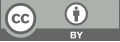
Enhancing chip design verification through AI-powered bug detection in RTL code
- 1 Electrical and Computer Engineering, New York University, NY, USA
- 2 Electrical & Computer Engineering, New York University, NY, USA
- 3 Applied Data Science,University of Southern California,CA,USA
- 4 Computer Science and Engineering , Santa Clara University, CA, USA
- 5 Computer Science and Technology, China University of Geosciences, Beijing, China
* Author to whom correspondence should be addressed.
Abstract
This paper presents a novel AI-driven approach for enhancing chip design verification through automated bug detection in Register Transfer Level (RTL) code. The proposed method integrates advanced machine learning techniques with domain-specific knowledge of chip design to address the challenges of increasing complexity and time-to-market pressures in modern integrated circuit development. Our system employs a comprehensive data preprocessing pipeline that effectively captures syntactic and semantic features of RTL code, feeding into an innovative attention-based neural network model. The model demonstrates superior bug detection accuracy across diverse design categories and bug types compared to traditional methods and existing AI-assisted approaches. Extensive experimental evaluation on a large-scale dataset of RTL designs, including both open-source projects and industry collaborations, validates the effectiveness of our method. The proposed approach achieves a 95% accuracy in bug detection, with a 28-35% reduction in verification time when applied to real-world chip design projects. The paper addresses the interpretability of AI decisions in the context of chip design, presenting novel visualization techniques that enhance trust and facilitate adoption among RTL designers. While acknowledging current limitations, we discuss future research directions, including integration with formal verification methods and extension to system-level verification scenarios. This work contributes significantly to AI-assisted chip design, paving the way for more efficient and reliable development of complex integrated circuits.
Keywords
RTL verification, machine learning, bug detection, chip design automation
[1]. Sankaranarayanan, R., Srinivasan, A., Zaliznyak, A., & Mittai, S. (2021). Chip package co-design and physical verification for heterogeneous integration. In 2021 22nd International Symposium on Quality Electronic Design (ISQED) (pp. 275–280). IEEE.
[2]. Iša, R., Benáček, P., & Puš, V. (2018). Verification of generated RTL from P4 source code. In 2018 IEEE 26th International Conference on Network Protocols (ICNP) (pp. 444-450). IEEE.
[3]. Khoo, K. Y. (2006). Formal verifications in modern chip designs. In 2006 IEEE International High-Level Design and Test Workshop (pp. 38–42). IEEE.
[4]. Sankaranarayanan, R., Srinivasan, A., Zaliznyak, A., & Mittai, S. (2021). Chip package co-design and physical verification for heterogeneous integration. In 2021 22nd International Symposium on Quality Electronic Design (ISQED) (pp. 275–280). IEEE.
[5]. R. Iša, P. Benáček, & V. Puš. (2018). Verification of generated RTL from P4 source code. In 2018 IEEE 26th International Conference on Network Protocols (ICNP) (pp. 444-450). IEEE.
[6]. Li, H., Wang, S. X., Shang, F., Niu, K., & Song, R. (2024). Applications of Large Language Models in Cloud Computing: An Empirical Study Using Real-world Data. International Journal of Innovative Research in Computer Science & Technology, 12(4), 59-69.
[7]. Ping, G., Wang, S. X., Zhao, F., Wang, Z., & Zhang, X. (2024). Blockchain-Based Reverse Logistics Data Tracking: An Innovative Approach to Enhance E-Waste Recycling Efficiency.
[8]. Zhan, X., Shi, C., Li, L., Xu, K., & Zheng, H. (2024). Aspect category sentiment analysis based on multiple attention mechanisms and pre-trained models. Applied and Computational Engineering, pp. 71, 21–26.
[9]. Liu, B., Zhao, X., Hu, H., Lin, Q., & Huang, J. (2023). Detection of Esophageal Cancer Lesions Based on CBAM Faster R-CNN. Journal of Theory and Practice of Engineering Science, 3(12), 36–42.
[10]. Liu, B., Yu, L., Che, C., Lin, Q., Hu, H., & Zhao, X. (2024). Integration and performance analysis of artificial intelligence and computer vision based on deep learning algorithms. Applied and Computational Engineering, pp. 64, 36–41.
[11]. Liu, B. (2023). Based on intelligent advertising recommendations and abnormal advertising monitoring systems in machine learning. International Journal of Computer Science and Information Technology, 1(1), 17–23.
Cite this article
Wang,S.;Zheng,H.;Wen,X.;Xu,K.;Tan,H. (2024). Enhancing chip design verification through AI-powered bug detection in RTL code. Applied and Computational Engineering,92,27-33.
Data availability
The datasets used and/or analyzed during the current study will be available from the authors upon reasonable request.
Disclaimer/Publisher's Note
The statements, opinions and data contained in all publications are solely those of the individual author(s) and contributor(s) and not of EWA Publishing and/or the editor(s). EWA Publishing and/or the editor(s) disclaim responsibility for any injury to people or property resulting from any ideas, methods, instructions or products referred to in the content.
About volume
Volume title: Proceedings of the 6th International Conference on Computing and Data Science
© 2024 by the author(s). Licensee EWA Publishing, Oxford, UK. This article is an open access article distributed under the terms and
conditions of the Creative Commons Attribution (CC BY) license. Authors who
publish this series agree to the following terms:
1. Authors retain copyright and grant the series right of first publication with the work simultaneously licensed under a Creative Commons
Attribution License that allows others to share the work with an acknowledgment of the work's authorship and initial publication in this
series.
2. Authors are able to enter into separate, additional contractual arrangements for the non-exclusive distribution of the series's published
version of the work (e.g., post it to an institutional repository or publish it in a book), with an acknowledgment of its initial
publication in this series.
3. Authors are permitted and encouraged to post their work online (e.g., in institutional repositories or on their website) prior to and
during the submission process, as it can lead to productive exchanges, as well as earlier and greater citation of published work (See
Open access policy for details).