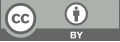
Machine learning insights into digital payment behaviors and fraud prediction
- 1 Carnegie Mellon University
* Author to whom correspondence should be addressed.
Abstract
With the continuous advancement of digital transformation, digital payments are playing an increasingly important role in the financial industry. This study aims to utilize machine learning models to predict and analyze digital payment behavior. Initially, the background and significance of digital payments in the financial sector are introduced. Subsequently, the current status and trends of traditional digital payment distribution are reviewed, alongside related work on digital payment behavior prediction. Methodologically, principles and applications of machine learning models such as logistic regression, decision trees, and random forests are elaborated, along with experimental design and data preprocessing methods. The experimental results and discussion section illustrates the performance of each model in digital payment prediction and explores their impact on credit decisions. This exploration equips financial institutions with more effective user behavior analysis and risk management tools, thereby fostering future development and application of digital payment technologies.
Keywords
Digital payments, machine learning models, forecasting, financial services
[1]. Patil, Pushp P., Yogesh K. Dwivedi, and Nripendra P. Rana. "Digital payments adoption: an analysis of literature." Digital Nations–Smart Cities, Innovation, and Sustainability: 16th IFIP WG 6.11 Conference on E-Business, E-Services, and E-Society, I3E 2017, Delhi, India, November 21–23, 2017, Proceedings 16. Springer International Publishing, 2017.
[2]. Chaudhary, K., Yadav, J., & Mallick, B. (2012). A review of fraud detection techniques: Credit card. International Journal of Computer Applications, 45(1), 39-44.
[3]. Awoyemi, J. O., Adetunmbi, A. O., & Oluwadare, S. A. (2017, October). Credit card fraud detection using machine learning techniques: A comparative analysis. In 2017 international conference on computing networking and informatics (ICCNI)(pp. 1-9). IEEE.
[4]. Carbo-Valverde, Santiago, Pedro Cuadros-Solas, and Francisco Rodríguez-Fernández. "A machine learning approach to the digitalization of bank customers: Evidence from random and causal forests." Plos one 15.10 (2020): e0240362.
[5]. Alexander, C., & Dakos, M. (2020). A critical investigation of cryptocurrency data and analysis. Quantitative Finance, 20(2), 173-188.
[6]. Ma, Haowei. "Research on promotion of lower limb movement function recovery after stroke by using lower limb rehabilitation robot in combination with constant velocity muscle strength training." 2021 7th international symposium on mechatronics and industrial informatics (ISMII). IEEE, 2021.
[7]. Sun, Y., Cui, Y., Hu, J., & Jia, W. (2018). Relation classification using coarse and fine-grained networks with SDP supervised key words selection. In Knowledge Science, Engineering and Management: 11th International Conference, KSEM 2018, Changchun, China, August 17–19, 2018, Proceedings, Part I 11 (pp. 514-522). Springer International Publishing.
[8]. Huo, Mingda, et al. "JPX Tokyo Stock Exchange Prediction with LightGBM." Proceedings of the 2nd International Conference on Bigdata Blockchain and Economy Management, ICBBEM 2023, May 19–21, 2023, Hangzhou, China. 2023.
[9]. Taylor, Sean J., and Benjamin Letham. "Forecasting at scale." The American Statistician 72.1 (2018): 37-45.
[10]. Srivastava, A., Kundu, A., Sural, S., & Majumdar, A. (2008). Credit card fraud detection using hidden Markov model. IEEE Transactions on dependable and secure computing, 5(1), 37-48.
[11]. Patidar, R., & Sharma, L. (2011). Credit card fraud detection using neural network. International Journal of Soft Computing and Engineering (IJSCE), 1(32-38).
Cite this article
Zhang,X. (2024). Machine learning insights into digital payment behaviors and fraud prediction. Applied and Computational Engineering,77,203-209.
Data availability
The datasets used and/or analyzed during the current study will be available from the authors upon reasonable request.
Disclaimer/Publisher's Note
The statements, opinions and data contained in all publications are solely those of the individual author(s) and contributor(s) and not of EWA Publishing and/or the editor(s). EWA Publishing and/or the editor(s) disclaim responsibility for any injury to people or property resulting from any ideas, methods, instructions or products referred to in the content.
About volume
Volume title: Proceedings of the 2nd International Conference on Software Engineering and Machine Learning
© 2024 by the author(s). Licensee EWA Publishing, Oxford, UK. This article is an open access article distributed under the terms and
conditions of the Creative Commons Attribution (CC BY) license. Authors who
publish this series agree to the following terms:
1. Authors retain copyright and grant the series right of first publication with the work simultaneously licensed under a Creative Commons
Attribution License that allows others to share the work with an acknowledgment of the work's authorship and initial publication in this
series.
2. Authors are able to enter into separate, additional contractual arrangements for the non-exclusive distribution of the series's published
version of the work (e.g., post it to an institutional repository or publish it in a book), with an acknowledgment of its initial
publication in this series.
3. Authors are permitted and encouraged to post their work online (e.g., in institutional repositories or on their website) prior to and
during the submission process, as it can lead to productive exchanges, as well as earlier and greater citation of published work (See
Open access policy for details).