1. Introduction
Since the 1970s, intelligent driving technology has been studied in the United States, England, Germany and other developed countries and has a great process in the feasibility and practicability field. In 2007, Carnegie Mellon University won the first prize in the self-driving car competition, therefore scientists around the world began to study this field and received great progress. However, this technology developed through difficulties. It was a complex subject including vehicle control, road mapping, sensing fusion and so on [1]. Recently, there has been a sensor scarcity in self-driving cars, which has resulted in a number of issues and increased traffic accident risk. Although the car was not completely self-controlled, these kinds of cars all use a self-driving function installed in the car. These vehicles' lack of awareness of the people or objects on the road in front of them caused a misinterpretation and an accident. People's mistrust of self-driving technology is growing as a result of the frequent accidents. It's also becoming more common to question who should bear the blame: the driver or the vehicle. Therefore, research on the car system should develop very fast and become more advanced so that the technologies can be more advanced [2]. Furthermore, the advancement of simulation technology offers an appropriate platform for automobile research. Traditional car tests are expensive, time-consuming, and inefficient. Additional drawbacks should also be noticed, for example, it is sometimes unable to confirm the actual circumstances. In contrast, virtual testing based on simulation can overcome the above difficulties, which reduces the occurrence of traffic accidents and reduces losses [2]. The vibration, temperature change, dust and so on affect the position of the car and the traditional system cannot overcome these challenges. However, the combination of the different information from different sensors can improve the position function in the car. This paper aims to discuss the application of multi-sensor fusion technology in improving the positioning accuracy of robots and the future development of the car in order to provide reference and guidance for the development of robot technology.
2. Sensing technologies and systems applied in intelligent driving
2.1. Monocular sensor
According to the author, additional research revealed that image-based monocular depth estimation is the most important and extensive intelligent driving technology currently in use. Its benefits include low cost, easy installation, and reliable perception [3]. It is popular among all the researchers and has been studied in various fields. Monocular Plan View Networks illustrate a whole view of the road or the environment around the car using a standard front-view image and 3D object localization [4]. The plan view network provides promising results for the reality of the road. The disadvantages of the monocular sensor are also covered by several researchers [3]. It is mentioned that in most of the existing methods of driving path planning using depth estimation, the simple decoding process is related [3]. The potential characteristics of well-featured targets is impossible to be used for monocular depth estimation. They need to consider the complexity of the environment of the actual lane, such as the shadows, water, pavement cracks, etc. It makes achieving both high detection efficiency and robustness more difficult, so they need to optimize the algorithm [5]. For the simulation, the gradient calculation \( Gx(x,y)=l(x+1,y) - l(x - 1,y) \) and \( Gy(x,y)=l(x,y+1) - l(x,y - 1) \) can be used to calculate the gradients and directions of the image processed in horizontal and vertical directions [6]. Then they can calculate the gradients and their directions based on the horizontal and the vertical gradients such as: \( G(x,y)=\sqrt{{Gx(x,y)}^2+{Gy(x,y)}^2} \) .
2.2. Infrared camera sensor
Another camera solution is infrared camera technology. The illumination system in front of the car can let the driver observe the road at night clearly to improve safety when driving late at night, also helps the self-driving car to observe the road better, to reduce the accident. So it can maintain the safety on the road. The driver can adjust the front lighting system and the direction in which the light is directed when other automobiles are approaching from the opposite side in order to prevent hitting other vehicles [7]. The concept of creating a self-changing system is developed since the conventional light control system must be adjusted manually. The camera senses the presence of cars on the other side and uses that information to adjust the system The system includes an infrared camera, radar, vehicle speed sensor and other equipment [7].
2.3. Ultrasound radar sensor
Ultrasonic radar has advantages. Firstly, the distance that ultrasonic radar can work is short but has the benefit of measuring the short distance and it can be used in reversing function. However, it was not suitable to be used in measuring obstacles with long distances that more than 15 meters. The cost of the ultrasonic radar is very low, and its size is really small. The radar is accurate and the sensitivity is higher than others. Ultrasonic radar also has other benefits such as a strong ability to obtain information, a wide range of observing and high resolution. According to Figure 1 below, it can use the ultrasonic transmitter and ultrasonic receiving device to measure the distance between obstacles and the car but it has some error between actual distance and measuring distance. It is assumed that the distance between the vehicle and the surface of the obstacle is L, the propagation speed of the ultrasonic wave in the air is v (340m/s), and the time between sending and receiving the ultrasonic signal is t. Then it will have an equation of L=1/2vt [8].
