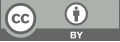
Composing jazz music pieces using LSTM neural networks approach
- 1 School of Computer Science and Engineering, South China University of Technology, Guangzhou 510641, China
* Author to whom correspondence should be addressed.
Abstract
The domain of artificial intelligence has increasingly extended into the creative arts, aiming to emulate and augment human creativity with automated processes. This is particularly evident in the field of music, where AI's ability to learn and produce intricate compositions has attracted significant attention. This study explores the challenge of generating jazz music using artificial intelligence, specifically focusing on the application of Long Short-Term Memory (LSTM) neural networks for jazz composition. By optimizing the model to address the genre's complexity, the research demonstrates the LSTM's capacity to capture and reproduce jazz's essential harmonic progressions and rhythmic nuances. Quantitative analyses show high accuracy and a deep understanding of musical structures, whereas qualitative feedback confirms the model's efficacy in producing compositions that embody jazz's spontaneity. Despite its achievements, the model's tendency to generate repetitive sequences suggests areas for improvement. This paper advances the field of AI in music, illustrating the potential of LSTM networks to mimic complex musical genres and emphasizing the necessity of ongoing model refinement to foster creativity. It highlights the evolving role of machine learning in music generation, proposing a foundation for future work aimed at diminishing the gap between AI capabilities and artistic expression.
Keywords
LSTM networks, jazz music, MIDI, model refinement
[1]. Zhu Y, Baca J, Rekabdar B, et al. 2023 A Survey of AI Music Generation Tools and Models arXiv preprint arXiv: 2308.12982.
[2]. Huang Q, Park D S, Wang T, et al. 2023 Noise2music: Text-conditioned music generation with diffusion models arXiv preprint arXiv: 2302.03917.
[3]. Dai S, Yu H and Dannenberg R B. 2022 What is missing in deep music generation? a study of repetition and structure in popular music arXiv preprint arXiv: 2209.00182.
[4]. Briot J P, Hadjeres G, Pachet F D 2017 Deep learning techniques for music generation--a survey arXiv preprint arXiv: 1709.01620.
[5]. Ji S, Luo J, Yang X 2020 A comprehensive survey on deep music generation: Multi-level representations, algorithms, evaluations, and future directions arXiv preprint arXiv:2011.06801.
[6]. Briot J P and Pachet F 2017 Music generation by deep learning-challenges and directions arXiv preprint arXiv:1712.04371.
[7]. Liang M 2022 Mobile Information Systems vol 2022 p 7618045.
[8]. Ghojogh B and Ghodsi A 2023 Recurrent neural networks and long short-term memory networks: Tutorial and survey arXiv preprint arXiv: 2304.11461.
[9]. Huang Y S and Yang Y H 2020 Pop music transformer: Generating music with rhythm and harmony arXiv preprint arXiv: 2002.00212 3.
[10]. Yang X, Yu Y, Wu X 2022 Applied Sciences vol 12(10) p 5050.
[11]. Hsiao W Y, Liu J Y, Yeh Y C, et al. 2021 Proceedings of the AAAI Conference on Artificial Intelligence vol 35(1) pp 178-186.
[12]. Peng B, Narayanan S and Papadimitriou C 2024 On Limitations of the Transformer Architecture arXiv preprint arXiv:2402.08164.
Cite this article
Zhang,X. (2024). Composing jazz music pieces using LSTM neural networks approach. Applied and Computational Engineering,68,128-136.
Data availability
The datasets used and/or analyzed during the current study will be available from the authors upon reasonable request.
Disclaimer/Publisher's Note
The statements, opinions and data contained in all publications are solely those of the individual author(s) and contributor(s) and not of EWA Publishing and/or the editor(s). EWA Publishing and/or the editor(s) disclaim responsibility for any injury to people or property resulting from any ideas, methods, instructions or products referred to in the content.
About volume
Volume title: Proceedings of the 6th International Conference on Computing and Data Science
© 2024 by the author(s). Licensee EWA Publishing, Oxford, UK. This article is an open access article distributed under the terms and
conditions of the Creative Commons Attribution (CC BY) license. Authors who
publish this series agree to the following terms:
1. Authors retain copyright and grant the series right of first publication with the work simultaneously licensed under a Creative Commons
Attribution License that allows others to share the work with an acknowledgment of the work's authorship and initial publication in this
series.
2. Authors are able to enter into separate, additional contractual arrangements for the non-exclusive distribution of the series's published
version of the work (e.g., post it to an institutional repository or publish it in a book), with an acknowledgment of its initial
publication in this series.
3. Authors are permitted and encouraged to post their work online (e.g., in institutional repositories or on their website) prior to and
during the submission process, as it can lead to productive exchanges, as well as earlier and greater citation of published work (See
Open access policy for details).