1. Introduction
In modern urban architecture, glass curtain walls are commonly used for high-rise building façades due to their excellent light transmission and contemporary appearance, which significantly contribute to the building’s aesthetic and functional qualities. However, over time, these walls accumulate dust, rain stains, and other dirt, adversely affecting their visual appeal and transparency. Therefore, regular cleaning is essential to preserve both the cleanliness and clarity of the glass curtain walls. Traditional cleaning methods, typically involving manual operations and “spider-man” style hanging techniques, are labor-intensive and inefficient, with significant safety risks at high altitudes. To enhance both efficiency and safety, automated cleaning technologies, especially high-rise glass curtain wall cleaning robots, have become a focus of research. Despite progress in high-rise glass curtain wall cleaning robot technology, several technical challenges persist, including optimization of adhesion technologies (magnetic, thrust, vacuum negative pressure, and bionic), mobility modes (legged, wheeled, tracked, and externally assisted), cleaning methods (physical and chemical), and path planning techniques (regular scanning, random walk, dynamic planning, and bionic planning). Thus, this paper provides a full review of current technologies for high-rise glass curtain wall cleaning robots, analyzing their applications, advantages, limitations, and challenges, and proposing optimization strategies. The aim is to offer valuable insights for advancing research and development in this field and improving practical applications.
2. Analysis of Existing Wall-Climbing Robot Technologies
2.1. Adsorption Techniques
Adsorption methods include magnetic adsorption, thrust adsorption, vacuum adsorption and bionic adsorption. Magnetic adsorption uses a magnetic field to attach the wall-climbing robot to the surface, and is divided into electromagnetic adsorption and permanent magnet adsorption. Electromagnetic adsorption achieves adhesion through the magnetic field generated by electric current, which is suitable for ferromagnetic surfaces and provides strong adhesion, but requires an external power supply, which limits its use during movement. Permanent magnet adsorption uses permanent magnets to generate a constant magnetic field, which can continue to operate during power outages, but is only effective for ferromagnetic materials, with limited application to non-ferromagnetic surfaces. Recently, researchers have explored hybrid models that combine electromagnetic and permanent magnet adsorption to address their respective shortcomings. Thrust adsorption methods use pneumatic or mechanical devices to generate thrust for attachment to walls. Pneumatic spiral thrust generates thrust through rotating pneumatic devices to stabilize the robot on the wall, suitable for scenarios requiring strong thrust but with high energy consumption and complex control. For example, the TITO500 robot performs well on high-rise windows using this method. [1]. Another thrust adsorption method involves using propellers, applied in some small wall-climbing robots, which provides simple control but still faces issues with efficiency and stability. Vacuum negative pressure adsorption utilizes the negative pressure generated between the robot and the surface for attachment, widely used on smooth surfaces like glass and tiles, providing strong adhesion. For instance, Ecovacs’ Window Treasure robot uses this technology, working effectively at up to 3000Pa of negative pressure, ensuring stability on vertical glass surfaces. However, this method performs poorly on rough or uneven surfaces and requires high equipment sealing. Bionic adsorption technology mimics the adsorption mechanisms of natural organisms, particularly the foot structure of geckos. Through the use of bionic materials or suction cups, it is possible to mimic biological adhesion mechanisms and attach to a variety of surfaces. This technique offers great flexibility by adapting to different surface conditions, including rough surfaces, but faces challenges in improving adhesion strength and maintaining long-term stability [2]. For instance, several wall-climbing robots have included biologically inspired design principles; yet, issues with cost and complexity still exist. [3].