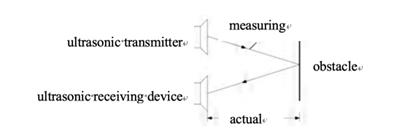
Figure 1. Principle of ultrasonic radar
Vehicle millimeter wave radar usually refers to the radar working in the 30 ~ 300 GHz frequency domain, covering all stages of L1-L4, for the front car collision warning, adaptive cruise, lane change assistance, blind spot monitoring and other auxiliary driving functions. The mainstream frequency band is mainly 24 GHz and 77 GHz two, and the technology and application have been relatively mature. It is not impacted by weather and can give vehicles a range of high-precision road space information, including the relative speed, azimuth, and distance of vehicles in the target region. This information is of great significance for vehicles to actively control speed, avoid other vehicles, and even perform emergency safety measures [9].
2.4. GPS system
At present, China's locomotive positioning system mainly adopts GNSS and BDS global positioning methods. In order to make up for its shortcomings in different projects and different uses, the application of SLAM, INS and other technologies has been enhanced, forming a diversified application. It is believed that the current multi-sensor has made great progress in terms of function, especially the application range of high-precision positioning, inertial navigation positioning, mileage, and other positioning technologies continues to expand under the current situation, laying a solid foundation for the development of the next generation of vehicles with integrated positioning technology. Specifically, the system of Beidou receiver is becoming more and more perfect, the application of inertial sensors and odometer sensors in inertial navigation and positioning technology has increased, and the application of electronic tag technology in the Internet of Things technology has been very popular. Consequently, technical support for the development of locomotive integrated positioning can be obtained from a system that combines Beidou positioning, inertial navigation, an odometer, and an electronic tag [10].
2.5. Car body sensing system
The main method of the global navigation satellite system to complete pedestrian navigation is inertial navigation, but due to the measurement error of the inertial sensor, the navigation error will accumulate continuously, which seriously affects the navigation accuracy. The pedestrian navigation system based on an inertial sensor array can effectively suppress the bias instability of random noise and the accumulation error by combining an inertial sensor array with zero speed correction. The function of fault detection and isolation can also be realized by detecting the output data of the sensor. Thus, different structures of inertial sensor arrays and fusion algorithms are studied. The types of inertial sensor arrays are divided into gyroscope array, accelerometer array and the array formed by the combination of the two. The array of accelerometer can be used to estimate the specific force and angular acceleration of an object according to the proportional relationship between the centrifugal force and the square of the angular velocity. But estimating angular velocity requires diagonal acceleration to be integrated, adding an extra integration step [11].
3. Multi-sensor fusion
There are two types of methods for combining data from multiple inertial sensor arrays: estimation domain fusion and observation domain fusion. Observation domain fusion combines the measured values of multiple inertial sensors into one measured value by weighted summation. A traditional single Inertial Measurement Unit (IMU) sensor estimator is adopted and its estimate is used as the estimate for data fusion. This is the most common approach to the inertial sensor array data fusion problem. It is also the most widely studied method at present. The disadvantage of this approach is that when the sensors have different specifications and require precise timing, it is difficult to explain the bias and error characteristics of a single sensor. It is suggested to combine several sensors to create one that is more sensitive and has many benefits in order to solve certain issues.
The development of multi-sensor algorithms is not only to improve the accuracy of the system but also to deal with more complex and changeable environmental challenges in the real world. The traditional single-sensor algorithm has some limitations in dealing with a complex environment, dynamic scene and sensor error. Therefore, using multiple sensor data fusion has become an effective way to solve these problems [10]. Due to the introduction of multiple sensors, the observation information of each sensor may not be obtained at the same time, so the fusion of sensor data has asynchronous problems. To solve this problem, the fusion algorithm based on a factor graph is proposed in this paper. Factor graph model is a graph theory tool used to model and solve the joint probability distribution in complex systems. Its theoretical basis is derived from the probability graph model. Factor graph model can effectively solve the asynchronous problem in data fusion, and has good scalability for multiple sensors, and can flexibly configure sensors [12].
The first to address is the camera and the radar combination. The fusion technology of vision and radar has become an important means to improve positioning accuracy. Specifically, vision sensors can accurately identify the surface of an object by capturing information such as its color, shape and texture. The radar sensor can obtain the three-dimensional structure and position information of the object by emitting laser pulses and measuring the reflected signal, and the information of the two sensors can be fused, which can complement each other and further improve the positioning accuracy of industrial robots. The specific methods of fusion of vision and radar sensors are as follows. Firstly, the data collected by the vision sensor and radar sensor are prepossessed, such as denouncing and calibration. Secondly, visual information is associated with radar information through feature extraction and matching. Ultimately, in order to achieve precise object surface recognition and three-dimensional location, the three-dimensional data from the radar is utilized to enhance and optimize the visual data [11].
