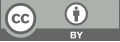
Data job requirement characteristics analysis based on large language model and K-means clustering
- 1 Beijing Language and Culture University
- 2 Beijing Language and Culture University
- 3 Beijing Language and Culture University
- 4 Beijing Language and Culture University
* Author to whom correspondence should be addressed.
Abstract
This paper aims to analyze the characteristics of data-related job requirements using a Large Language Model (LLM), providing decision support for the construction of data-related courses in universities. Using Zhaopin.com as the data source and employing a key attribute extraction method based on ChatGLM3 along with prompting engineering techniques, this study extracts and clusters key attributes from job descriptions. It analyzes the characteristics of data-related job requirements in terms of competencies, fields of expertise, and tools. The research found that the quality of key attribute extraction using ChatGLM3 improved by 54.1% compared to dictionary-based methods, significantly reducing the workload of manual annotation. Additionally, the analysis revealed commonalities and differences in the demand features of data analysis, data engineering, and data operations roles across six dimensions: soft skills, tools, specialties required, educational background, experience, and salary.
Keywords
Large Language Model, Text Mining, ChatGLM3, Clustering Algorithm, Requirement Analysis
[1]. "Data Element Value Steadily Unleashed—Excerpt from the Data Element White Paper (2023)." (2024). Business Management, 2024(01), 46-52.
[2]. Zhang, L. J. (2023). "The unveiling of the National Data Bureau: How to activate a trillion-yuan market?" China Report, 2023(12), 58-59.
[3]. Renrui Human Resources & Deloitte China. (2023, March 17). "Industrial Digital Talent Research and Development Report (2023)." Retrieved from https://www.renruihr.com/web/news/detail?uid=20230317134128622U00000000003063
[4]. Gu, Y., & Lü, B. (2018). "Research on the demand structure of domestic information analysts—Based on the mining analysis of recruitment website information." Intelligence Exploration, 2018(07), 41-47.
[5]. Zhang, J. F. (2018). "Mining and application research on the demand characteristics of domestic website recruitment positions" [Doctoral dissertation, Anhui University of Finance and Economics].
[6]. Wei, T. T., Fang, H. Y., Song, S. L., et al. (2019). "Recruitment characteristic mining of data analysis positions under the background of big data." Modern Computer, 2019(25), 14-17+27.
[7]. Kang, P., Zang, J., & Cao, L. H. (2021). "Undergraduate teaching reform and practice under job demand matching—Taking the construction of big data analysis class course system as an example." Business Economy, 2021(04), 194-196. https://doi.org/10.19905/j.cnki.syjj1982.2021.04.069
[8]. Wang, Y. C. (2023). "Analysis of talent demand in data-related positions based on text mining" [Doctoral dissertation, Lanzhou University of Finance and Economics]. https://doi.org/10.27732/d.cnki.gnzsx.2022.000296
[9]. Zhao, J. L., Zhu, H., & Liu, X. (2019). "Research on the extraction of library knowledge group characteristics based on improved TFIDF." Systems Science and Mathematics, 2019, 39(09), 1450-1461.
[10]. Tian, Ziyang, "A Comparative Study of Document Representation Methods" (2019). Electronic Theses and Dissertations. 8183.
[11]. White, J., et al., A Prompt Pattern Catalog to Enhance Prompt Engineering with ChatGPT. ArXiv, 2023. abs/2302.11382.
[12]. Wei, W., Ding, X. X., & Guo, M. X., et al. (2024, February 21). "A review of text similarity calculation methods." Computer Engineering. https://doi.org/10.19678/j.issn.1000-3428.0068086
[13]. Guan, S. Z., Tang, Y. B., Cai, Z., et al. (2023). "Ultra-short-term forecasting of solar irradiance based on Kmeans++-Bi-LSTM." Journal of Solar Energy, 2023, 44(12), 170-174. https://doi.org/10.19912/j.0254-0096.tynxb.2022-1294
[14]. Yue, S., & Yong, Q. L. (2023). "An optimized K-means algorithm based on determining initial cluster centers." Digital Technology and Applications, 2023, 41(11), 140-142. https://doi.org/10.19695/j.cnki.cn12-1369.2023.11.44
[15]. Debao, D., Y. Ma and Z. Min, Analysis of big data job requirements based on K-means text clustering in China. PLoS ONE, 2021. 16.
[16]. Gardiner, A., et al., Skill Requirements in Big Data: A Content Analysis of Job Advertisements. Journal of Computer Information Systems, 2018. 58: p. 374 - 384.
[17]. Stojanović, D., et al., Employer Requirements for Graduate Competencies in Applied Artificial Intelligence. 2023 16th International Conference on Advanced Technologies, Systems and Services in Telecommunications (TELSIKS), 2023: p. 299-303.
Cite this article
Zhou,J.;Chen,Y.;Guo,Y.;Zhang,C. (2024). Data job requirement characteristics analysis based on large language model and K-means clustering. Applied and Computational Engineering,64,175-184.
Data availability
The datasets used and/or analyzed during the current study will be available from the authors upon reasonable request.
Disclaimer/Publisher's Note
The statements, opinions and data contained in all publications are solely those of the individual author(s) and contributor(s) and not of EWA Publishing and/or the editor(s). EWA Publishing and/or the editor(s) disclaim responsibility for any injury to people or property resulting from any ideas, methods, instructions or products referred to in the content.
About volume
Volume title: Proceedings of the 6th International Conference on Computing and Data Science
© 2024 by the author(s). Licensee EWA Publishing, Oxford, UK. This article is an open access article distributed under the terms and
conditions of the Creative Commons Attribution (CC BY) license. Authors who
publish this series agree to the following terms:
1. Authors retain copyright and grant the series right of first publication with the work simultaneously licensed under a Creative Commons
Attribution License that allows others to share the work with an acknowledgment of the work's authorship and initial publication in this
series.
2. Authors are able to enter into separate, additional contractual arrangements for the non-exclusive distribution of the series's published
version of the work (e.g., post it to an institutional repository or publish it in a book), with an acknowledgment of its initial
publication in this series.
3. Authors are permitted and encouraged to post their work online (e.g., in institutional repositories or on their website) prior to and
during the submission process, as it can lead to productive exchanges, as well as earlier and greater citation of published work (See
Open access policy for details).