2.2. Mobile Modes
Wall-climbing robots employ several forms of movement, each possessing distinct benefits and constraints appropriate for various environment conditions [4]. Legged wall-climbing robots imitate the leg mechanisms of animals, offering exceptional flexibility and the ability to navigate obstacles. These robots usually feature a multi-legged configuration to ensure steady motion in intricate surroundings. For instance, the Spot from Boston Dynamics exhibits outstanding performance over uneven surfaces. Nevertheless, legged designs frequently entail greater structural intricacy and challenges in control, while their pace of movement is comparatively sluggish. The outrigger design is being streamlined in related research to enhance efficiency and tackle conditions in high-altitude applications. Wheeled wall-climbing robots deploy wheels to facilitate locomotion, making them well-suited for level surfaces. This architecture enables accelerated locomotion and streamlined control systems, exemplified as the iRobot Looj series. Although wheeled robots operate with precision on level ground, their flexibility is restricted when confronted with uneven surfaces or impediments. Notwithstanding this, the straightforwardness of wheeled designs nevertheless enables them to compete in some application situations. Tracked robots employ a rigid track system to augment their stability and ability to bear loads. For instance, the TARF robot utilizes a tracked architecture to navigate smoothly over intricate landscapes, providing excellent capability to surmount obstacles. Nevertheless, this particular design brings about a greater level of structural intricacy, therefore augmenting the challenges associated with operation and maintenance. The primary objective of researchers is to enhance the adaptability and cost-effectiveness of tracked systems by simplified their design. Externally-assisted wall-climbing robots depend on external mechanisms, such as airborne baskets or track systems, to facilitate their locomotion. For example, the ispider robot developed by Xiamen Pufeng Technology employs a cable system to ensure steady operation, rendering it well-suited for conducting extensive cleaning operations at high altitudes. Although this design achieves exceptional performance in intricate settings, it necessitates supplementary installation equipment and encounters constraints in commercial applications. The present study is primarily concerned with enhancing system adaptability and minimising installation expenses.
2.3. Cleaning Methods
Methods of cleaning can be categorized into physical and chemical procedures, each possessing distinct advantages and constraints, appropriate for certain cleaning situations. Mechanical cleaning and high-pressure water spraying are examples of physical techniques [5]. Mechanical cleaning involves the use of implements such as brushes, scrapers, and sponges to physically remove dirt and grime. This method is typically efficient for regular cleaning applications, effortlessly managing common dirt. Nevertheless, the effectiveness of this approach decreases when confronted with persistent or deeply embedded stains, as alone physical techniques may not offer adequate strength or coverage. To overcome this constraint, high-pressure water spraying utilizes strong streams of water to forcefully remove more enduring dirt and debris. This approach is very advantageous for doing extensive cleaning operations and is capable of managing substantial soiling. However, the operation necessitates a sufficient water supply and an effective drainage system, and there is a potential for substantial water loss during the procedure. Chemical techniques in cleaning include the application of specific cleaning solutions that chemically react with and degrade stains and dirt. These agents are specifically formulated to selectively remove particular forms of grime, such as water stains, bird droppings, and other persistent residues, therefore prolonging the overall cleaning efficacy. The co-application of chemical agents with mechanical cleaning methods frequently produces exceptional outcomes. As an illustration, contemporary wall-climbing robots, like Cobos' "Window Treasure," combine chemical cleansers with physical brushes or sponges to efficiently clean walls. The use of chemicals, however, necessitates meticulous evaluation of their possible environmental consequences and suitability for the surfaces being cleaned. The excessive application or improper use of chemicals might result in surface deterioration or negative environmental consequences.
2.4. Path Planning Method
In the domain of path planning, a variety of methods have been developed that exhibit distinctive strengths and are subject to particular constraints, thereby rendering them applicable to disparate scenarios. The rule-based scanning method employs predefined patterns, such as straight lines or grids, and is particularly effective in predictable environments with regular surfaces. This is due to the simplicity and efficiency of the method. However, its efficacy is diminished in complex or irregular settings due to its lack of adaptability to dynamic changes. Researchers are endeavoring to enhance its flexibility by integrating environmental sensing for dynamic rule adjustments. The random walk method enables robots to explore paths randomly, which is advantageous in unknown or complex environments due to its adaptability. Nevertheless, this approach may result in reduced efficiency and the inefficient use of resources, such as the traversal of redundant or missed areas. Attempts are being made to enhance the efficiency and reduce the wastage of resources through the optimization of algorithms. The sensor feedback-based dynamic planning method enables real-time adjustments to paths based on environmental sensing, thereby facilitating high adaptability to complex and changing conditions. However, this approach requires a sophisticated computational system, which adds to the complexity and cost. The objective of the research is to enhance the real-time performance and accuracy of the method while managing the computational demands. The bionic path planning method employs a combination of biological navigation strategies, such as those observed in ant foraging, and artificial intelligence to optimize paths [6].
