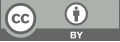
Advanced obstacle recognition and trajectory prediction for autonomous vehicles using 3D CNN and GRU
- 1 School of Information Technology, University of New South Wales, Sydney, Australia
* Author to whom correspondence should be addressed.
Abstract
Recent years have seen a rapid advancement in new energy vehicle technology, which has increased consumer demand for autonomous driving systems. However, current self-driving cars still face many technical barriers. Obstacle detection and obstacle trajectory prediction in dynamic environments is one of the main difficulties of the technique. To improve self-driving cars' capacity for route planning and decision-making in dynamic contexts, this thesis proposes an obstacle identification and trajectory prediction model that combines a gated recurrent unit (GRU) network with a three-dimensional convolutional neural network (3D CNN). The integrated framework utilises the feature recognition capability of 3D CNN and the prediction capability of GRU to accurately predict dynamic obstacles, thereby improving the performance of the model in various types of environments (e.g., visual SLAM). Experimental results based on publicly available datasets (e.g., KITTI and Argoverse) show that the proposed method has significant advantages in dynamic obstacle identification and prediction as well as overall model operation efficiency. The findings show that the integrated framework is useful and successful in advancing the creation of self-driving automobiles.
Keywords
Gated Recurrent Units, 3D Convolutional Neural Network, Obstacle recognition, Simultaneous Localization and Mapping.
[1]. Yao, J., Zhang, B., Li, C., Hong, D., & Chanussot, J. (2023). Extended vision transformer (ExViT) for land use and land cover classification: A multimodal deep learning framework. IEEE Transactions on Geoscience and Remote Sensing, 61, 1-15.
[2]. Taye, M. M. (2023). Theoretical understanding of convolutional neural network: Concepts, architectures, applications, future directions. Computation, 11(3), 52.
[3]. Salehi, A. W., Khan, S., Gupta, G., Alabduallah, B. I., Almjally, A., Alsolai, H., ... & Mellit, A. (2023). A study of CNN and transfer learning in medical imaging: Advantages, challenges, future scope. Sustainability, 15(7), 5930.
[4]. Shiri, F. M., Perumal, T., Mustapha, N., & Mohamed, R. (2023). A comprehensive overview and comparative analysis on deep learning models: CNN, RNN, LSTM, GRU. arXiv preprint arXiv:2305.17473.
[5]. Li, S. E. (2023). Deep reinforcement learning. In Reinforcement learning for sequential decision and optimal control (pp. 365-402). Singapore: Springer Nature Singapore.
[6]. Liu, Y., Lu, Y., Nayak, K., Zhang, F., Zhang, L., & Zhao, Y. (2022, November). Empirical analysis of eip-1559: Transaction fees, waiting times, and consensus security. In Proceedings of the 2022 ACM SIGSAC Conference on Computer and Communications Security (pp. 2099-2113).
[7]. Huang, K., Shi, B., Li, X., Li, X., Huang, S., & Li, Y. (2022). Multi-modal sensor fusion for auto driving perception: A survey. arXiv preprint arXiv:2202.02703.
[8]. Ebadi, K., Bernreiter, L., Biggie, H., Catt, G., Chang, Y., Chatterjee, A., ... & Carlone, L. (2023). Present and future of slam in extreme environments: The darpa subt challenge. IEEE Transactions on Robotics.
[9]. Elbaz, K., Shaban, W. M., Zhou, A., & Shen, S. L. (2023). Real time image-based air quality forecasts using a 3D-CNN approach with an attention mechanism. Chemosphere, 333, 138867.
[10]. Wang, P., Wang, P., Wang, C., Xue, B., & Wang, D. (2022). Using a 3D convolutional neural network and gated recurrent unit for tropical cyclone track forecasting. Atmospheric Research, 269, 106053.
[11]. Ma, X., Man, Q., Yang, X., Dong, P., Yang, Z., Wu, J., & Liu, C. (2023). Urban feature extraction within a complex urban area with an improved 3D-CNN using airborne hyperspectral data. Remote Sensing, 15(4), 992.
[12]. Tay, N. C., Tee, C., Ong, T. S., & Teh, P. S. (2019, November). Abnormal behavior recognition using CNN-LSTM with attention mechanism. In 2019 1st International Conference on Electrical, Control and Instrumentation Engineering (ICECIE) (pp. 1-5). IEEE.
[13]. Xiao, H., Wang, C., Li, Z., Wang, R., Bo, C., Sotelo, M. A., & Xu, Y. (2020). UB‐LSTM: a trajectory prediction method combined with vehicle behavior recognition. Journal of Advanced Transportation, 2020(1), 8859689.
[14]. Halder, R., & Chatterjee, R. (2020). CNN-BiLSTM model for violence detection in smart surveillance. SN Computer science, 1(4), 201.
Cite this article
Wang,Z. (2024). Advanced obstacle recognition and trajectory prediction for autonomous vehicles using 3D CNN and GRU. Applied and Computational Engineering,101,164-174.
Data availability
The datasets used and/or analyzed during the current study will be available from the authors upon reasonable request.
Disclaimer/Publisher's Note
The statements, opinions and data contained in all publications are solely those of the individual author(s) and contributor(s) and not of EWA Publishing and/or the editor(s). EWA Publishing and/or the editor(s) disclaim responsibility for any injury to people or property resulting from any ideas, methods, instructions or products referred to in the content.
About volume
Volume title: Proceedings of the 2nd International Conference on Machine Learning and Automation
© 2024 by the author(s). Licensee EWA Publishing, Oxford, UK. This article is an open access article distributed under the terms and
conditions of the Creative Commons Attribution (CC BY) license. Authors who
publish this series agree to the following terms:
1. Authors retain copyright and grant the series right of first publication with the work simultaneously licensed under a Creative Commons
Attribution License that allows others to share the work with an acknowledgment of the work's authorship and initial publication in this
series.
2. Authors are able to enter into separate, additional contractual arrangements for the non-exclusive distribution of the series's published
version of the work (e.g., post it to an institutional repository or publish it in a book), with an acknowledgment of its initial
publication in this series.
3. Authors are permitted and encouraged to post their work online (e.g., in institutional repositories or on their website) prior to and
during the submission process, as it can lead to productive exchanges, as well as earlier and greater citation of published work (See
Open access policy for details).