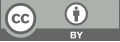
AI-driven automatic generation and rendering of game characters
- 1 Faculty of Science, Western University, ON, Canada
* Author to whom correspondence should be addressed.
Abstract
This paper provides a comprehensive review of AI-driven techniques for the automatic generation and rendering of game characters, with a particular focus on Generative Adversarial Networks (GANs) and Variational Autoencoders (VAEs). GANs, using adversarial training approaches, have revolutionized character development by producing incredibly realistic and aesthetically pleasing gaming characters. VAEs, despite frequently encountering issues like image blurriness, provide an alternate strategy that emphasizes diversity and originality in the generated content. Additionally, conditional models that enable more individualized and regulated character generation are explored, as well as hybrid models that combine the best features of both GANs and VAEs. The difficulties with mode collapse in GANs and the requirement for big datasets for both GANs and VAEs are also covered, along with some possible fixes like transfer learning and semi-supervised learning strategies. This analysis emphasizes the growing significance of AI-driven game character generation in the gaming industry by highlighting its current state, problems, and future directions.
Keywords
Game, generative adversarial networks, variational autoencoders.
[1]. Goodfellow I, Pouget-Abadie J, Mirza M, Xu B, Warde-Farley D, Sherjil O, Aaron C and Yoshua B Generative adversarial nets 2014 Adv. Neural Inf. Process. Syst. 27 2672-80
[2]. Shi T, Zuo Z, Yuan Y and Fan C Fast and robust face-to-parameter translation for game character auto-creation 2020 Proc. AAAI Conf. Artif. Intell. 34(02) 1733-1740
[3]. Emekligil F G A and Öksüz İ Game character generation with generative adversarial networks 2022 In: 30th Signal Processing and Communications Applications Conference (SIU) 1-4
[4]. Radford A, Metz L and Chintala S Unsupervised representation learning with deep convolutional generative adversarial networks 2016 arXiv:1511.06434
[5]. Gulrajani I, Ahmed F, Arjovsky M, Dumoulin V and Courville A C Improved training of Wasserstein GANs 2017 Adv. Neural Inf. Process. Syst. 30 5769-79
[6]. Miyato T, Kataoka T, Koyama M and Yoshida Y Spectral normalization for generative adversarial networks 2018 arXiv:1802.05957
[7]. Brock A, Donahue J and Simonyan K Large scale GAN training for high fidelity natural image synthesis 2019 arXiv:1809.11096
[8]. Shi T, Yuan Y, Fan C, Zou Z, Shi Z and Liu Y Face-to-parameter translation for game character auto-creation 2019 In: IEEE/CVF International Conference on Computer Vision (ICCV) 161-170
[9]. Zhao R, Li W, Hu Z, Li L, Zou Z, Shi Z and Fan C Zero-shot text-to-parameter translation for game character auto-creation 2023 In: IEEE/CVF Conference on Computer Vision and Pattern Recognition (CVPR) 21013-21023
[10]. Wang S, Zeng W, Wang X, Yang H, Chen L, Yuan Y, Zeng Y, Zheng M, Zhang C and Wu M SwiftAvatar: Efficient auto-creation of parameterized stylized character on arbitrary avatar engines 2023 arXiv:2301.08153
[11]. Emekligil F G A Generative models for game character generation 2023
Cite this article
Xie,Y. (2024). AI-driven automatic generation and rendering of game characters. Applied and Computational Engineering,82,137-141.
Data availability
The datasets used and/or analyzed during the current study will be available from the authors upon reasonable request.
Disclaimer/Publisher's Note
The statements, opinions and data contained in all publications are solely those of the individual author(s) and contributor(s) and not of EWA Publishing and/or the editor(s). EWA Publishing and/or the editor(s) disclaim responsibility for any injury to people or property resulting from any ideas, methods, instructions or products referred to in the content.
About volume
Volume title: Proceedings of the 2nd International Conference on Machine Learning and Automation
© 2024 by the author(s). Licensee EWA Publishing, Oxford, UK. This article is an open access article distributed under the terms and
conditions of the Creative Commons Attribution (CC BY) license. Authors who
publish this series agree to the following terms:
1. Authors retain copyright and grant the series right of first publication with the work simultaneously licensed under a Creative Commons
Attribution License that allows others to share the work with an acknowledgment of the work's authorship and initial publication in this
series.
2. Authors are able to enter into separate, additional contractual arrangements for the non-exclusive distribution of the series's published
version of the work (e.g., post it to an institutional repository or publish it in a book), with an acknowledgment of its initial
publication in this series.
3. Authors are permitted and encouraged to post their work online (e.g., in institutional repositories or on their website) prior to and
during the submission process, as it can lead to productive exchanges, as well as earlier and greater citation of published work (See
Open access policy for details).