3. Technical Issues and Challenges
3.1. Limitations of Adhesion Technology
Adhesion technology has several constraints when used in the implementation of wall-climbing robots. The adaptation to various surfaces is a notable obstacle. Despite its strong performance on smooth surfaces such as glass, magnetic adhesion and vacuum suction often encounter difficulties when used to rough or uneven surfaces. Specifically, magnetic adhesion is not successful on non-ferromagnetic materials, and vacuum suction may not be able to generate sufficient negative pressure on uneven surfaces. Furthermore, the constraint of adhesive strength is a crucial concern. The adhesive force is affected by several parameters, such as surface roughness, material composition, and ambient conditions. Insufficient adhesion strength might result in the robot disengaging from the surface while in motion, therefore impacting operational stability and safety [7].
3.2. Suitability of Mobility Modes
Wall-climbing robots are characterized by distinct advantages and disadvantages associated with various mobility modes. Although legged robots have great flexibility and obstacle-crossing skills, their structural complexity and slower movement speed serve as limitations that hinder their use in efficient operations. Wheeled robots execute quick movements on level terrain but exhibit suboptimal performance in uneven or blocked surroundings. Track-based robots offer superior stability and load capacity, but their intricate design and technical maintenance difficulties impede their performance. Externally aided robots, which depend on supplementary equipment support, are well-suited for extensive high-altitude operations. However, their ability to be widely commercially adopted is limited due to the significant expenses associated with their installation and operation. The selection and usage strategies of these different modes are determined by their applicability and functional limits in particular application settings [8].
3.3. Cleaning Efficiency and Effectiveness
Enhancing cleaning efficiency and effectiveness requires finding an optimal balance between physical and chemical methods and optimizing them. Existing cleaning methods face issues with efficiency and effectiveness. Physical cleaning methods, while straightforward, are less efficient against stubborn dirt and require a great deal of time and manpower. High-pressure water jetting improves efficiency for large-scale cleaning but demands high water source and drainage system requirements and may lead to water resource wastage. Chemical cleaning methods enhance cleaning effectiveness through the use of detergents, but their efficacy is influenced by detergent type, concentration, and dirt nature. Besides, environmental impact and potential damage to equipment from detergents must be considered [9].
3.4. Complexity of Path Planning
The problem of path planning in wall-climbing robot technology is of great complexity, particularly in terms of adaptation in intricate situations. Although simple, rule-based scanning approaches may not adequately cover all areas in complicated situations. Random walk algorithms, although providing a certain degree of flexibility, can be affected by path uncertainty, resulting in decreased efficiency. Sensor feedback-based dynamic planning approaches can actively modify routes in real-time to adapt to environmental changes. However, these methods need significant computational and reaction capabilities, which leads to an increase in system complexity. Despite their tremendous adaptability, biomimetic path planning approaches need intricate implementations and the integration of biological and artificial intelligence algorithms. To improve the adaptability and accuracy of route planning, algorithms and system structures need to be optimized for practical applications [9].
4. Optimization Strategies and Recommendations
4.1. Structural Design and Function Integration Optimization
Optimizing the compactness and mobility of the structural framework is essential for high-rise glass curtain wall cleaning robots. An optimal approach is to employ a three-segment I-frame structure, as depicted in Figure 1, which not only guarantees the robot's rigidity but also enhances its flexibility and stability during operation. The implementation of this design facilitates improved load distribution and amplifies the robot's capacity to navigate through diverse surface conditions. Moreover, the integration of a self-cleaning mechanism, such as a modular plate brush, into the upper part of the cleaning plate, can greatly enhance cleaning effectiveness and decrease the need for frequent maintenance. The inbuilt self-cleaning function guarantees the continued effectiveness of the cleaning surface, even in settings characterized by significant dirt buildup. For further optimization of the structure, it is crucial to integrate and design different functional modules in a cohesive manner, therefore enhancing the overall coordination and operational reliability of the system. Furthermore, this integration will contribute to the reduction of assembly complexity and the optimization of operational procedures.
