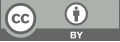
Prediction of heart attack based on ensemble learning
- 1 Shandong University
* Author to whom correspondence should be addressed.
Abstract
Heart disease has always been a high proportion of diseases worldwide, and it is also one of the leading causes of death worldwide. The prevention and detection of heart disease is still a key issue. To enhance patient outcomes in the real world and add to the expanding body of knowledge in heart disease research, this work aims to develop an integrated learning model to predict heart disease using machine learning techniques. The main research object of this paper is the heart disease data set. This paper's primary approach is to use 10 distinct machine-learning algorithms to construct a model for predicting heart attacks. And then, score and compare the performance of the models with some fundamental metrics. According to the result of the performance, the best three are selected, and a better-ensembled model is built through the ensemble learning of the voting method with Multilayer Perceptron Classifier (MLPC), the Support Vector Machine (SVM), and the Naive Bayes. This may be likely to help with real-life diagnoses in the future.
Keywords
Heart-attack prediction, Machine learning, Classification, Ensemble learning
[1]. Palaniappan, Sellappan, and Rafiah Awang. 2008. “Intelligent Heart Disease Prediction System Using Data Mining Techniques.” In 2008 IEEE/ACS International Conference on Computer Systems and Applications. doi:10.1109/aiccsa.2008.4493524.
[2]. Rajkumar, Asha, and G.Sophia Reena. 2010. “Diagnosis of Heaer Disease Using Datamining Algorithm.” Global Journal of Computer Science and Technology, Global Journal of Computer Science and Technology, September.
[3]. Soni, Jyoti, Ujma Ansari, Dipesh Sharma, and Sunita Soni. 2011. “Predictive Data Mining for Medical Diagnosis: An Overview of Heart Disease Prediction.” International Journal of Computer Applications, April, 43–48. doi:10.5120/2237-2860.
[4]. S. Dangare, Chaitrali, and Sulbha S. Apte. 2012. “Improved Study of Heart Disease Prediction System Using Data Mining Classification Techniques.” International Journal of Computer Applications, July, 44–48. doi:10.5120/7228-0076.
[5]. García, Salvador, Sergio Ramírez-Gallego, Julián Luengo, José Manuel Benítez, and Francisco Herrera. 2016. “Big Data Preprocessing: Methods and Prospects.” Big Data Analytics, December. doi:10.1186/s41044-016-0014-0.
[6]. Wang, Hai, and Shouhong Wang. 2010. “Mining Incomplete Survey Data through Classification.” Knowledge and Information Systems, August, 221–33. doi:10.1007/s10115-009-0245-8.
[7]. Rodriguez, J.D., A. Perez, and J.A. Lozano. 2010. “Sensitivity Analysis of K-Fold Cross Validation in Prediction Error Estimation.” IEEE Transactions on Pattern Analysis and Machine Intelligence, March, 569–75. doi:10.1109/tpami.2009.187.
[8]. A. Patel and D. S. Chouhan, "SVM kernel functions for classification," 2013 International Conference on Advances in Technology and Engineering (ICATE), Mumbai, India, 2013, pp. 1-9, doi: 10.1109/ICAdTE.2013.6524743.
[9]. Lobo, Jorge M., Alberto Jiménez-Valverde, and Raimundo Real. 2008. “AUC: A Misleading Measure of the Performance of Predictive Distribution Models.” Global Ecology and Biogeography, March, 145–51. doi:10.1111/j.1466-8238.2007.00358.x.
[10]. Mountrakis, Giorgos, Jungho Im, and Caesar Ogole. 2011. “Support Vector Machines in Remote Sensing: A Review.” ISPRS Journal of Photogrammetry and Remote Sensing, May, 247–59. doi:10.1016/j.isprsjprs.2010.11.001.
[11]. Friedman, Nir, Dan Geiger, and Moises Goldszmidt. 1997. “Bayesian Network Classifiers.” Machine Learning, January, 131–63. doi:10.1023/a:1007465528199.
[12]. Leiva, RafaelGarcia, AntonioFernández Anta, Vincenzo Mancuso, and Paolo Casari. 2019. “A Novel Hyperparameter-Free Approach to Decision Tree Construction That Avoids Overfitting by Design.” arXiv: Learning,arXiv: Learning, June.
[13]. Rokach, Lior, and Oded Maimon. 2007. “Data Mining with Decision Trees: Theory and Applications.” Series in Machine Perception and Artificial Intelligence, Series in Machine Perception and Artificial Intelligence, December.
Cite this article
Zhang,X. (2024). Prediction of heart attack based on ensemble learning. Applied and Computational Engineering,73,302-314.
Data availability
The datasets used and/or analyzed during the current study will be available from the authors upon reasonable request.
Disclaimer/Publisher's Note
The statements, opinions and data contained in all publications are solely those of the individual author(s) and contributor(s) and not of EWA Publishing and/or the editor(s). EWA Publishing and/or the editor(s) disclaim responsibility for any injury to people or property resulting from any ideas, methods, instructions or products referred to in the content.
About volume
Volume title: Proceedings of the 2nd International Conference on Software Engineering and Machine Learning
© 2024 by the author(s). Licensee EWA Publishing, Oxford, UK. This article is an open access article distributed under the terms and
conditions of the Creative Commons Attribution (CC BY) license. Authors who
publish this series agree to the following terms:
1. Authors retain copyright and grant the series right of first publication with the work simultaneously licensed under a Creative Commons
Attribution License that allows others to share the work with an acknowledgment of the work's authorship and initial publication in this
series.
2. Authors are able to enter into separate, additional contractual arrangements for the non-exclusive distribution of the series's published
version of the work (e.g., post it to an institutional repository or publish it in a book), with an acknowledgment of its initial
publication in this series.
3. Authors are permitted and encouraged to post their work online (e.g., in institutional repositories or on their website) prior to and
during the submission process, as it can lead to productive exchanges, as well as earlier and greater citation of published work (See
Open access policy for details).