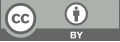
Exploiting EEG to identify confused students in online learning: A machine learning model comparison
- 1 School of Bioengineering, Chongqing University, Chongqing, 400044, China
* Author to whom correspondence should be addressed.
Abstract
The COVID-19 pandemic has forced most schools, including universities, to adopt online courses for teaching. Due to the breaking of the constraints of time and space, online courses still occupy a large proportion in education until now, and large-scale open online education courses will become a major trend in the future. Of course, problems also arise in this process. As teachers cannot receive feedback from students anytime and anywhere in online courses, such as micro expressions, body movements, etc., they cannot know whether students are confused. Therefore, how to effectively detect students' learning status has grown in popularity. In the last few years, machine learning has developed rapidly, and a large number of artificial intelligence have emerged, making it possible to detect whether students are confused by combining electroencephalography with machine learning. In order to determine whether students were confused, this study used a wireless single channel Mindset device to collect Electroencephalography (EEG) signals. Six machine learning models—random forests, eXtreme Gradient boosting, K-nearest neighbors, gradient boosting machines, logistic regression, and support vector machines—were then chosen. The findings indicate that other machine learning models have a high accuracy rate in classifying students' bewilderment, with the exception of the logistic regression model. With a 99.69% accuracy rate, the eXtreme gradient boosting model performs better than a number of other models.
Keywords
Machine Learning, Electroencephalography, Artificial Intelligence.
[1]. Shah, S., & Arinze, B. (2023). Comparing student learning in face-to-face versus online sections of an information technology course. IEEE Transactions on Professional Communication, 66(1), 48-58.
[2]. He, S., Xu, Y., & Zhong, L. (2021). EEG-based confusion recognition using different machine learning methods. International Conference on Artificial Intelligence and Computer Engineering, 826-831.
[3]. Ibrahim, S., Djemal, R., & Alsuwailem, A. (2018). Electroencephalography (EEG) signal processing for epilepsy and autism spectrum disorder diagnosis. Biocybernetics and Biomedical Engineering, 38(1), 16-26.
[4]. Janiesch, C., Zschech, P., & Heinrich, K. (2021). Machine learning and deep learning. Electronic Markets, 31(3), 685-695.
[5]. Han, Y., Ma, Y., Zhu, L., Zhang, Y., et, al. (2018). Study on mind controlled robotic arms by collecting and analyzing brain alpha waves. International Conference on Applied Mathematics, Modelling and Statistics Application, 145-148.
[6]. Contreras-Luján, E. E., García-Guerrero, E. E., López-Bonilla, O. R., Tlelo-Cuautle, E., López-Mancilla, D., & Inzunza-González, E. (2022). Evaluation of machine learning algorithms for early diagnosis of deep venous thrombosis. Mathematical and Computational Applications, 27(2), 24.
[7]. Ramírez-Arias, F. J., García-Guerrero, E. E., Tlelo-Cuautle, E., et, al. (2022). Evaluation of machine learning algorithms for classification of EEG signals. Technologies, 10(4), 79.
[8]. Jaiswal, A. K., & Banka, H. (2018). Epileptic seizure detection in EEG signal using machine learning techniques. Australasian physical & engineering sciences in medicine, 41, 81-94.
[9]. He, Y. (2024). Classification of confusion states of students viewing online classes using machine learning models. International Conference on Computer Vision, Image and Deep Learning, 651-664.
[10]. Haohan, W. (2018). Confused student EEG brainwave data. URL: https://www.kaggle.com/datasets/wanghaohan/confused-eeg. Last Accessed: 2024/08/12.
[11]. Lv, C. X., An, S. Y., Qiao, B. J., & Wu, W. (2021). Time series analysis of hemorrhagic fever with renal syndrome in mainland China by using an XGBoost forecasting model. BMC infectious diseases, 21, 1-13.
[12]. Breiman, L. (2001). Random forests. Machine learning, 45, 5-32.
[13]. Natekin, A., & Knoll, A. (2013). Gradient boosting machines, a tutorial. Frontiers in neurorobotics, 7, 21.
[14]. Zhang, S., Cheng, D., Deng, Z., Zong, M., & Deng, X. (2018). A novel kNN algorithm with data-driven k parameter computation. Pattern Recognition Letters, 109, 44-54.
[15]. Nick, T. G., & Campbell, K. M. (2007). Logistic regression. Topics in biostatistics, 273-301.
[16]. Suthaharan, S., & Suthaharan, S. (2016). Support vector machine. Machine learning models and algorithms for big data classification: thinking with examples for effective learning, 207-235.
[17]. Vafeiadis, T., Diamantaras, K. I., Sarigiannidis, G., & Chatzisavvas, K. C. (2015). A comparison of machine learning techniques for customer churn prediction. Simulation Modelling Practice and Theory, 55, 1-9.
Cite this article
Chen,J. (2024). Exploiting EEG to identify confused students in online learning: A machine learning model comparison. Applied and Computational Engineering,81,63-70.
Data availability
The datasets used and/or analyzed during the current study will be available from the authors upon reasonable request.
Disclaimer/Publisher's Note
The statements, opinions and data contained in all publications are solely those of the individual author(s) and contributor(s) and not of EWA Publishing and/or the editor(s). EWA Publishing and/or the editor(s) disclaim responsibility for any injury to people or property resulting from any ideas, methods, instructions or products referred to in the content.
About volume
Volume title: Proceedings of the 2nd International Conference on Machine Learning and Automation
© 2024 by the author(s). Licensee EWA Publishing, Oxford, UK. This article is an open access article distributed under the terms and
conditions of the Creative Commons Attribution (CC BY) license. Authors who
publish this series agree to the following terms:
1. Authors retain copyright and grant the series right of first publication with the work simultaneously licensed under a Creative Commons
Attribution License that allows others to share the work with an acknowledgment of the work's authorship and initial publication in this
series.
2. Authors are able to enter into separate, additional contractual arrangements for the non-exclusive distribution of the series's published
version of the work (e.g., post it to an institutional repository or publish it in a book), with an acknowledgment of its initial
publication in this series.
3. Authors are permitted and encouraged to post their work online (e.g., in institutional repositories or on their website) prior to and
during the submission process, as it can lead to productive exchanges, as well as earlier and greater citation of published work (See
Open access policy for details).