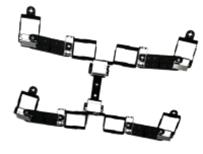
Figure 1. 3D Model of Torso
4.2. Prototype Modeling and Motion Simulation Optimization
It is advisable to use SolidWorks for the creation of 3D models of the components of the robot and to confirm the logical approach of the structural design by visual assembly. Accordingly, ADAMS should be utilized for motion simulation in order to get data on the fluctuation of motor torque in various motion states, informing the choice and setup of motors. For structural optimization, ANSYS Workbench is recommended for optimizing the strength and lightweight of important components to guarantee their reliability and durability in real-world applications. Thorough analysis of power design and motor size, together with static mechanical analysis, is necessary to evaluate possible failure modes and verify that the robot can deliver adequate suction during static adsorption circumstances. Essential design modifications should be implemented by comparing simulation results with real test data in order to improve the overall performance of the robot.
4.3. Leg Design and Gait Planning Optimization
In the design of the robot's legs, it is recommended that a three-degree-of-freedom 3-R structure be employed, wherein each leg is driven by a steering motor for rotational movement. The objective of this design is to optimize the placement of the motors and the mechanisms controlling their motion, with the aim of enhancing both the mobility and stability of the robot. By enhancing the kinematic and dynamic attributes of the legs, the robot can attain superior movement efficacy and adaptability to diverse surfaces. In the context of gait planning, it is advantageous to draw inspiration from the gait patterns of biological organisms, such as lizards or crocodiles, which have evolved efficient movement strategies. Figure 2 illustrates the linear walking gait diagram of the legged robot. The development of a gait planning scheme based on these biological models will facilitate a detailed analysis of the kinematic and dynamic properties of the robot, thereby improving its stability and efficiency of movement. Furthermore, optimizing the gait patterns will facilitate smooth locomotion in complex environments, thereby enhancing the robot's ability to navigate and perform tasks effectively. The refinement of the gait planning will facilitate enhanced adaptability and overall performance of the robot in a multitude of operational scenarios.
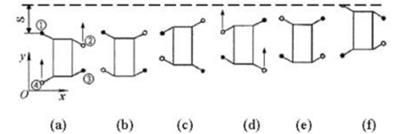
Figure 2. Gait Planning
5. Conclusion
This paper provides an overview of the present state of robotic technology advancements in cleaning glass curtain walls in tall buildings. It then thoroughly examines the uses, benefits, and constraints of adsorption technologies, motion modes, cleaning techniques, and path navigation. The results show that though the distinct technologies have contributed to enhancing the efficiency of wall-climbing robots, a number of issues remain unresolved, including the inflexibility of adsorption technologies on a variety of surfaces, limitations on the effectiveness of locomotion patterns in complex environments, the need for cleaning technologies to be more efficient and ecologically sustainable, and complex route planning that affects the robot's ability to operate in dynamic environments In order to tackle these problems, forthcoming research should prioritize the advancement of novel materials and technologies. While the current technological developments have greatly progressed the field of wall-climbing robots, ongoing investigation and improvement of associated technologies are necessary to attain broader applications and enhanced performance. This will facilitate the further advancement of glass curtain wall cleaning robot technology for tall buildings and enhance its efficiency and market competitiveness in real-world scenarios.
References
[1]. Wu, S., Wu, L. and Liu, T. (2011) Design of A Sliding Wall Climbing Robot With A Novel Negative Adsorption Device. 2011 8th International Conference on Ubiquitous Robots and Ambient Intelligence (URAI), 97-100.
[2]. Xu, J., Xu, L., Liu, J., Li, X. and Wu, X. (2018) Survey on Bioinspired Adhesive Methods and Design and Implementation of A Multi-Mode Biomimetic Wall-Climbing Robot. AIM 2018: 688-693.
[3]. Nansai, S. and Mohan, R.E. (2016) A Survey of Wall Climbing Robots: Recent Advances and Challenges. Robotics, 5(3):14.
[4]. Fang, Y., Wang, S., Bi, Q. et al. (2022) Design and Technical Development of Wall-Climbing Robots: A Review. J. Bionic. Eng. 19, 877-901.
