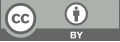
Research on personal credit scoring model based on deep learning
- 1 Financial Engineering in UCLA
* Author to whom correspondence should be addressed.
Abstract
The increasing integration of internet technology and the financial industry has led to the gradual replacement of traditional credit evaluation models with those based on deep learning, which have demonstrated excellent accuracy. This has become a prominent area of research. Nevertheless, the credit scoring model based on a deep neural network encounters significant challenges in terms of its applicability in the field of credit scoring, largely due to the opaque nature of its learning and decision-making processes. The application of deep learning to personal credit scoring has been shown to enhance the accuracy of the resulting scores by leveraging large amounts of data. The model employs a deep neural network (DNN) architecture that integrates multiple input features, including the user's transaction history, social behaviour and other relevant data. The model is trained using supervised learning, with a large amount of labelled data used to optimise its prediction performance. Experimental results demonstrate that the deep learning-based model exhibits a notable improvement in accuracy and robustness compared to traditional credit scoring models.
Keywords
Credit Scoring Model, Deep Neural Network, Personal Features, Prediction Performance
[1]. Qin, Chao, et al. "XGBoost optimized by adaptive particle swarm optimization for credit scoring." Mathematical Problems in Engineering 2021.1 (2021): 6655510.
[2]. Liu, Wanan, Hong Fan, and Meng Xia. "Credit scoring based on tree-enhanced gradient boosting decision trees." Expert Systems with Applications 189 (2022): 116034.
[3]. Moscato, Vincenzo, Antonio Picariello, and Giancarlo Sperlí. "A benchmark of machine learning approaches for credit score prediction." Expert Systems with Applications 165 (2021): 113986.
[4]. Zhang, Wenyu, et al. "A novel multi-stage ensemble model with enhanced outlier adaptation for credit scoring." Expert Systems with Applications 165 (2021): 113872.
[5]. Ala'raj, Maher, Maysam F. Abbod, and Munir Majdalawieh. "Modelling customers credit card behaviour using bidirectional LSTM neural networks." Journal of Big Data 8.1 (2021): 69.
[6]. Gao, Lu, and Jian Xiao. "Big data credit report in credit risk management of consumer finance." Wireless Communications and Mobile Computing 2021.1 (2021): 4811086.
[7]. Huang, Cheng-Lung, Mu-Chen Chen, and Chieh-Jen Wang. "Credit scoring with a data mining approach based on support vector machines." Expert systems with applications 33.4 (2007): 847-856.
[8]. Chern, Ching-Chin, et al. "A decision tree classifier for credit assessment problems in big data environments." Information Systems and e-Business Management 19 (2021): 363-386.
[9]. Itoo, Fayaz, Meenakshi, and Satwinder Singh. "Comparison and analysis of logistic regression, Naïve Bayes and KNN machine learning algorithms for credit card fraud detection." International Journal of Information Technology 13.4 (2021): 1503-1511.
[10]. Zhang, Wenyu, et al. "A novel multi-stage ensemble model with enhanced outlier adaptation for credit scoring." Expert Systems with Applications 165 (2021): 113872.
[11]. Neagoe, Victor-Emil, Adrian-Dumitru Ciotec, and George-Sorin Cucu. "Deep convolutional neural networks versus multilayer perceptron for financial prediction." 2018 International Conference on Communications (COMM). IEEE, 2018.
[12]. Kvamme, Håvard, et al. "Predicting mortgage default using convolutional neural networks." Expert Systems with Applications 102 (2018): 207-217.
[13]. Dastile, Xolani, and Turgay Celik. "Making deep learning-based predictions for credit scoring explainable." IEEE Access 9 (2021): 50426-50440.
Cite this article
Yan,T. (2024). Research on personal credit scoring model based on deep learning. Applied and Computational Engineering,87,203-208.
Data availability
The datasets used and/or analyzed during the current study will be available from the authors upon reasonable request.
Disclaimer/Publisher's Note
The statements, opinions and data contained in all publications are solely those of the individual author(s) and contributor(s) and not of EWA Publishing and/or the editor(s). EWA Publishing and/or the editor(s) disclaim responsibility for any injury to people or property resulting from any ideas, methods, instructions or products referred to in the content.
About volume
Volume title: Proceedings of the 6th International Conference on Computing and Data Science
© 2024 by the author(s). Licensee EWA Publishing, Oxford, UK. This article is an open access article distributed under the terms and
conditions of the Creative Commons Attribution (CC BY) license. Authors who
publish this series agree to the following terms:
1. Authors retain copyright and grant the series right of first publication with the work simultaneously licensed under a Creative Commons
Attribution License that allows others to share the work with an acknowledgment of the work's authorship and initial publication in this
series.
2. Authors are able to enter into separate, additional contractual arrangements for the non-exclusive distribution of the series's published
version of the work (e.g., post it to an institutional repository or publish it in a book), with an acknowledgment of its initial
publication in this series.
3. Authors are permitted and encouraged to post their work online (e.g., in institutional repositories or on their website) prior to and
during the submission process, as it can lead to productive exchanges, as well as earlier and greater citation of published work (See
Open access policy for details).