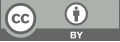
Apply multiple machine learning models to diabetes prediction
- 1 Aberdeen Institute of Data Science and Artificial Intelligence, South China Normal University, Foshan, China
* Author to whom correspondence should be addressed.
Abstract
Diabetes mellitus, a pervasive and chronic metabolic disorder, imposes a substantial burden on global health systems due to its requirement for lifelong management and the myriad of complications associated with inadequate control. The ability to accurately forecast the onset of this disease is paramount, as it enables preemptive interventions and tailored treatment strategies that can significantly mitigate its impact. This paper investigates the application of machine learning techniques and deep learning models in diabetes prediction. This paper makes use of the Pima Indian Diabetes Dataset (PIDD) from Kaggle, which has 768 data entries and eight characteristics like blood pressure, blood sugar, and body mass index (BMI). Various algorithms, including Support Vector Machine (SVM), Decision Trees(DT), Random Forest(RF) , and Fully Connected Neural Network (FCNN), are implemented and compared. Identifying the strengths and limitations of each model, the results emphasize the potential of advanced computational models in improving the accuracy and clinical usefulness of diabetes prediction. The best-performing model is the FCNN model, with a test accuracy of 78.67% and an AUC value of 83.36%.
Keywords
Diabetes prediction, Fully Connected Neural Network, Random Forest, Decision Tree, Support Vector Machine
[1]. Alazwari A, Johnstone A, Tafakori L, et al., Alshamrani M.A., 2023. Predicting the development of T1D and identifying its Key Performance Indicators in children. PLoS ONE, 18(3): e0282426.
[2]. Mangal A, Jain V, 2022. Performance analysis of machine learning models for prediction of diabetes. Conf Inf Sci Comput Technol, Dehradun, India: 1-4.
[3]. Pan L, 2024. Construction and validation of a risk prediction model for diabetic nephropathy complicated by hyperuricemia. Med Theory Pract, (09): 1559-1561.
[4]. Pal M, Parija S, Panda G, 2021. Improved Prediction of Diabetes Mellitus using Machine Learning Based Approach. Int Conf on Robot Technol, Chandipur, Balasore, India: 1-6.
[5]. Jiang L, Xia Z, Zhu R, et al., 2023. Diabetes risk prediction model based on community follow-up data using machine learning. Phys Med Rehabil, Volume 35: 102358.
[6]. Bhat S, Banu M, Ansari G, et al., 2023. A risk assessment and prediction framework for diabetes mellitus using machine learning algorithms. Healthcare, Volume 4: 100273.
[7]. Reza M, Hafsha U, Amin R, et al., 2023. Improving SVM performance for type II diabetes prediction with an improved non-linear kernel: Insights from the PIMA dataset. Comput Methods Programs Biomed Update, Volume 4: 100118.
[8]. Mahajan S, Sarangi P, Sahoo A, Rohra M, 2023. Diabetes Mellitus Prediction using Supervised Machine Learning Techniques. Int Conf Adv Comput Comput Technol, Gharuan, India: 587-592.
[9]. Paul B, Karn B, 2021. Diabetes Mellitus Prediction using Hybrid Artificial Neural Network. Int Bus Soc Sci Conf, Gwalior, India: 1-5.
[10]. Hu N, Gao J, 2023. Research on Diabetes Prediction Model Based on Machine Learning Algorithms. Conf Int Prod Autom Eng, Ottawa, ON, Canada: 200-203.
Cite this article
Qin,S. (2024). Apply multiple machine learning models to diabetes prediction. Applied and Computational Engineering,86,221-230.
Data availability
The datasets used and/or analyzed during the current study will be available from the authors upon reasonable request.
Disclaimer/Publisher's Note
The statements, opinions and data contained in all publications are solely those of the individual author(s) and contributor(s) and not of EWA Publishing and/or the editor(s). EWA Publishing and/or the editor(s) disclaim responsibility for any injury to people or property resulting from any ideas, methods, instructions or products referred to in the content.
About volume
Volume title: Proceedings of the 6th International Conference on Computing and Data Science
© 2024 by the author(s). Licensee EWA Publishing, Oxford, UK. This article is an open access article distributed under the terms and
conditions of the Creative Commons Attribution (CC BY) license. Authors who
publish this series agree to the following terms:
1. Authors retain copyright and grant the series right of first publication with the work simultaneously licensed under a Creative Commons
Attribution License that allows others to share the work with an acknowledgment of the work's authorship and initial publication in this
series.
2. Authors are able to enter into separate, additional contractual arrangements for the non-exclusive distribution of the series's published
version of the work (e.g., post it to an institutional repository or publish it in a book), with an acknowledgment of its initial
publication in this series.
3. Authors are permitted and encouraged to post their work online (e.g., in institutional repositories or on their website) prior to and
during the submission process, as it can lead to productive exchanges, as well as earlier and greater citation of published work (See
Open access policy for details).