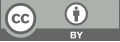
Design of automatic sorting system based on capsule neural network
- 1 School of Information Science and Technology, East China University of Science and Technology, Shanghai, China
* Author to whom correspondence should be addressed.
Abstract
This paper proposes a vegetable disease classification system based on Capsule Neural Network (CapsNet), which uses the visual recognition mechanism of deep learning algorithms to improve the efficiency and accuracy of agricultural disease recognition. The CapsNet algorithm outperforms traditional Convolutional Neural Network (CNN) in few-shot learning and generalization due to its unique capsule structure and dynamic routing mechanism. Experimental results show that the proposed system performs well in the accuracy, robustness, and model interpretation of image recognition, and can accurately identify diseases even in the case of poor image quality or noise and occlusion. In addition, the embedded system of this experiment includes an image acquisition module, an algorithm core module, and a logic control module, which realizes the automation of the whole process from image acquisition to recognition result display. This study also discusses the scalability and adaptability of the system, as well as the future application prospects in agricultural intelligence and automation. It is expected that CapsNet will play a more critical role in artificial intelligence, especially in agrarian automation and smart agriculture.
Keywords
capsule neural network, embedded system, visual recognition, smart agriculture.
[1]. Hanyu Zhao, Hongyan Chai. Strengthen agricultural mechanization technology innovation Promoting agricultural modernization process [J]. Journal of Jiangsu Agricultural Mechanization, 2012, (4): 14-16. DOI: 10.16271 / j.carol carroll nki JSNJH. 2012.04.011.
[2]. Liu Fo, LI Weisheng. Application of embedded system in Shuguang 4000A cluster Monitoring [J]. Microcomputer Information, 2005, (05): 57-58.
[3]. Kranti K, Joydeep S . A comprehensive survey on emotion recognition based on electroencephalograph (EEG) signals [J]. Multimedia Tools and Applications, 2023, 82 (18): 27269-27304.
[4]. Li Guanghui. Research on Embedded Software Related problems [J]. Information Technology, 2007, (02): 67-68.
[5]. Ran C, Hao S, Zhong-Qiu Z, et al. Global routing between capsules [J]. Pattern Recognition, 2024, 148
[6]. Bao Xiaomin, WANG Jingbo. IoT technology in agricultural pests telemetry applications and development [J]. Journal of Xinjiang Agricultural Mechanization, 2020, (5): 25-29. DOI: 10.13620 / j.carol carroll nki issn1007-7782.2020.05.007.
[7]. Wu Lin, SUN Jingyu. Multi-branch RA capsule Network and its application in image classification [J]. Computer Science, 2022, 49 (06): 224-230.
[8]. Qiao Yu-si, Qiao Lian-Jun. Analysis of the Development Status and Industry Prospect of Embedded System [J]. Statistics and Management, 2013, (03): 89-90.
[9]. Duan Gui-duo, Luo Guang-chun, Zhang Li-Zong. zhu Dayong, Wang Zi-peng. Fundus Retinal image classification method Based on capsule Network [P]. China: CN201811197008.6.
[10]. Chen Jian, ZHOU Ping. Chinese Handwriting Identification Algorithm Based on Capsule Network [J]. Journal of Packaging, 2018, 10 (05): 51-56.
[11]. Feng Fenling, YAN Meihao, LIU Chengguang, et al. Export demand forecasting of China-Europe Train based on IPSO-Capsule-NN model [J]. China Railway Science, 2020, 41 (02): 147-156.
[12]. Sheng Guanqun, FANG Hao. Method and system for picking up microseismic P-wave based on capsule neural network [P]. China :CN202010057384.6.
[13]. Hu Chuntao, Xia Lingling, Zhang Liang, et al. Comparison of Text Classification Based on Capsule Network and Convolutional Network [J]. Computer Technology and Development, 2020, 30 (10): 86-91.
[14]. Huang Xiaogang, Huang Runcai, WANG Guijiang, et al. Facial expression Recognition Based on Feature Extraction and Capsule Network [J]. Intelligent Computer & Application, 2022, 12 (10): 68-74.
[15]. Geng Shaoguang. Design and Research of intelligent Car based on embedded device and deep learning Model [J]. Digital Technology and Application, 2020, 38 (05): 161-162. DOI:10.19695/j.cnki.cn12-1369.2020.05.90.
[16]. Application analysis of agricultural machinery automation and agricultural intelligence [J]. Agricultural Engineering Technology, 2023, 43 (29): 31-32. (in Chinese) DOI:10.16815/j.cnki.11-5436/s.2023.29.011.
Cite this article
Ju,Y. (2024). Design of automatic sorting system based on capsule neural network. Applied and Computational Engineering,101,198-206.
Data availability
The datasets used and/or analyzed during the current study will be available from the authors upon reasonable request.
Disclaimer/Publisher's Note
The statements, opinions and data contained in all publications are solely those of the individual author(s) and contributor(s) and not of EWA Publishing and/or the editor(s). EWA Publishing and/or the editor(s) disclaim responsibility for any injury to people or property resulting from any ideas, methods, instructions or products referred to in the content.
About volume
Volume title: Proceedings of the 2nd International Conference on Machine Learning and Automation
© 2024 by the author(s). Licensee EWA Publishing, Oxford, UK. This article is an open access article distributed under the terms and
conditions of the Creative Commons Attribution (CC BY) license. Authors who
publish this series agree to the following terms:
1. Authors retain copyright and grant the series right of first publication with the work simultaneously licensed under a Creative Commons
Attribution License that allows others to share the work with an acknowledgment of the work's authorship and initial publication in this
series.
2. Authors are able to enter into separate, additional contractual arrangements for the non-exclusive distribution of the series's published
version of the work (e.g., post it to an institutional repository or publish it in a book), with an acknowledgment of its initial
publication in this series.
3. Authors are permitted and encouraged to post their work online (e.g., in institutional repositories or on their website) prior to and
during the submission process, as it can lead to productive exchanges, as well as earlier and greater citation of published work (See
Open access policy for details).