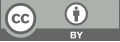
The Latest Development of Synthetic Aperture Radar: An Overview
- 1 Wuhan University, 299 Bayi Road, Wuchang District, Wuhan, Hubei, China
* Author to whom correspondence should be addressed.
Abstract
Synthetic Aperture Radar (SAR) technology enables high-resolution imaging regardless of weather conditions, offering immense potential for target detection and recognition. This paper reviews the latest advancements in SAR technology, highlighting key developments and applications. The limitations of traditional SAR imaging techniques, particularly the trade-off between resolution and swath width, have been addressed by High Resolution Wide Swath (HRWS) SAR, enabling simultaneous acquisition of high-resolution and wide-swath data. Multi-polarimetric SAR provides richer scattering information for improved target recognition accuracy, while deep learning algorithms enhance image quality and recognition performance. Emerging technologies like 3D-ISAR and holographic imaging offer novel approaches to target recognition and tracking. We discuss the development history, performance advantages, and limitations of these technologies, providing a comprehensive overview of their practical applications. We identify current challenges and future trends in SAR target imaging and recognition, including computational efficiency, adversarial attack defense, data acquisition and processing, and feature extraction and classification. Potential research directions, such as integrated multi-mode SAR systems, multi-source data fusion, and adversarial attack defense strategies, are outlined to fully harness the potential of SAR technology in target imaging and recognition.
Keywords
Synthetic Aperture Radar, Imaging, Object Detection.
[1]. Zuzana Hajkova What is SAR Imagery? Introduction to Synthetic Aperture Radar[OL], https://www.euspaceimaging.com/blog/2024/04/05/what-is-sar-imagery/, 5 April, 2024
[2]. Sommervold O, Gazzea M, Arghandeh R. A survey on SAR and optical satellite image registration[J]. Remote Sensing, 2023, 15(3): 850.
[3]. Li J, Yu Z, Yu L, et al. A comprehensive survey on SAR ATR in deep-learning era[J]. Remote Sensing, 2023, 15(5): 1454.
[4]. Passah A, Sur S N, Abraham A, et al. Synthetic Aperture Radar image analysis based on deep learning: A review of a decade of research[J]. Engineering Applications of Artificial Intelligence, 2023, 123: 106305.
[5]. Jiang W, Han H, Zhang Y, et al. Federated split learning for sequential data in satellite–terrestrial integrated networks[J]. Information Fusion, 2024, 103: 102141.
[6]. Yang B, Wang X, Xing Y, et al. Modality Fusion Vision Transformer for Hyperspectral and LiDAR Data Collaborative Classification[J]. IEEE Journal of Selected Topics in Applied Earth Observations and Remote Sensing, 2024.
[7]. Jiang W, Zhan Y, Xiao X. Multi-domain network slicing in satellite–terrestrial integrated networks: A multi-sided ascending-price auction approach[J]. Aerospace, 2023, 10(10): 830.
[8]. Jianping W, Guangqiu Q, Chunming W, et al. Federated learning for network attack detection using attention-based graph neural networks[J]. Scientific Reports, 2024, 14(1): 19088.
[9]. Jiang W. Cellular traffic prediction with machine learning: A survey[J]. Expert Systems with Applications, 2022, 201: 117163.
[10]. Jiang W. Graph-based deep learning for communication networks: A survey[J]. Computer Communications, 2022, 185: 40-54.
[11]. Meng X, Zhu D, Dong L. Highly Squinted Airborne SAR Imaging Based on Multi-Core DSP System[C]//2021 IEEE 6th International Conference on Signal and Image Processing (ICSIP). IEEE, 2021: 361-365.
[12]. Yonel B, Choudhury N, Yazici B. Phaseless Multi-Static Synthetic Aperture Radar Imaging[J]. IEEE Journal of Selected Topics in Applied Earth Observations and Remote Sensing, 2024.
