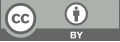
Research on movie recommendation algorithms based on machine learning
- 1 Taiyuan University of Technology, School of Software, Software Engineering, Taiyuan, China, 030000
* Author to whom correspondence should be addressed.
Abstract
In recent years, the number of movies released worldwide has grown exponentially. Due to the large number of movies, it is difficult for users to find movies that match their preferences. Therefore, with the development of Internet technology, it has become an important research direction how to filter out the movies that users are interested in from the massive movie data. This paper mainly focuses on the film recommendation algorithms based on machine learning, including the traditional collaborative filtering algorithm, rating-based sorting recommendation algorithm and content-based recommendation algorithm. By conducting a detailed analysis of the principles, advantages and disadvantages, as well as application scenarios of these recommendation algorithms, this paper aims to identify methods that best fit current movie recommendation systems. The objective is to improve the real-time and personalized recommendation, while providing users with better film recommendation services.
Keywords
Movie recommendations, machine learning, collaborative filtering, recommendation algorithm
[1]. Wang Z. A content-based collaborative filtering algorithm for movies and TVS recommendation. Proceedings of the 5th International Conference on Computing and Data Science. 2023:10. DOI:10.26914/c.cnkihy.2023.108612.
[2]. Zhang Qi, Yu Shuangyuan, Yin Hong-feng and Xu Baomin. Neural Collaborative Filtering for Social Recommendation Algorithm. Computer Science 2023(02): 115-122.
[3]. Zhao J. Research and improvement of movie recommendation based on a collaborative filtering algorithm. Proceedings of the 5th International Conference on Computing and Data Science. 2023:13. DOI:10.26914/c.cnkihy.2023.108744.
[4]. Myers L, Sirois M J. Spearman correlation coefficients, differences between[J]. Wiley StatsRef: Statistics Reference Online, 2014.
[5]. Benesty J, Chen J, Huang Y. On the importance of the Pearson correlation coefficient in noise reduction[J]. IEEE Transactions on Audio, Speech, and Language Processing, 2008, 16(4): 757-765.
[6]. Sedgwick P. Pearson’s correlation coefficient[J]. Bmj, 2012, 345.
[7]. Kim S, Oh B, Kim M, et al. A movie recommendation algorithm combining collaborative filtering and content information[J]. J Korea Inform Sci Soc Softw Appl, 2012, 39(4): 261-268.
[8]. Wang C, Wang H, Pi J, et al. Park recommendation algorithm based on user reviews and ratings[J]. International Journal of Performability Engineering, 2019, 15(3): 803.
[9]. Ze W, Dengwen Z. Optimization collaborative filtering recommendation algorithm based on ratings consistent[C]//2016 7th IEEE International Conference on Software Engineering and Service Science (ICSESS). IEEE, 2016: 1055-1058.
[10]. Zhang J, Peng Q, Sun S, et al. Collaborative filtering recommendation algorithm based on user preference derived from item domain features[J]. Physica A: Statistical Mechanics and its Applications, 2014(396): 66-76.
[11]. Zhang B, Zhang Y, Shen J, et al. Research on differential pulse voltammetry detection method for low concentration glucose based on machine learning model[J]. International Journal of Electrochemical Science, 2024, 19(2): 100479.
Cite this article
Wang,K. (2024). Research on movie recommendation algorithms based on machine learning. Applied and Computational Engineering,71,7-13.
Data availability
The datasets used and/or analyzed during the current study will be available from the authors upon reasonable request.
Disclaimer/Publisher's Note
The statements, opinions and data contained in all publications are solely those of the individual author(s) and contributor(s) and not of EWA Publishing and/or the editor(s). EWA Publishing and/or the editor(s) disclaim responsibility for any injury to people or property resulting from any ideas, methods, instructions or products referred to in the content.
About volume
Volume title: Proceedings of the 6th International Conference on Computing and Data Science
© 2024 by the author(s). Licensee EWA Publishing, Oxford, UK. This article is an open access article distributed under the terms and
conditions of the Creative Commons Attribution (CC BY) license. Authors who
publish this series agree to the following terms:
1. Authors retain copyright and grant the series right of first publication with the work simultaneously licensed under a Creative Commons
Attribution License that allows others to share the work with an acknowledgment of the work's authorship and initial publication in this
series.
2. Authors are able to enter into separate, additional contractual arrangements for the non-exclusive distribution of the series's published
version of the work (e.g., post it to an institutional repository or publish it in a book), with an acknowledgment of its initial
publication in this series.
3. Authors are permitted and encouraged to post their work online (e.g., in institutional repositories or on their website) prior to and
during the submission process, as it can lead to productive exchanges, as well as earlier and greater citation of published work (See
Open access policy for details).