1. Introduction
Nowadays, there are various ways to track the motion status of robots in the system, and estimating their motion status is a challenge. Robots need to know their position, speed, and attitude information in real time during motion. The commonly used method to solve such problems in previous research was to add multiple sensors, such as Global Positioning System (GPS), Inertial Measurement Unit, and camera [1]. However, when fusing data from multiple sensors in many real-world situations, the interference of noise can also be amplified. Kalman filtering has a very good effect on processing auto-correlation data.
From the above, Kalman filtering has significant advantages in processing multi type or complex data in Robot Simultaneous Localization and Mapping (SLAM) technology [2,3], which is also an important motivation for its application in robot path SLAM planning in this article. This article aims to use the Extended Kalman Filtering (EKF) algorithm to process the fused data and estimate the motion state of the robot. One of the challenges in data fusion today is precisely this, where the differences in data between different types of data directly affect the accuracy and robustness of data fusion [4].
This article aims to use the extended Kalman filtering algorithm to process the fused data and estimate the motion state of the robot. At the same time, this article expects to use auto-correlation databases as the analysis object; Add specific noise to the data during fusion; Using the extended Kalman filtering algorithm to process it, the estimated data is compared with the original data, and a feasible estimation method for data fusion is analyzed and obtained.
2. Literature Review
The Kalman filter proposed by Hungarian scientist Rudolf E. Kalman in 1960 has a wide range of applications in modern engineering [5]. Kalman filtering is often used in systems that cannot be directly measured due to its unique and efficient recursive nature, as well as its ability to estimate dynamic system states from a series of measurements containing noise, for example,
(1) It is used for temperature measurement in surgical endoscopic robot, which can be used to estimate the temperature in complex situations. It is difficult for instruments to directly measure the local temperature inside the human body cavity, and the actual measurement value has a significant deviation from the true value. However, the use of Kalman filtering significantly improves the accuracy of temperature monitoring [6].
(2) It used for robot battery monitoring, the internal of the battery is a complex physical chemical system, which means that it is impossible to directly measure the battery's state during battery use. However, using Kalman filtering can accurately and real-time estimate the battery's charging and discharging state [7,8].
(3) Improvement of sensors for complex situations [9,10]. In most complex situations, the data of various sensors working together is diverse, difficult to coordinate, and the resistance of the data to noise after fusion is very weak. Using Kalman filtering can effectively reduce the impact of modeled noise on the system and improve estimation accuracy.
3. Methodology
3.1. Experimental Method
EKF is not uncommon in SLAM, and some application scenarios have been introduced in the previous text. This article sets the experimental scenario as the movement of robots in indoor environments, and designs experiments related to SLAM around this scenario [11,12]. For this study, based on the existing conditions, this article prefers to use simulation experiments as the basis, model the extended Kalman filter in MATLAB software, and import data for experiments. The main purpose is to carry out experimental activities based on this.
The essence of the EKF and the Kalman Filter (KF) is the same, with one difference being that Extended Kalman Filter requires the addition of a discrete matrix in the Kalman Filter. Therefore, this article first introduces the basic principle of its operation.
In the introduction, it has been mentioned that the extended Kalman filter itself is a dynamic equation, and this algorithm has a very obvious feature, that is, it follows the order of "prediction – correction -prediction". And then, the following text will provide a detailed introduction to the basic principles of its work.
It is worth noting that observations are used to correct predicted values, and the weight they occupy during correction is the Kalman filter gain. The Kalman gain determines the combination of predicted and measured values. The larger the gain, the smaller the weight of the corrected value, which means that the credibility and usability of the output value are higher. That is to say, there are two situations. (1) If the prediction error is large, the Kalman gain will rely more on the measured values to update the state. (2) If the observation noise is large, the Kalman gain will rely more on the predicted value to update the state.