Secondly, the fusion technology of ultrasonic sensors and radar was created by allowing the two to come together in the field of industrial robots to improve the positioning accuracy of robots in complex environments. The ultrasonic sensor can effectively identify the obstacles in a short distance to make up for the shortcomings of radar. In a small space, the robot needs to rely on high-precision positioning to complete the task. At this time, the ultrasonic sensor can provide real-time distance information, which is combined with the radar data to greatly improve the positioning accuracy of the robot. Ultrasonic sensors can monitor the environment around the robot in real time and provide immediate feedback when obstacles are encountered. Meanwhile, radar is responsible for scanning the distant environment to obtain a broader field of view. Data fusion of the two sensors can make the positioning of the robot in a narrow space more accurate. By processing the information of the two sensors, the positioning error can be effectively reduced and the machine can improve human operational stability [12].
Although above combinations had solved a lot of problems, there are still some drawbacks that can be improved. It is particularly important to strengthen the theoretical exploration and practical application of data fusion algorithm. In terms of accuracy, researchers should strive to improve the anti-interference ability of the algorithm to noise and outliers, and improve the accuracy of data fusion. The adaptive ability of the algorithm cannot be ignored, and it should be able to automatically adjust parameters and processing methods according to different working environments and task requirements, to improve the working efficiency and stability of industrial robots, and further promote the development of industrial automation and intelligence [13]. With the continuous development of technology and the reduction of cost, the application of multi-sensor fusion technology in the field of industrial robot positioning will be more widely developed. By strengthening research and development investment and optimizing the algorithm, the positioning accuracy and reliability can be further improved. At the same time, the standardization of sensor interfaces and the establishment of test and evaluation platforms will help simplify the system integration and maintenance process, improve the stability and flexibility of the system. For future applications, the vehicles need to do more tests to make themselves more acceptable to humans.
4. Conclusion
Young people are growing increasingly interested in self-driving cars. More and more people are willing to purchase vehicles equipped with this technology and experience the convenience. It seems like a new fashion but is not yet being widely used nowadays. Self-driving cars are becoming a new mode of transportation outside, especially with the fast development of vision and robot technology. Sensor-based perception technology has developed rapidly but is relatively slow in the field of planning and control compared to visual processing. This article explores the reason for developing this technology. The article primarily explains different sensors used in cars as well as some drawbacks to using just one of these types of sensors. A single sensor performs a limited support to the driving and the decision-making and emergency-dealing process are not ideal. After a thorough analysis of other studies, this paper proposes that combining several types of sensors could improve performance.
References
[1]. Wang, J., Huang, H., Zhi, P., Shen, Z., & Zhou, Q. (2019). Review of development and key technologies in automatic driving. Application of Electronic Technique, 45(6), 28-36.
[2]. Wang, R., Wu, J., & Xu, H. (2022). Overview of Research and Application on Autonomous Vehicle Oriented Perception System Simulation. Journal of System Simulation, 34(12), 2507-2521.
[3]. Zhang, Y., Cui, Z, Wang, X., Xun,J., Hu,Y., &Dan. (2023). Intelligent driving path planning method based on monocular depth estimation. Foreign Electronic Measurement Technology, 42(8), 71-79.
[4]. Wang, D., Devin, C., Cai, Q. Z., Krähenbühl, P., & Darrell, T. (2019, November). Monocular plan view networks for autonomous driving. In 2019 IEEE/RSJ International Conference on Intelligent Robots and Systems (IROS) (pp. 2876-2883). IEEE.
[5]. Miao, X., Li, S., & Shen, H. (2012). On-board lane detection system for intelligent vehicle based on monocular vision. International journal on smart sensing and intelligent systems, 5(4), 957-972.
[6]. Zhang, B., Li, L., Cheng, S., Zhang, W., Li, S., & **ao, L. (2019). Research on Obstacle Avoidance of Intelligent Driving Vehicles Based on Monocular Camera. In CICTP 2019 (pp. 5576-5586).
[7]. Lan, J., & Yang, C., (2019). Adaptive Far Light Control System Based on Infrared Camera Technology. Automobile Applied Technology, 9, 66-67.
[8]. Yang, S., (2024). Application analysis of ultrasonic radar in intelligent networked vehicles. Automotive Industry Research, 1, 51-54.
[9]. Yan, L., Chen, C., & Han, X., (2023). Research on Automatic Parking Perception System Based on Ultrasonic Sensor and Millimeter Wave Radar. Practical Technology of Automobile, 40-44.
[10]. Li, Z., Chang, Y., & Liu, Y., (2024). Research on locomotive fusion positioning system based on multiple sensors. Electronic Components and Information Technology, (01), 64-67.
