95% in training dataset. Both of them are higher than the previous model with great amount. Talking about its applications, by using the model, map-makers can extract and digitalize the roads in large cities and update them in time conveniently and quickly. High-accuracy remote sensing images which contain the digitized roads can also play an important role in auto pilot because auto pilot sometimes need them to assist to make various decisions in autonomous driving, such as lane changing and turning more precisely. As a result, this model has a wide application prospects.
4.2. Limitation analysis and future work
For the first limitation, due to the issue of time cost on labeling, the dataset size of the project is not large enough (only 234 images in all). Moreover, there are not enough categories in the dataset. Currently, only remote sensing images from Beijing have been used, and remote sensing map images from other major cities in China, such as Shanghai, have not been used. So, it may cause overfitting which shows that this model can only be used large cities, especially Beijing.
For the second limitation, because some optimization methods in deep learning are too difficult for me and require a long time to learn, currently a little optimization methods have been added to the training, only the dataset has been pre-processed. Moreover, due to the long duration of a single training session, the times of changing the parameters used for training is only 3. 83% accuracy in testing dataset and 95% accuracy in training dataset is the best result I get until now.
In the future, more dataset, including categories and amounts, need to be added to the training process of the model. Moreover, having more times of adjusting parameters and adding more optimization methods can significantly improve the performance of the model in testing dataset. That is also the work I need to do in the future. In sum, the research still has limitations and can be improved through using various methods and consider more variables.
References
[1]. Ronnebergere O, Fischer P, Brox T. U-Net: Convolutional Networks for biomedical image segmentation. arXiv. 2015 May 8; arXiv:1505.04597. Available from: https://arxiv.org/abs/1505.04597 doi:10.48550/arXiv.1505.04597
[2]. M Cordts, M Omran, S Ramos, T Rhefield, M Enzweiler, R Benenson, et al. The Cityscapes Dataset for semantic urban scene understanding. arXiv. 2014 Apr 6; arXiv:1604.01685. Available from: https://arxiv.org/abs/1604.01685 doi:10.48550/arXiv.1604.01685
[3]. Xie E, Wang W H, Yu Z D, A Anandkumar, M Alvarez J, Luo P. SegFormer: simple and efficient design for semantic segmentation with transformers. arXiv. 2021 Oct 28; arXiv:2105.15203. Available from: https://arxiv.org/abs/2015.15023 doi:10.48550/arXiv.2105.15203
[4]. Zhu Q Q. CHN6-CUG Chinese road dataset [Internet]. 2021 [cited 2023 Nov 20]. Available from: https://grzy.cug.edu.cn/zhuqiqi/zh_CN/index.html
[5]. Lian R B, Wang W X, Mustafa N, Huang L Q. Road extraction methods in high-resolution remote sensing images: a comprehensive review. IEEE Xplore. 2020 Sep 11; 13:5489-5507. Available from: https://ieeexplore.ieee.org/document/9195124 doi:10.1109/JSTARS.2929.3023549
Cite this article
Xie,S. (2024). A road semantic segmentation system for remote sensing images based on deep learning. Applied and Computational Engineering,64,15-22.
Data availability
The datasets used and/or analyzed during the current study will be available from the authors upon reasonable request.
Disclaimer/Publisher's Note
The statements, opinions and data contained in all publications are solely those of the individual author(s) and contributor(s) and not of EWA Publishing and/or the editor(s). EWA Publishing and/or the editor(s) disclaim responsibility for any injury to people or property resulting from any ideas, methods, instructions or products referred to in the content.
About volume
Volume title: Proceedings of the 6th International Conference on Computing and Data Science
© 2024 by the author(s). Licensee EWA Publishing, Oxford, UK. This article is an open access article distributed under the terms and
conditions of the Creative Commons Attribution (CC BY) license. Authors who
publish this series agree to the following terms:
1. Authors retain copyright and grant the series right of first publication with the work simultaneously licensed under a Creative Commons
Attribution License that allows others to share the work with an acknowledgment of the work's authorship and initial publication in this
series.
2. Authors are able to enter into separate, additional contractual arrangements for the non-exclusive distribution of the series's published
version of the work (e.g., post it to an institutional repository or publish it in a book), with an acknowledgment of its initial
publication in this series.
3. Authors are permitted and encouraged to post their work online (e.g., in institutional repositories or on their website) prior to and
during the submission process, as it can lead to productive exchanges, as well as earlier and greater citation of published work (See
Open access policy for details).
References
[1]. Ronnebergere O, Fischer P, Brox T. U-Net: Convolutional Networks for biomedical image segmentation. arXiv. 2015 May 8; arXiv:1505.04597. Available from: https://arxiv.org/abs/1505.04597 doi:10.48550/arXiv.1505.04597
[2]. M Cordts, M Omran, S Ramos, T Rhefield, M Enzweiler, R Benenson, et al. The Cityscapes Dataset for semantic urban scene understanding. arXiv. 2014 Apr 6; arXiv:1604.01685. Available from: https://arxiv.org/abs/1604.01685 doi:10.48550/arXiv.1604.01685
[3]. Xie E, Wang W H, Yu Z D, A Anandkumar, M Alvarez J, Luo P. SegFormer: simple and efficient design for semantic segmentation with transformers. arXiv. 2021 Oct 28; arXiv:2105.15203. Available from: https://arxiv.org/abs/2015.15023 doi:10.48550/arXiv.2105.15203
[4]. Zhu Q Q. CHN6-CUG Chinese road dataset [Internet]. 2021 [cited 2023 Nov 20]. Available from: https://grzy.cug.edu.cn/zhuqiqi/zh_CN/index.html
[5]. Lian R B, Wang W X, Mustafa N, Huang L Q. Road extraction methods in high-resolution remote sensing images: a comprehensive review. IEEE Xplore. 2020 Sep 11; 13:5489-5507. Available from: https://ieeexplore.ieee.org/document/9195124 doi:10.1109/JSTARS.2929.3023549