3.2. Experimental Design
Based on the above basic principles, the basic process of designing experiments can be clarified
1. Establish the basic program for extended Kalman filtering in MATLAB software,
2. Identify the basic parameters, they should be corresponded one-by-one with the parameters in the program.
3. Import known data in order and output estimated data
4. Compare the error between the original data and the existing data
This experiment is mainly divided into three stages: (1) Equipment preparation. Mainly including the programming software and experimental data before the experiment, the programming software and simulation software selected for this experiment are MATLAB 2019b; (2) EKF implementation process. Firstly, initialize the parameters and define them; Then import various parameters; Finally, the parameters are iterated in EKF; (3) Output data and compare images. Visualizing and digitizing the above results facilitates subsequent analysis and discussion of the results.
3.3. Experimental implementation
3.3.1. Data Process. It is difficult to find a very suitable auto-correlation data set in this experiment, that is, data with similar characteristics collected from different sensors on a device, such as linear velocity, angular velocity, acceleration; Relative altitude, absolute altitude, coordinate information (longitude and latitude); Or it could be a radar image compared to a camera image. That means improving the auto-correlation of data is an important part. This article breaks down multiple data into different dimensions for analysis, and classifies and coordinates them based on these dimensions to enhance the correlation between data. So, the data used in this article is a dataset of linear velocity, angular velocity, and distance from a robot; At the same time, simulation methods were also used to obtain data.
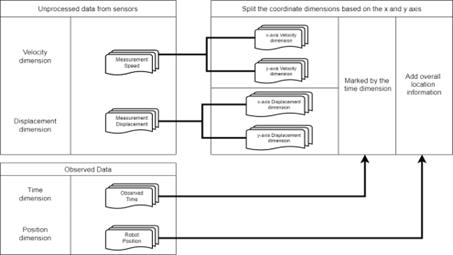
Figure 1. The process of establishing data correlation
As shown in Figure 1, this is a method proposed in this article that relies on data correlation to normalize different data. (1) Firstly, this article will split the unprocessed data into two categories: observation dataset and sensor dataset. The sensor dataset contains unprocessed data which has correlation, while the observation dataset contains data with weak correlation but distinctive features. (2) Subsequently, this article proposes to split the correlation data into spatial dimensions (different types of sensor data correspond to different dimensions) to extract similar features between different data. For example, the data used in this article can be vectorized on the coordinate axis and split into different components on the x and y axis. (3) Then, the time data is used as landmark data to mark and organize the split data, so that all data has timestamps. (4) The final step of data processing is to import special observation data, which is used to correct the estimated data in the EKF mentioned earlier, that is, to proofread the split data during the estimation process and fuse the new dataset.
3.3.2. Simulation. The simulation part of the program is used to generate data, thereby reducing the actual data collection process. However, this may differ from the actual situation, in order to avoid this situation from occurring and affecting the accuracy of the results, random noise was manually added to simulate the real situation in this part of the experiment.
3.3.3. Core Program. The core program is evolved from the core formula of EKF, and the entire filter is an iterative process. Based on the filtering result of the previous frame and the input state, the state and state covariance are first predicted, and then the input updates the state and covariance to obtain the updated state. After multiple frame updates, more accurate results can be obtained. It is worth noting that this article improved the noise matrix in the original EKF model during the experimental process, which varies with time. Through this improvement. In this experiment, the influence of Gaussian noise on experimental data in real environments can be simulated to a certain extent. Because the covariance matrix is used to describe the uncertainty of position and velocity, and is continuously updated through filters, this modification can improve the distortion of some data during the covariance prediction update process.
4. Results
![]()
|
(a) |
![]()
|
(b) |
![]()
|
(c) |
Figure 2. (a) Robot displacement trajectory at different stages (b) Actual GPS location and estimated GPS location (c) Error
Figure 2 shows the results which are from experiments by using real data.
Figure 2(a) is robot displacement trajectory at different stages. It shows the trajectory of robot motion at different stages in the case of real data, with the green curve representing the true optimal estimated trajectory; The red curve represents the observation trajectory; The blue curve represents the prior estimated trajectory.
