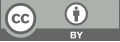
Dynamic pricing and demand forecasting: Integrating time-series analysis, regression models, machine learning, and competitive analysis
- 1 Beijing University of Posts and Telecommunications, Beijing, China
- 2 University College London, London, United Kingdom of Britain
- 3 University of New South Wales, Sydney, Australia
* Author to whom correspondence should be addressed.
Abstract
Dynamic pricing is a critical strategy for businesses seeking to optimize revenue and stay competitive in fluctuating markets. This paper explores the integration of various demand forecasting techniques, including time-series analysis, regression models, and machine learning algorithms, with competitive analysis methodologies to enhance dynamic pricing strategies. Time-series analysis focuses on decomposing data into trend, seasonality, and random fluctuations, using ARIMA models for accurate demand prediction. Regression models delve into the complexities of variable interactions, extending beyond linear relationships to include advanced techniques like Ridge, Lasso, and Elastic Net regression. Machine learning algorithms, such as decision trees, random forests, gradient boosting, and neural networks, revolutionize demand forecasting by uncovering complex patterns in large datasets. Competitive analysis incorporates market scanning, price elasticity estimation, and competitor behavior modeling to inform dynamic pricing decisions. Optimization algorithms, including linear programming, genetic algorithms, and simulated annealing, are employed to refine pricing strategies, while revenue management techniques like yield management and overbooking ensure maximum revenue from perishable goods and services. This comprehensive approach enables businesses to dynamically adjust prices in real-time, maintaining competitiveness and maximizing profitability in an ever-evolving market landscape.
Keywords
Dynamic pricing, demand forecasting, time-series analysis, ARIMA
[1]. Shin, Dongwook, Stefano Vaccari, and Assaf Zeevi. "Dynamic pricing with online reviews." Management Science 69.2 (2023): 824-845.
[2]. Sarkar, Malay, et al. "Optimizing E-Commerce Profits: A Comprehensive Machine Learning Framework for Dynamic Pricing and Predicting Online Purchases." Journal of Computer Science and Technology Studies 5.4 (2023): 186-193.
[3]. Varma, Sushil Mahavir, et al. "Dynamic pricing and matching for two-sided queues." Operations Research 71.1 (2023): 83-100.
[4]. Sanders, Robert Evan. "Dynamic pricing and organic waste bans: A study of grocery retailers’ incentives to reduce food waste." Marketing Science 43.2 (2024): 289-316.
[5]. Aparicio, Diego, and Kanishka Misra. "Artificial intelligence and pricing." Artificial intelligence in marketing (2023): 103-124.
[6]. Schittekatte, Tim, et al. "Electricity retail rate design in a decarbonizing economy: an analysis of time-of-use and critical peak pricing." The Energy Journal 45.3 (2024): 25-56.
[7]. Sarfarazi, Seyedfarzad, et al. "An optimal real-time pricing strategy for aggregating distributed generation and battery storage systems in energy communities: A stochastic bilevel optimization approach." International Journal of Electrical Power & Energy Systems 147 (2023): 108770.
Cite this article
Ma,Q.;Feng,S.;Liu,J. (2024). Dynamic pricing and demand forecasting: Integrating time-series analysis, regression models, machine learning, and competitive analysis. Applied and Computational Engineering,93,149-154.
Data availability
The datasets used and/or analyzed during the current study will be available from the authors upon reasonable request.
Disclaimer/Publisher's Note
The statements, opinions and data contained in all publications are solely those of the individual author(s) and contributor(s) and not of EWA Publishing and/or the editor(s). EWA Publishing and/or the editor(s) disclaim responsibility for any injury to people or property resulting from any ideas, methods, instructions or products referred to in the content.
About volume
Volume title: Proceedings of the 2nd International Conference on Machine Learning and Automation
© 2024 by the author(s). Licensee EWA Publishing, Oxford, UK. This article is an open access article distributed under the terms and
conditions of the Creative Commons Attribution (CC BY) license. Authors who
publish this series agree to the following terms:
1. Authors retain copyright and grant the series right of first publication with the work simultaneously licensed under a Creative Commons
Attribution License that allows others to share the work with an acknowledgment of the work's authorship and initial publication in this
series.
2. Authors are able to enter into separate, additional contractual arrangements for the non-exclusive distribution of the series's published
version of the work (e.g., post it to an institutional repository or publish it in a book), with an acknowledgment of its initial
publication in this series.
3. Authors are permitted and encouraged to post their work online (e.g., in institutional repositories or on their website) prior to and
during the submission process, as it can lead to productive exchanges, as well as earlier and greater citation of published work (See
Open access policy for details).