Volume 153
Published on May 2025Volume title: Proceedings of the 3rd International Conference on Mechatronics and Smart Systems

Driven by advanced capabilities of fifth-generation (5G) mobile networks, vehicle-to-everything (V2X) communication is transitioning from theoretical concept to practical implementation. However, the inherent characteristics of vehicular environments—high mobility, dynamic topology and dense traffic scenarios—pose significant challenges in meeting the stringent and diverse quality-of-service (QoS) requirements for V2X applications. This article explores the application of network slicing technology, supported by Network Function Virtualization (NFV) and Software Defined Networking (SDN). The requirements for network slicing in the context of the Internet of Vehicles are analyzed. In response to these needs, the article further studies the specific applications of network slicing technology in these scenarios. Finally, the article discusses the challenges faced by network slicing in the Internet of Vehicles and looks forward to future prospects such as intelligent management, cross industry cooperation, and standardization. The findings reveal that effective implementation of network slicing can significantly enhance the performance and reliability of V2X communication, paving the way for safer and more efficient vehicular networks.
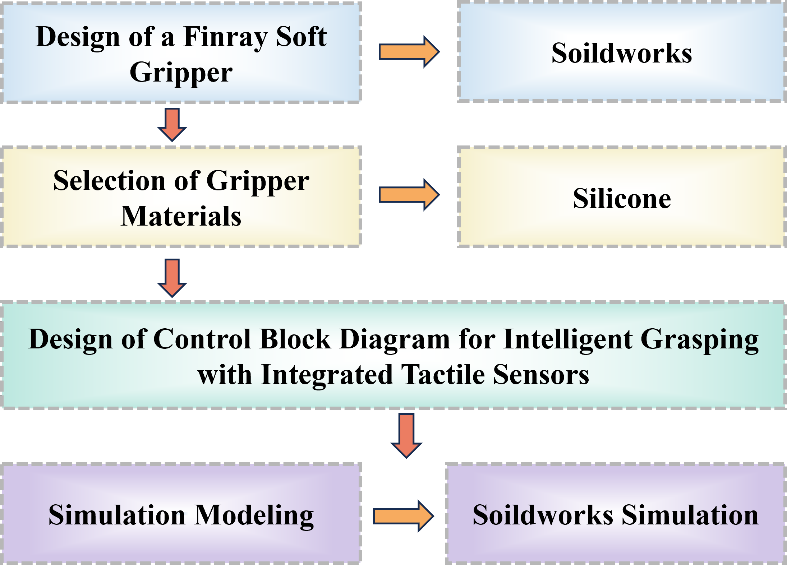
As a key process in agricultural production, the demand for high-efficiency and high-quality tomato harvesting is continuously rising with the rapid advancement of smart agriculture. However, conventional rigid manipulators often fail to meet the needs of intelligent harvesting due to the limitations of adaptability, dexterity, and safety. To address these challenges, this study proposes a silicone-based fin-ray soft gripper inspired by bio-mimetic fin structures. The gripper is designed to adaptively deform when grasping tomatoes, thereby enhancing grip stability while minimizing mechanical damage. The gripper’s mechanical performance is evaluated through SolidWorks modeling and built-in simulation analysis. Static analysis result shows uniform stress distribution, free-end displacement for adaptability, and controlled strain along ribs, confirming its flexibility and reliability under load conditions. Additionally, a closed-loop control system integrated with tactile sensors is developed to enable adaptive force regulation, further improving the intelligence of the harvesting process. The stress, displacement, and strain analyses demonstrate that under a 30N load, the designed gripper exhibits uniform stress distribution, excellent flexibility, and favorable mechanical properties. Compared to conventional grippers, this design offers significant improvements in notable flexibility, safety, and operational efficiency. This research provides an innovative end-effector solution for tomato-harvesting robots and serves as a valuable technical reference for the automated harvesting of other crops. It further demonstrates the significant application potential and scalability of agricultural harvesting grippers.