Figure 2(b) is actual GPS location and estimated GPS location. It shows the actual GPS location and estimated GPS location. The red curve represents the original GPS location information, and the blue curve represents the estimated GPS location information
Figure 2(c) is Error between actual GPS location and estimated GPS location. It shows the difference between the actual GPS location and the GPS estimated location.
![]()
|
(a) |
![]()
|
(b) |
Figure 3. (a) Simulation GPS location and estimated GPS location (b) Error
Figure 3 shows the results which is from experiments by using simulation data.
Figure 3(a)shows the simulation GPS location and estimated GPS location. The red curve represents the original simulated GPS data, while the blue curve represents the estimated GPS data.
Figure 3(b) is Error between simulation GPS location and estimated GPS location. It shows the difference between the actual GPS location and the GPS estimated location. (The experiments simulated in this section serve as the reference group for this experiment and can adjust internal parameters on their own, so they are not considered as specific analysis objects).
5. Discussion
After several steps of updating, the estimated value of the EKF filter can gradually approach the true value, and the error can reach a stable range over time.
Regarding the display in the above image, it can be observed that after the measurement data is used to modify the prediction results, the estimated results gradually approach the actual results through continuous iteration. In general, the EKF estimated results are close to the actual location information. In the estimation process, the convergence of the data gradually increases, and the errors start from the beginning (0.126539408, 0.195391733, 0.024811649, 0.093043682, 0.456416361) and even higher values, gradually reaching the optimal value in the (0.002699985, 0.045229858, 0.002159863, 0.001281849, 0.010885512).
The EKF filter can still provide stable and reliable state estimation in the face of noise, uncertainty, model errors, and sensor failures.
After adding noise, the filter can still reach a stable estimation state after iteration for a period of time. In the experiment, there was a clear peak at 4.2s, which was clearly due to a strong interference on the data during this period. However, the estimation results quickly returned to the normal state.
Based on the analysis of existing experimental results and processes, there are some points for the development of this experiment; (1) Most of the errors in the path trajectory estimation process come from the accumulation and amplification of errors caused by the failure to make timely corrections after high fluctuation data occurs. This kind of fluctuation is usually caused by sudden changes in the posture of the experimental object during driving. So modifying the covariance matrix can make the original EKF model converge better in high volatility data and improve the convergence during estimation. In addition, changing the Kalman gain in the EKF method calculation can reduce the occurrence of high error estimation results and improve the robustness of the estimation results. (2) Meanwhile, through this experiment and the review of relevant materials, by introducing adaptive algorithms and utilizing their adaptability [13], the constantly changing noise characteristics in the system can be solved on the basis of this experiment, and the error sources can be modeled and adjusted more finely.
6. Conclusion
In most cases, the estimated data is very close to the actual situation, which proves that EKF can play a very effective role in strong correlation data fusion. This is because the differences in auto-correlation data are small, and the weights in the Kalman gain are more credible, moreover, the improved EKF can help improve the accuracy of state estimation and the robustness of filters, making it better suited for practical complex systems. However, in some cases, there is a significant gap between 2 different type data, this gap will affect the accuracy of the estimation. This proves that it is very necessary to classify multiple strongly correlated data into one category before data fusion in this article. Compared to process data fusion blindly, this classification method will greatly reduce the impact of special or large difference data.
Through the above improvement directions, this article hopes to expand this model to higher dimensional data fusion fields and apply different levels of data to individual robot path planning. In the future, with the continuous development of deep learning, graph optimization, multi robot collaboration, strong learning, and new sensor technologies, based on the conclusions and analysis of this article, SLAM technology will be further improved and data fusion technology will be used to achieve more efficient and reliable positioning and map construction in various complex environments in the future.
References
[1]. Mariga, L., Nascimento, C. L. & Dos Santos, S. R. B. (2024). 3D EKF-SLAM Using Cylindrical and Planar Features with Multilevel Mapping Representation, 2024 IEEE International Systems Conference (Montreal, QC, Canada), pp. 1-8.
[2]. Ok, M., Ok, S., & Park, J. H. (2021). Estimation of Vehicle Attitude, Acceleration, and Angular Velocity Using Convolutional Neural Network and Dual Extended Kalman Filter. Sensors (Basel, Switzerland), 21(4), 1282.
