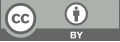
Predicting Movie Box Office Based on Machine Learning, Deep Learning, and Statistical Methods
- 1 School of Science, Beijing Jianzhu University, Beijing, 100000, China
* Author to whom correspondence should be addressed.
Abstract
The global film industry has been proved to impose a significant impact on both culture and the economy, with box office revenue serving as a crucial indicator of a film's commercial success. This study utilizes data from the Kaggle "TMDB Box Office Prediction" competition, encompassing 3,000 films released between 1990 and 2018, to predict movie box office revenue using Random Forest, XGBoost algorithms, and deep learning models such as Bidirectional Long Short-Term Memory (Bidirectional LSTM) and Simple Recurrent Neural Network (SimpleRNN). The goal is to develop a model that accurately predicts movie box office. By comprehensively considering multiple factors such as budget, popularity and film characteristics, this study not only significantly improves the accuracy of box office prediction, but also provides a scientific basis for the formulation of film market strategies. The results demonstrate that the Bidirectional LSTM excels in handling complex time-series data, showing strong trend-capturing capabilities, while XGBoost exhibits greater robustness in dealing with complex data and outliers. These findings can not only provide guidance for making more effective strategies on film production and distribution, but also provide new directions for future research, such as delving into the impact of social media on box office and developing more sophisticated predictive models to adapt to changing market dynamics.
Keywords
Machine learning, deep learning, statistical methods, movie box office.
[1]. Litman B R and Kohl L S 1989 Predicting financial success of motion pictures: the '80s experience. Journal of Media Economics, 2(2), 35-50.
[2]. Jehoshua E, Jedid-Jah J, Mohanbir S S and Berend W 2000 MOVIEMOD: An Implementable Decision Support System for Prerelease Market Evaluation of Motion Pictures. Marketing Science, 19(3), 226-243.
[3]. Dellarocas C and Wood C A 2008 The sound of silence in online feedback: Estimating trading risks in the presence of reporting bias. Management Science, 54(3), 460-476.
[4]. Cheung C M and Thadani D R 2012 The impact of electronic word-of-mouth communication: A literature analysis and integrative model. Decision Support Systems, 54(1), 461-470.
[5]. Yao W 2018 A Study on the Box Office Characteristics of Domestic Films in the Chinese Mainland Market and Their Influencing Factors: An Empirical Analysis Based on the PLS Model. Price Theory and Practice, 2, 83-86.
[6]. Hua R, Wang S and Xu Y 2019 A Study on the Influencing Factors of Chinese Film Box Office. Statistics and Decision, 4, 97-100.
[7]. Zhou H 2021 Research and Implementation of Movie Box Office Analysis Based on Machine Learning. Beijing University of Posts and Telecommunications.
[8]. He Q and Hu B 2021 Research on the influencing factors of film consumption and box office forecast in the digital era: based on the perspective of machine learning and model integration. Wireless Communications and Mobile Computing, 1, 6094924.
[9]. Dai D and Chen J 2021 Research on mathematical model of box office forecast through BP neural network and big data technology. Journal of Physics: Conference Series, 1952(4), 042118.
[10]. Yan X 2022 Research on Movie Box Office Prediction Based on Machine Learning. University of International Business and Economics.
[11]. Lu H 2022 Research on Movie Box Office Prediction Based on Ensemble Learning. Anhui University.
[12]. Yao L 2022 Prediction of Domestic Film Box Office Based on XGB-CatBoost Combined Model. Zhongnan University of Economics and Law.
[13]. Han X 2023 Research on Movie Box Office Prediction Based on Stacking Ensemble and Hyperparameter Optimization. Shanxi University.
[14]. Fang L 2023 Movie Box Office Data Prediction Based on GBRT-Stacking Ensemble Learning Algorithm. Shanghai Normal University.
[15]. Madongo C T and Zhongjun T 2023 A movie box office revenue prediction model based on deep multimodal features. Multimedia Tools and Applications, 82(21), 31981-32009.
[16]. Chen X 2024 Movie Box Office Prediction Based on Machine Learning. North China University of Technology.
[17]. Cha C 2024 Research on Hollywood Movie Box Office Prediction Based on BP Neural Network. Inner Mongolia University of Finance and Economics.
Cite this article
Zheng,Y. (2024). Predicting Movie Box Office Based on Machine Learning, Deep Learning, and Statistical Methods. Applied and Computational Engineering,94,20-32.
Data availability
The datasets used and/or analyzed during the current study will be available from the authors upon reasonable request.
Disclaimer/Publisher's Note
The statements, opinions and data contained in all publications are solely those of the individual author(s) and contributor(s) and not of EWA Publishing and/or the editor(s). EWA Publishing and/or the editor(s) disclaim responsibility for any injury to people or property resulting from any ideas, methods, instructions or products referred to in the content.
About volume
Volume title: Proceedings of CONF-MLA 2024 Workshop: Securing the Future: Empowering Cyber Defense with Machine Learning and Deep Learning
© 2024 by the author(s). Licensee EWA Publishing, Oxford, UK. This article is an open access article distributed under the terms and
conditions of the Creative Commons Attribution (CC BY) license. Authors who
publish this series agree to the following terms:
1. Authors retain copyright and grant the series right of first publication with the work simultaneously licensed under a Creative Commons
Attribution License that allows others to share the work with an acknowledgment of the work's authorship and initial publication in this
series.
2. Authors are able to enter into separate, additional contractual arrangements for the non-exclusive distribution of the series's published
version of the work (e.g., post it to an institutional repository or publish it in a book), with an acknowledgment of its initial
publication in this series.
3. Authors are permitted and encouraged to post their work online (e.g., in institutional repositories or on their website) prior to and
during the submission process, as it can lead to productive exchanges, as well as earlier and greater citation of published work (See
Open access policy for details).