[13]. Villano M, Peixoto M N, Kim S, et al. Potential of Multi-Static SAR Systems for Earth Monitoring and Their Demonstration Using Swarms of Drones[C]//IGARSS 2023-2023 IEEE International Geoscience and Remote Sensing Symposium. IEEE, 2023: 4586-4589.
[14]. Tian H, Li D. Sparse flight array SAR downward-looking 3-D imaging based on compressed sensing[J]. IEEE Geoscience and Remote Sensing Letters, 2016, 13(10): 1395-1399.
[15]. Pui C Y, Ng B, Rosenberg L, et al. Target classification for 3D-ISAR using CNNs[J]. IEEE Transactions on Aerospace and Electronic Systems, 2023, 60(1): 94-105.
[16]. Ren H, Zhang Y, Li G, et al. The Characteristic Analysis of Spatial Variance of MEO/Missile-Borne Bistatic SAR[C]//IGARSS 2023-2023 IEEE International Geoscience and Remote Sensing Symposium. IEEE, 2023: 8142-8145.
[17]. Brunner D, Lemoine G, Bruzzone L, et al. Building height retrieval from VHR SAR imagery based on an iterative simulation and matching technique[J]. IEEE Transactions on Geoscience and Remote Sensing, 2009, 48(3): 1487-1504.
[18]. Fornaro G, Reale D, Serafino F. Four-dimensional SAR imaging for height estimation and monitoring of single and double scatterers[J]. IEEE Transactions on Geoscience and Remote Sensing, 2008, 47(1): 224-237.
[19]. Liu L, Chen G, Pan Z, et al. Inshore ship detection in SAR images based on deep neural networks[C]//IGARSS 2018-2018 IEEE International Geoscience and Remote Sensing Symposium. IEEE, 2018: 25-28.
[20]. Wong S K. High range resolution profiles as motion-invariant features for moving ground targets identification in SAR-based automatic target recognition[J]. IEEE Transactions on Aerospace and Electronic Systems, 2009, 45(3): 1017-1039.
[21]. Xuanshen WAN, Wei LIU, Chaoyang NIU, et al. Black-box Attack Algorithm for SAR-ATR Deep Neural Networks Based on MI-FGSM[J]. Journal of Radars, 2024, 13: 1-16.
[22]. He M, Jiang W, Gu W. TriChronoNet: Advancing electricity price prediction with Multi-module fusion[J]. Applied Energy, 2024, 371: 123626.
[23]. Jiang W, Zhang Y, Han H, et al. Mobile traffic prediction in consumer applications: a multimodal deep learning approach[J]. IEEE Transactions on Consumer Electronics, 2024.
Cite this article
Guo,J. (2024). The Latest Development of Synthetic Aperture Radar: An Overview. Applied and Computational Engineering,112,186-193.
Data availability
The datasets used and/or analyzed during the current study will be available from the authors upon reasonable request.
Disclaimer/Publisher's Note
The statements, opinions and data contained in all publications are solely those of the individual author(s) and contributor(s) and not of EWA Publishing and/or the editor(s). EWA Publishing and/or the editor(s) disclaim responsibility for any injury to people or property resulting from any ideas, methods, instructions or products referred to in the content.
About volume
Volume title: Proceedings of the 5th International Conference on Signal Processing and Machine Learning
© 2024 by the author(s). Licensee EWA Publishing, Oxford, UK. This article is an open access article distributed under the terms and
conditions of the Creative Commons Attribution (CC BY) license. Authors who
publish this series agree to the following terms:
1. Authors retain copyright and grant the series right of first publication with the work simultaneously licensed under a Creative Commons
Attribution License that allows others to share the work with an acknowledgment of the work's authorship and initial publication in this
series.
2. Authors are able to enter into separate, additional contractual arrangements for the non-exclusive distribution of the series's published
version of the work (e.g., post it to an institutional repository or publish it in a book), with an acknowledgment of its initial
publication in this series.
3. Authors are permitted and encouraged to post their work online (e.g., in institutional repositories or on their website) prior to and
during the submission process, as it can lead to productive exchanges, as well as earlier and greater citation of published work (See
Open access policy for details).