[3]. Rossi, Y., Tatsis, K., Awadaljeed, M., Arbogast, K., Chatzi, E., Rothacher, M., & Clinton, J. (2021). Kalman Filter-Based Fusion of Collocated Acceleration, GNSS and Rotation Data for 6C Motion Tracking. Sensors (Basel, Switzerland), 21(4), 1543.
[4]. Jiang, P., Hu, C., Wang, T., Lv, K., Guo, T., Jiang, J., Hu, W. (2024). Research on a Visual/Ultra-Wideband Tightly Coupled Fusion Localization Algorithm. Sensors, 24, 1710.
[5]. Vladimír, K. (2017). Rudolf E. Kalman: Life and Works. IFAC-PapersOnLine, 50(1), 631-636.
[6]. VilasBoas-Ribeiro, I., Nouwens, S. A. N., Curto, S., Jager, B., Franckena, M., Rhoon, G. C., Heemels, W. P. M. H., & Paulides, M. M. (2022). POD-Kalman filtering for improving noninvasive 3D temperature monitoring in MR-guided hyperthermia. Medical physics, 49(8).
[7]. Hu, L., Hu, R., Ma, Z., & Jiang, W. (2022). State of Charge Estimation and Evaluation of Lithium Battery Using Kalman Filter Algorithms. Materials (Basel, Switzerland), 15(24), 8744.
[8]. Lin, Q., Li, X., Tu, B., Cao, J., Zhang, M., & Xiang, J. (2023). Stable and Accurate Estimation of SOC Using eXogenous Kalman Filter for Lithium-Ion Batteries. Sensors (Basel, Switzerland), 23(1), 467.
[9]. Wang, J., Pan, Z., Li, B. and Wang, R. (2023). Terrain Estimation for Quadruped Robots Based on Kalman Filter, 2023 8th International Conference on Intelligent Computing and Signal Processing (Xi'an, China), pp. 747-754.
[10]. Santana, B., Cherif, E. K., Bernardino, A., & Ribeiro, R. (2022). Real-Time Georeferencing of Fire Front Aerial Images Using Iterative Ray-Tracing and the Bearings-Range Extended Kalman Filter. Sensors (Basel, Switzerland), 22(3).
[11]. Li, N., Gao, Y., Wang, Y., Liu, Z., Guan, L., & Liu, X. (2019). A Low-Cost Underground Garage Navigation Switching Algorithm Based on Kalman Filtering. Sensors (Basel, Switzerland), 19(8), 1861.
[12]. Eman, A., & Ramdane, H. (2020). Mobile Robot Localization Using Extended Kalman Filter. 2020 3rd International Conference on Computer Applications & Information Security (Riyadh, Saudi Arabia), pp. 1-5.
[13]. Hou, K., & Tang, Y. (2023). Visual Positioning System of Intelligent Robot Based on Improved Kalman Filter. 2023 China Automation Congress (Chongqing, China), pp. 5804-5808.
Cite this article
Li,G. (2024). A modified extended Kalman filter based fusion of auto-correlation data for robot SLAM estimation. Applied and Computational Engineering,81,33-40.
Data availability
The datasets used and/or analyzed during the current study will be available from the authors upon reasonable request.
Disclaimer/Publisher's Note
The statements, opinions and data contained in all publications are solely those of the individual author(s) and contributor(s) and not of EWA Publishing and/or the editor(s). EWA Publishing and/or the editor(s) disclaim responsibility for any injury to people or property resulting from any ideas, methods, instructions or products referred to in the content.
About volume
Volume title: Proceedings of the 2nd International Conference on Machine Learning and Automation
© 2024 by the author(s). Licensee EWA Publishing, Oxford, UK. This article is an open access article distributed under the terms and
conditions of the Creative Commons Attribution (CC BY) license. Authors who
publish this series agree to the following terms:
1. Authors retain copyright and grant the series right of first publication with the work simultaneously licensed under a Creative Commons
Attribution License that allows others to share the work with an acknowledgment of the work's authorship and initial publication in this
series.