[11]. Luo, X., Liu, L., Cao, L., & Wang, Z., (2023). A review of the development of pedestrian navigation based on inertial sensor arrays. Journal of Ordnance Engineering, (12), 151-158.
[12]. Xu, Y., (2024) Research and Implementation of Environment Sensing Algorithm for Quadruped Robot Based on Multi-Sensor Fusion. (Master, Thesis, Qilu University of Technology).
[13]. Xu, Y., (2024) Application of multi-sensor fusion technology in improving positioning accuracy of industrial robots. Paper Making Equipment and Materials, (06), 36-38.
Cite this article
Li,Y. (2024). Review on Intelligent Driving Schemes Based on Different Sensors. Applied and Computational Engineering,93,29-34.
Data availability
The datasets used and/or analyzed during the current study will be available from the authors upon reasonable request.
Disclaimer/Publisher's Note
The statements, opinions and data contained in all publications are solely those of the individual author(s) and contributor(s) and not of EWA Publishing and/or the editor(s). EWA Publishing and/or the editor(s) disclaim responsibility for any injury to people or property resulting from any ideas, methods, instructions or products referred to in the content.
About volume
Volume title: Proceedings of the 2nd International Conference on Machine Learning and Automation
© 2024 by the author(s). Licensee EWA Publishing, Oxford, UK. This article is an open access article distributed under the terms and
conditions of the Creative Commons Attribution (CC BY) license. Authors who
publish this series agree to the following terms:
1. Authors retain copyright and grant the series right of first publication with the work simultaneously licensed under a Creative Commons
Attribution License that allows others to share the work with an acknowledgment of the work's authorship and initial publication in this
series.
2. Authors are able to enter into separate, additional contractual arrangements for the non-exclusive distribution of the series's published
version of the work (e.g., post it to an institutional repository or publish it in a book), with an acknowledgment of its initial
publication in this series.
3. Authors are permitted and encouraged to post their work online (e.g., in institutional repositories or on their website) prior to and
during the submission process, as it can lead to productive exchanges, as well as earlier and greater citation of published work (See
Open access policy for details).
References
[1]. Wang, J., Huang, H., Zhi, P., Shen, Z., & Zhou, Q. (2019). Review of development and key technologies in automatic driving. Application of Electronic Technique, 45(6), 28-36.
[2]. Wang, R., Wu, J., & Xu, H. (2022). Overview of Research and Application on Autonomous Vehicle Oriented Perception System Simulation. Journal of System Simulation, 34(12), 2507-2521.
[3]. Zhang, Y., Cui, Z, Wang, X., Xun,J., Hu,Y., &Dan. (2023). Intelligent driving path planning method based on monocular depth estimation. Foreign Electronic Measurement Technology, 42(8), 71-79.
[4]. Wang, D., Devin, C., Cai, Q. Z., Krähenbühl, P., & Darrell, T. (2019, November). Monocular plan view networks for autonomous driving. In 2019 IEEE/RSJ International Conference on Intelligent Robots and Systems (IROS) (pp. 2876-2883). IEEE.
[5]. Miao, X., Li, S., & Shen, H. (2012). On-board lane detection system for intelligent vehicle based on monocular vision. International journal on smart sensing and intelligent systems, 5(4), 957-972.
[6]. Zhang, B., Li, L., Cheng, S., Zhang, W., Li, S., & **ao, L. (2019). Research on Obstacle Avoidance of Intelligent Driving Vehicles Based on Monocular Camera. In CICTP 2019 (pp. 5576-5586).
[7]. Lan, J., & Yang, C., (2019). Adaptive Far Light Control System Based on Infrared Camera Technology. Automobile Applied Technology, 9, 66-67.
[8]. Yang, S., (2024). Application analysis of ultrasonic radar in intelligent networked vehicles. Automotive Industry Research, 1, 51-54.
[9]. Yan, L., Chen, C., & Han, X., (2023). Research on Automatic Parking Perception System Based on Ultrasonic Sensor and Millimeter Wave Radar. Practical Technology of Automobile, 40-44.
[10]. Li, Z., Chang, Y., & Liu, Y., (2024). Research on locomotive fusion positioning system based on multiple sensors. Electronic Components and Information Technology, (01), 64-67.
[11]. Luo, X., Liu, L., Cao, L., & Wang, Z., (2023). A review of the development of pedestrian navigation based on inertial sensor arrays. Journal of Ordnance Engineering, (12), 151-158.
[12]. Xu, Y., (2024) Research and Implementation of Environment Sensing Algorithm for Quadruped Robot Based on Multi-Sensor Fusion. (Master, Thesis, Qilu University of Technology).
[13]. Xu, Y., (2024) Application of multi-sensor fusion technology in improving positioning accuracy of industrial robots. Paper Making Equipment and Materials, (06), 36-38.