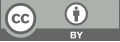
Application of machine learning optimization in cloud computing resource scheduling and management
- 1 Executive Master of Business Administration,Amazon Connect Technology Services (Beijing),Xi’an, Shaanxi, China
- 2 Software Engineering,Zhejiang University
- 3 Computer & Information Technology,Northern Arizona University
- 4 Information Studies,Trine University
- 5 Computer Information Technology,Northern Arizona University
- 6 Electronics & Communication Engineering,University of Electronic Science and Technology of China
* Author to whom correspondence should be addressed.
Abstract
In recent years, cloud computing has been widely used. Cloud computing refers to the centralized computing resources, users through the access to the centralized resources to complete the calculation, the cloud computing center will return the results of the program processing to the user. Cloud computing is not only for individual users, but also for enterprise users. By purchasing a cloud server, users do not have to buy a large number of computers, saving computing costs. According to a report by China Economic News Network, the scale of cloud computing in China has reached 209.1 billion yuan.Rational allocation of resources plays a crucial role in cloud computing. In the resource allocation of cloud computing, the cloud computing center has limited cloud resources, and users arrive in sequence. Each user requests the cloud computing center to use a certain number of cloud resources at a specific time.
Keywords
Cloud computing, Resource scheduling, Machine learning optimization, Artificial intelligence
[1]. X. Mo and J. Xu, “Energy-efficient federated edge learning with joint communication and computation design,” Journal of Communications and Information Networks, vol. 6, no. 2,pp. 110–124, 2021.
[2]. Q. Zeng, Y. Du, K. Huang, and K. K. Leung, “Energy-efficient resource management for federated edge learning with cpu-gpu heterogeneous computing,” IEEE Transactions on Wireless Communications, vol. 20, no. 12, pp. 7947–7962, 2021.
[3]. Hussain H, Malik S U R, Hameed A, et al. A Survey on Resource Allocation in High Performance Distributed Computing Systems[J]. Parallel Computing (S0167-8191), 2013, 39(11): 709-736.
[4]. Bellendorf J, Mann Z Á. Classification of Optimization Problems in Fog Computing[J]. Future Generation Computer Systems (S0167-739X), 2020, 107(1): 158-176.
[5]. Brogi A, Forti S, Guerrero C, et al. How to Place Your Apps in the Fog: State of the Art and Open Challenges[J]. Software: Practice and Experience (S0167-739X), 2019, 1(1): 1-8.
[6]. Wu C, Li W, Wang L, et al. Hybrid Evolutionary Scheduling for Energy-efficient Fog-enhanced Internet of Things[J]. IEEE Transactions on Cloud Computing (S2168-7161), 2018, 1(1): 1-1.
[7]. Atzori L, Iera A, Morabito G. The Internet of Things: A Survey[J]. Computer Networks (S1389-1286), 2010, 54(15): 2787-2805.
[8]. Bonomi F, Milito R, Natarajan P, et al. Fog Computing: A Platform for Internet of Things and Analytics. N. Bessis, C. Dobre. Big Data and Internet of Things: A roadmap for smart environments[M]. Cham: Springer, 2014, 546: 169-186.
[9]. Xiaoxi Zhang, Jianyu Wang, Li-Feng Lee, Tom Yang, Akansha Kalra,Gauri Joshi, Carlee Joe-Wong, “Machine Learning on Volatile Instances:Convergence, Runtime, and Cost Trade-offs”, IEEE/ACM Transactions on Networking, 30(1):215—228, 2022
[10]. Yichen Ruan, Xiaoxi Zhang, Shu-Che Liang,Carlee Joe-Wong, "Towards Flexible Device Participation in Federated Learning for Non-IID Data", International Conference on Artificial Intelligence and Statistics(AISTATS), 2021.
[11]. Yichen Ruan, Xiaoxi Zhang, Carlee Joe-Wong, “How Valuable Is Your Data? Optimizing Device Recruitment in Federated Learning”, submitted to ToN,prelimianary results are published in WiOpt 2021.
Cite this article
Zhang,Y.;Liu,B.;Gong,Y.;Huang,J.;Xu,J.;Wan,W. (2024). Application of machine learning optimization in cloud computing resource scheduling and management. Applied and Computational Engineering,64,9-14.
Data availability
The datasets used and/or analyzed during the current study will be available from the authors upon reasonable request.
Disclaimer/Publisher's Note
The statements, opinions and data contained in all publications are solely those of the individual author(s) and contributor(s) and not of EWA Publishing and/or the editor(s). EWA Publishing and/or the editor(s) disclaim responsibility for any injury to people or property resulting from any ideas, methods, instructions or products referred to in the content.
About volume
Volume title: Proceedings of the 6th International Conference on Computing and Data Science
© 2024 by the author(s). Licensee EWA Publishing, Oxford, UK. This article is an open access article distributed under the terms and
conditions of the Creative Commons Attribution (CC BY) license. Authors who
publish this series agree to the following terms:
1. Authors retain copyright and grant the series right of first publication with the work simultaneously licensed under a Creative Commons
Attribution License that allows others to share the work with an acknowledgment of the work's authorship and initial publication in this
series.
2. Authors are able to enter into separate, additional contractual arrangements for the non-exclusive distribution of the series's published
version of the work (e.g., post it to an institutional repository or publish it in a book), with an acknowledgment of its initial
publication in this series.
3. Authors are permitted and encouraged to post their work online (e.g., in institutional repositories or on their website) prior to and
during the submission process, as it can lead to productive exchanges, as well as earlier and greater citation of published work (See
Open access policy for details).