2. Authors are able to enter into separate, additional contractual arrangements for the non-exclusive distribution of the series's published
version of the work (e.g., post it to an institutional repository or publish it in a book), with an acknowledgment of its initial
publication in this series.
3. Authors are permitted and encouraged to post their work online (e.g., in institutional repositories or on their website) prior to and
during the submission process, as it can lead to productive exchanges, as well as earlier and greater citation of published work (See
Open access policy for details).
References
[1]. Mariga, L., Nascimento, C. L. & Dos Santos, S. R. B. (2024). 3D EKF-SLAM Using Cylindrical and Planar Features with Multilevel Mapping Representation, 2024 IEEE International Systems Conference (Montreal, QC, Canada), pp. 1-8.
[2]. Ok, M., Ok, S., & Park, J. H. (2021). Estimation of Vehicle Attitude, Acceleration, and Angular Velocity Using Convolutional Neural Network and Dual Extended Kalman Filter. Sensors (Basel, Switzerland), 21(4), 1282.
[3]. Rossi, Y., Tatsis, K., Awadaljeed, M., Arbogast, K., Chatzi, E., Rothacher, M., & Clinton, J. (2021). Kalman Filter-Based Fusion of Collocated Acceleration, GNSS and Rotation Data for 6C Motion Tracking. Sensors (Basel, Switzerland), 21(4), 1543.
[4]. Jiang, P., Hu, C., Wang, T., Lv, K., Guo, T., Jiang, J., Hu, W. (2024). Research on a Visual/Ultra-Wideband Tightly Coupled Fusion Localization Algorithm. Sensors, 24, 1710.
[5]. Vladimír, K. (2017). Rudolf E. Kalman: Life and Works. IFAC-PapersOnLine, 50(1), 631-636.
[6]. VilasBoas-Ribeiro, I., Nouwens, S. A. N., Curto, S., Jager, B., Franckena, M., Rhoon, G. C., Heemels, W. P. M. H., & Paulides, M. M. (2022). POD-Kalman filtering for improving noninvasive 3D temperature monitoring in MR-guided hyperthermia. Medical physics, 49(8).
[7]. Hu, L., Hu, R., Ma, Z., & Jiang, W. (2022). State of Charge Estimation and Evaluation of Lithium Battery Using Kalman Filter Algorithms. Materials (Basel, Switzerland), 15(24), 8744.
[8]. Lin, Q., Li, X., Tu, B., Cao, J., Zhang, M., & Xiang, J. (2023). Stable and Accurate Estimation of SOC Using eXogenous Kalman Filter for Lithium-Ion Batteries. Sensors (Basel, Switzerland), 23(1), 467.
[9]. Wang, J., Pan, Z., Li, B. and Wang, R. (2023). Terrain Estimation for Quadruped Robots Based on Kalman Filter, 2023 8th International Conference on Intelligent Computing and Signal Processing (Xi'an, China), pp. 747-754.
[10]. Santana, B., Cherif, E. K., Bernardino, A., & Ribeiro, R. (2022). Real-Time Georeferencing of Fire Front Aerial Images Using Iterative Ray-Tracing and the Bearings-Range Extended Kalman Filter. Sensors (Basel, Switzerland), 22(3).
[11]. Li, N., Gao, Y., Wang, Y., Liu, Z., Guan, L., & Liu, X. (2019). A Low-Cost Underground Garage Navigation Switching Algorithm Based on Kalman Filtering. Sensors (Basel, Switzerland), 19(8), 1861.
[12]. Eman, A., & Ramdane, H. (2020). Mobile Robot Localization Using Extended Kalman Filter. 2020 3rd International Conference on Computer Applications & Information Security (Riyadh, Saudi Arabia), pp. 1-5.
[13]. Hou, K., & Tang, Y. (2023). Visual Positioning System of Intelligent Robot Based on Improved Kalman Filter. 2023 China Automation Congress (Chongqing, China), pp. 5804-5808.