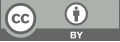
A comprehensive review on the application of CVSS 4.0 and deep learning in vulnerability
- 1 Beijing University of Posts and Telecommunications
* Author to whom correspondence should be addressed.
Abstract
This paper reviews the evolution of the Common Vulnerability Scoring System (CVSS), focusing on the enhancements and applications of CVSS 4.0. It also explores the potential integration of deep Learning techniques in vulnerability assessment. Key findings include identifying critical improvements in CVSS 4.0 that address previous limitations and enhance accuracy and granularity in vulnerability scoring. Additionally, the paper demonstrates how deep Learning models can predict vulnerability scores and trends, thereby improving the speed and precision of assessments. By combining CVSS 4.0 with deep Learning technologies, this paper proposes a more comprehensive and efficient approach to vulnerability assessment, which could significantly enhance proactive security measures and risk management strategies.
Keywords
CVSS 4.0, Deep Learning, Vulnerability Assessment, Risk Management
[1]. Scarfone K, Mell P. An analysis of CVSS version 2 vulnerability scoring[C]//2009 3rd International Symposium on Empirical Software Engineering and Measurement. IEEE, 2009: 516-525.
[2]. Gallon L, Bascou J J. Using CVSS in attack graphs[C]//2011 Sixth International Conference on Availability, Reliability, and Security. IEEE, 2011: 59-66.
[3]. Ruohonen J. A look at the time delays in CVSS vulnerability scoring[J]. Applied Computing and Informatics, 2019, 15(2): 129-135.
[4]. Costa J C, Roxo T, Sequeiros J B F, et al. Predicting CVSS metric via description interpretation[J]. IEEE Access, 2022, 10: 59125-59134.
[5]. Gallon L. On the impact of environmental metrics on CVSS scores[C]//2010 IEEE Second international conference on social computing. IEEE, 2010: 987-992.
Cite this article
Xie,H. (2024). A comprehensive review on the application of CVSS 4.0 and deep learning in vulnerability. Applied and Computational Engineering,87,234-240.
Data availability
The datasets used and/or analyzed during the current study will be available from the authors upon reasonable request.
Disclaimer/Publisher's Note
The statements, opinions and data contained in all publications are solely those of the individual author(s) and contributor(s) and not of EWA Publishing and/or the editor(s). EWA Publishing and/or the editor(s) disclaim responsibility for any injury to people or property resulting from any ideas, methods, instructions or products referred to in the content.
About volume
Volume title: Proceedings of the 6th International Conference on Computing and Data Science
© 2024 by the author(s). Licensee EWA Publishing, Oxford, UK. This article is an open access article distributed under the terms and
conditions of the Creative Commons Attribution (CC BY) license. Authors who
publish this series agree to the following terms:
1. Authors retain copyright and grant the series right of first publication with the work simultaneously licensed under a Creative Commons
Attribution License that allows others to share the work with an acknowledgment of the work's authorship and initial publication in this
series.
2. Authors are able to enter into separate, additional contractual arrangements for the non-exclusive distribution of the series's published
version of the work (e.g., post it to an institutional repository or publish it in a book), with an acknowledgment of its initial
publication in this series.
3. Authors are permitted and encouraged to post their work online (e.g., in institutional repositories or on their website) prior to and
during the submission process, as it can lead to productive exchanges, as well as earlier and greater citation of published work (See
Open access policy for details).