[5]. Su, Y., et al. (2022) Design of Intelligent Cleaning Machine for Glass Exterior Wall. Agricultural Equipment & Vehicle Engineering, 9: 146-148.
[6]. Sariff, N. and Buniyamin, N. (2006) An Overview of Autonomous Mobile Robot Path Planning Algorithms. 2006 4th Student Conference on Research and Development, 183-188.
[7]. Zhu, J., et al. (2024) Review of Advancements in Wall Climbing Robot Techniques. Franklin Open.
[8]. Chen, S., Li, Y. and Kwok, N.M. (2011) Active vision in robotic systems: A survey of recent developments. The International Journal of Robotics Research, 30: 1343 - 1377.
[9]. Yang, L., et al. (2023) Path Planning Technique for Mobile Robots: A Review. Machines. 2023; 11(10): 980.
Cite this article
Zhang,K. (2024). Technological State and Optimization Analysis of High-Rise Glass Curtain Wall Cleaning Robots. Applied and Computational Engineering,102,148-154.
Data availability
The datasets used and/or analyzed during the current study will be available from the authors upon reasonable request.
Disclaimer/Publisher's Note
The statements, opinions and data contained in all publications are solely those of the individual author(s) and contributor(s) and not of EWA Publishing and/or the editor(s). EWA Publishing and/or the editor(s) disclaim responsibility for any injury to people or property resulting from any ideas, methods, instructions or products referred to in the content.
About volume
Volume title: Proceedings of the 2nd International Conference on Machine Learning and Automation
© 2024 by the author(s). Licensee EWA Publishing, Oxford, UK. This article is an open access article distributed under the terms and
conditions of the Creative Commons Attribution (CC BY) license. Authors who
publish this series agree to the following terms:
1. Authors retain copyright and grant the series right of first publication with the work simultaneously licensed under a Creative Commons
Attribution License that allows others to share the work with an acknowledgment of the work's authorship and initial publication in this
series.
2. Authors are able to enter into separate, additional contractual arrangements for the non-exclusive distribution of the series's published
version of the work (e.g., post it to an institutional repository or publish it in a book), with an acknowledgment of its initial
publication in this series.
3. Authors are permitted and encouraged to post their work online (e.g., in institutional repositories or on their website) prior to and
during the submission process, as it can lead to productive exchanges, as well as earlier and greater citation of published work (See
Open access policy for details).
References
[1]. Wu, S., Wu, L. and Liu, T. (2011) Design of A Sliding Wall Climbing Robot With A Novel Negative Adsorption Device. 2011 8th International Conference on Ubiquitous Robots and Ambient Intelligence (URAI), 97-100.
[2]. Xu, J., Xu, L., Liu, J., Li, X. and Wu, X. (2018) Survey on Bioinspired Adhesive Methods and Design and Implementation of A Multi-Mode Biomimetic Wall-Climbing Robot. AIM 2018: 688-693.
[3]. Nansai, S. and Mohan, R.E. (2016) A Survey of Wall Climbing Robots: Recent Advances and Challenges. Robotics, 5(3):14.
[4]. Fang, Y., Wang, S., Bi, Q. et al. (2022) Design and Technical Development of Wall-Climbing Robots: A Review. J. Bionic. Eng. 19, 877-901.
[5]. Su, Y., et al. (2022) Design of Intelligent Cleaning Machine for Glass Exterior Wall. Agricultural Equipment & Vehicle Engineering, 9: 146-148.
[6]. Sariff, N. and Buniyamin, N. (2006) An Overview of Autonomous Mobile Robot Path Planning Algorithms. 2006 4th Student Conference on Research and Development, 183-188.
[7]. Zhu, J., et al. (2024) Review of Advancements in Wall Climbing Robot Techniques. Franklin Open.
[8]. Chen, S., Li, Y. and Kwok, N.M. (2011) Active vision in robotic systems: A survey of recent developments. The International Journal of Robotics Research, 30: 1343 - 1377.
[9]. Yang, L., et al. (2023) Path Planning Technique for Mobile Robots: A Review. Machines. 2023; 11(10): 980.