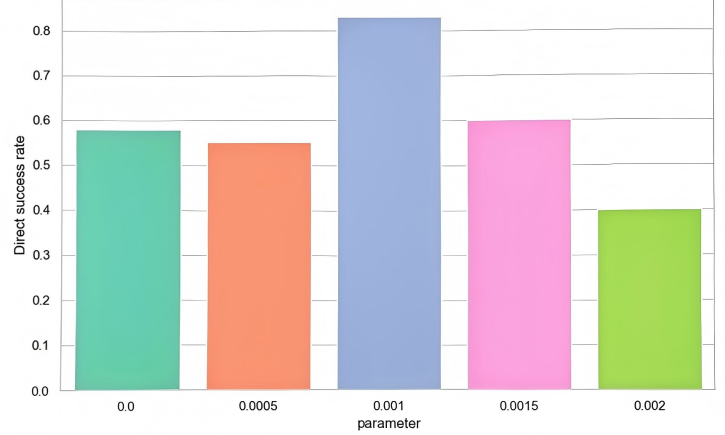
With autonomous driving technology playing an increasingly important role in intelligent transportation systems, how to improve ride comfort while ensuring safety has become an urgent challenge. This paper proposes a multi-objective optimization approach for autonomous driving based on reinforcement learning. By designing a multi-objective reward function that integrates rewards based on position, speed, direction, and acceleration, the method aims to balance driving efficiency and ride comfort. The Proximal Policy Optimization (PPO) algorithm is employed for training on the high-fidelity simulation platform MetaDrive, and experiments in multiple scenarios verify the effectiveness of the proposed approach. The experimental results show that as the comfort penalty coefficient in the reward function changes, the success rates for left turns, straight driving, and right turns exhibit a non-linear trend of first increasing then decreasing, with the best performance achieved when the parameter value is 0.001. This fully demonstrates the critical impact of parameter selection on the performance of autonomous driving strategies. It provides an optimization solution for reinforcement learning-based autonomous driving decision-making that balances safety and ride comfort, and offers a reference for subsequent related research.
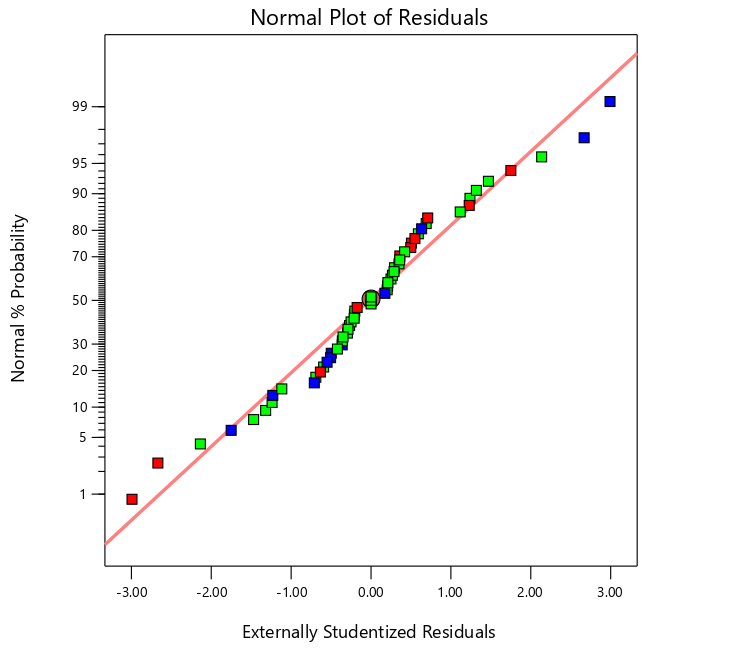
Excavators, as specialized equipment for energy extraction and construction in engineering machinery, play an important role in the quality and effectiveness of construction. With the gradual introduction of the concept of green environmental protection into society, energy conservation and material reduction have become one of the main development directions of excavators. This article takes a small excavator as an example to study the optimization design of its boom from the perspective of mechanical structure optimization design. SolidWorks is used to create a three-dimensional model of the boom in the excavator's working device. Ansys Workbench is used to perform finite element static analysis on the boom, obtaining the stress situation and hazardous conditions under various working conditions. The optimized position is determined through the topology optimization module. Design a response surface optimization simulation test group using Design Expert, and generate an optimization regression equation for the boom based on the results. Under the conditions of satisfying stiffness and strength, the optimal solution for boom optimization was obtained through this equation. A comprehensive and feasible method and approach are proposed for the optimization design of excavator boom by modeling the optimal solution and ultimately analyzing and verifying its correctness.