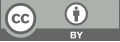
Deep learning in autonomous driving: Advantages, limitations, and innovative solutions
- 1 Minjiang University
* Author to whom correspondence should be addressed.
Abstract
With the rapid development of autonomous driving technology, deep learning has become a core driver for innovation in testing autonomous driving scenarios. This review paper delves into the critical role of deep learning in autonomous driving technology. The paper will describe how deep learning is at the center of driving innovation. The paper thoroughly explores the application of deep learning in obstacle detection, scene classification and understanding, and image segmentation, emphasizing the significant benefits in perception and decision-making while pointing out the challenges and innovative solutions adopted. The innovative solutions section proposes multimodal fusion and joint learning, new methods for 3D semantic segmentation, etc., aiming to improve image segmentation's accuracy and generalization ability. Overall, deep learning has great potential in automated driving technology, and by innovating and solving challenges, it will advance the system and provide reliable, intelligent, and efficient solutions for future transportation systems.
Keywords
Deep Learning, Autonomous Driving Technology, Image Segmentation, Innovative Solutions
[1]. A. Kumar and P. Saha, "A review of deep learning models for traffic flow prediction in Autonomous Vehicles," 2020 2nd International Conference on Advances in Computing, Communication Control and Networking (ICACCCN), Greater Noida, India, 2020, pp. 303-308, doi: 10.1109/ICACCCN51052.2020.9362972.
[2]. A. Khanum, C. -Y. Lee and C. -S. Yang, "Involvement of Deep Learning for Vision Sensor-Based Autonomous Driving Control: A Review," in IEEE Sensors Journal, vol. 23, no. 14, pp. 15321-15341, 15 July 15, 2023, doi: 10.1109/JSEN.2023.3280959.
[3]. E. Jebessa, K. Olana, K. Getachew, S. Isteefanos and T. K. Mohd, "Analysis of Reinforcement Learning in Autonomous Vehicles," 2022 IEEE 12th Annual Computing and Communication Workshop and Conference (CCWC), Las Vegas, NV, USA, 2022, pp. 0087-0091, doi: 10.1109/CCWC54503.2022.9720883.
[4]. G. Tong, J. Cai, L. Huang, B. Shang, J. Liu and M. Chen, "SSD Based Target Detection Algorithm for the Autonomous Driving Vision System," 2021 IEEE 2nd International Conference on Information Technology, Big Data and Artificial Intelligence (ICIBA), Chongqing, China, 2021, pp. 213-217, doi: 10.1109/ICIBA52610.2021.9688170.
[5]. N. S. Manikandan, G. Kaliyaperumal and Y. Wang, "Ad Hoc-Obstacle Avoidance-Based Navigation System Using Deep Reinforcement Learning for Self-Driving Vehicles," in IEEE Access, vol. 11, pp. 92285-92297, 2023, doi: 10.1109/ACCESS.2023.3297661.
[6]. A. Agrawal, "Autonomous Self Driving System Using Deep Learning Appraoches and Impact," 2023 International Conference on Circuit Power and Computing Technologies (ICCPCT), Kollam, India, 2023, pp. 961-970, doi: 10.1109/ICCPCT58313.2023.10245567.
[7]. N. S. Manikandan, G. Kaliyaperumal and Y. Wang, "Ad Hoc-Obstacle Avoidance-Based Navigation System Using Deep Reinforcement Learning for Self-Driving Vehicles," in IEEE Access, vol. 11, pp. 92285-92297, 2023, doi: 10.1109/ACCESS.2023.3297661.
[8]. Jingyuan Zhao et al., “Autonomous driving system: A comprehensive survey,” in Expert Systems with Applications Volume 242, 15 May 2024, 122836
[9]. Badr Ben Elallid et al., “A Comprehensive Survey on the Application of Deep and Reinforcement Learning Approaches in Autonomous Driving,” in Journal of King Saud University - Computer and Information Sciences, Volume 34, Issue 9, October 2022, Pages 7366-7390.
[10]. N. N. Ali, N. A. Kako and A. S. Abdi, "Review on Image Segmentation Methods Using Deep Learning," 2022 4th International Conference on Advanced Science and Engineering (ICOASE), Zakho, Iraq, 2022, pp. 7-12, doi: 10.1109/ICOASE56293.2022.10075607.
[11]. Y. Cui et al., "Deep Learning for Image and Point Cloud Fusion in Autonomous Driving: A Review," in IEEE Transactions on Intelligent Transportation Systems, vol. 23, no. 2, pp. 722-739, Feb. 2022, doi: 10.1109/TITS.2020.3023541.
[12]. A. Srivastav and S. Mandal, "Radars for Autonomous Driving: A Review of Deep Learning Methods and Challenges," in IEEE Access, vol. 11, pp. 97147-97168, 2023, doi: 10.1109/ACCESS.2023.3312382.
[13]. Y. Qiao, J. Yin, W. Wang, F. Duarte, J. Yang, and C. Ratti, "Survey of Deep Learning for Autonomous Surface Vehicles in Marine Environments," in IEEE Transactions on Intelligent Transportation Systems, vol. 24, no. 4, pp. 3678-3701, April 2023, doi: 10.1109/TITS.2023.3235911.
[14]. A. Juyal, S. Sharma and P. Matta, "Traffic Sign Detection using Deep Learning Techniques in Autonomous Vehicles," 2021 International Conference on Innovative Computing, Intelligent Communication and Smart Electrical Systems (ICSES), Chennai, India, 2021, pp. 1-7, doi: 10.1109/ICSES52305.2021.9633959.
[15]. Li, Y., Ma, L., Zhong, Z., Liu, F., Chapman, M. A., Cao, D., & Li, J. (2020). Deep learning for lidar point clouds in autonomous driving: A review. IEEE Transactions on Neural Networks and Learning Systems, 32(8), 3412-3432, doi: 10.1109/TNNLS.2020.3015992.
Cite this article
You,S. (2024). Deep learning in autonomous driving: Advantages, limitations, and innovative solutions. Applied and Computational Engineering,75,147-153.
Data availability
The datasets used and/or analyzed during the current study will be available from the authors upon reasonable request.
Disclaimer/Publisher's Note
The statements, opinions and data contained in all publications are solely those of the individual author(s) and contributor(s) and not of EWA Publishing and/or the editor(s). EWA Publishing and/or the editor(s) disclaim responsibility for any injury to people or property resulting from any ideas, methods, instructions or products referred to in the content.
About volume
Volume title: Proceedings of the 2nd International Conference on Software Engineering and Machine Learning
© 2024 by the author(s). Licensee EWA Publishing, Oxford, UK. This article is an open access article distributed under the terms and
conditions of the Creative Commons Attribution (CC BY) license. Authors who
publish this series agree to the following terms:
1. Authors retain copyright and grant the series right of first publication with the work simultaneously licensed under a Creative Commons
Attribution License that allows others to share the work with an acknowledgment of the work's authorship and initial publication in this
series.
2. Authors are able to enter into separate, additional contractual arrangements for the non-exclusive distribution of the series's published
version of the work (e.g., post it to an institutional repository or publish it in a book), with an acknowledgment of its initial
publication in this series.
3. Authors are permitted and encouraged to post their work online (e.g., in institutional repositories or on their website) prior to and
during the submission process, as it can lead to productive exchanges, as well as earlier and greater citation of published work (See
Open access policy for details).