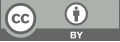
Optimization of Heat Reflective Triple Glazing Thickness Using the Artificial Fish Swarm Algorithm
- 1 City University of Macau
- 2 Dongguan University of Technology
- 3 Shanghai Ocean University
* Author to whom correspondence should be addressed.
Abstract
The significance of this research lies in its potential to improve energy efficiency in buildings, particularly in regions prone to high temperatures. By optimizing the thermal performance of heat-reflective glass, buildings can achieve better temperature regulation, leading to reduced dependency on air conditioning systems and lower energy costs. This research specifically targets the optimization of glass thickness, a critical factor influencing the overall effectiveness of heat-reflective glazing. The focus is on minimizing the transmitted light intensity for sunlight within the wavelength range of 300 to 2000 nm, which is vertically incident on the glass. The optimized glass thickness aims to maximize the thermal reflective properties while ensuring adequate natural light within indoor spaces. This study employs the Artificial Fish Swarm Algorithm to explore the optimal thickness of heat-reflective triple glazing. The effectiveness of this optimization method is assessed by comparing its results with experimental findings obtained through a systematic traversal of glazing thicknesses. The results highlight the potential of the Artificial Fish Swarm Algorithm to significantly enhance the thermal performance of heat-reflective glass, offering a practical solution for energy-efficient building design in hot climates.
Keywords
Glass, Artificial Fish Swarm Algorithm, Heat-Reflection, Light Intensity.
[1]. Zhang, Q. (2002). Glass begins to develop into multi-layer. Engineering Design CAD and Intelligent Building, (03), 77.
[2]. Lian, S., Yu, Y., Lin, S., Lin, G., Xu, C., & Wang, J. (2017). Design and calculation of optical anti-reflection and anti-reflection multilayer films. Materials Science, 7(1), 78-87.
[3]. Ma, L., & Yu, J. (2024). Preparation and properties of nanoparticle transparent thermal insulation coatings. Shanghai Coatings, 62(02), 12-16.
[4]. Zhu, X., Guo, C., Feng, H., Huang, Y., Feng, Y., Wang, X., & Wang, R. (2024). A Review of Key Technologies for Emotion Analysis Using Multimodal Information. Cognitive Computation, 1-27.
[5]. Zhang, H., An, Y., Xu, G., et al. (2022). Analysis of photothermal properties of single piece building glass. China Building Materials Science and Technology, 31(01), 22-24.
[6]. Liu, H., Feng, Q., & Liu, C. (2020). Thickness optimization of multilayer films using a modified artificial fish swarm algorithm. Journal of Applied Physics, 128(15), 153101.
[7]. Garlisi, C., Trepci, E., Li, X., Al Sakkaf, R., Al-Ali, K., Pereira Nogueira, R., Zheng, L., Azar, E., & Palmisano, G. (2020). Multilayer thin film structures for multifunctional glass: Self-cleaning, antireflective and energy-saving properties. Applied Energy, 264, 114697.
[8]. Zero Carbon Hub, (UK). Fabric energy effciency for zero carbon homes. Aflexible performance standard for 2016.www.zerocarbonhub.org accessed08.10.15].
[9]. Kumar K, Saboor S, Kumar V, et al. Experimental and theoretical studies of various solar control window glasses for the reduction of cooling and heating loads in buildings across different climatic regions[J]. Energy and Buildings, 2018, 173: 326-336.
[10]. Yamaç H İ, Koca A. Performance analysis of triple glazing water flow window systems during winter season[J]. Energy, 2023, 282: 128808.
[11]. Ismail K A R, Lago T G S, Lino F A M, et al. Experimental investigation on ventilated window with reflective film and development of correlations[J]. Solar Energy, 2021, 230: 421-434.
[12]. Gorantla K, Shaik S, Kontoleon K J, et al. Sustainable reflective triple glazing design strategies: Spectral characteristics, air-conditioning cost savings, daylight factors, and payback periods[J]. Journal of Building Engineering, 2021, 42: 103089.
[13]. Huang Y, Niu J, Chung T. Comprehensive analysis on thermal and daylighting performance of glazing and shading designs on office building envelope in cooling-dominant climates[J]. Applied energy, 2014, 134: 215-228.
[14]. Wang, R., Zhu, J., Wang, S., Wang, T., Huang, J., & Zhu, X. (2024). Multi-modal emotion recognition using tensor decomposition fusion and self-supervised multi-tasking. International Journal of Multimedia Information Retrieval, 13(4), 39.
[15]. Chen, Y. (2024). Influence analysis of exterior window glass on ultra-low energy consumption building. Shanghai Building Materials, (03), 22-24+28. Retrieved from [2024-08-21].
[16]. Farzaneh H, Malehmirchegini L, Bejan A, et al. Artificial intelligence evolution in smart buildings for energy efficiency[J]. Applied Sciences, 2021, 11(2): 763.
[17]. Si B, Tian Z, Jin X, et al. Performance indices and evaluation of algorithms in building energy efficient design optimization[J]. Energy, 2016, 114: 100-112.
[18]. Pourpanah F, Wang R, Lim C P, et al. A review of artificial fish swarm algorithms: Recent advances and applications[J]. Artificial Intelligence Review, 2023, 56(3): 1867-1903.
[19]. Li Xiaolei. A new intelligent optimization method-artificial fish swarm algorithm[D].Zhejiang University,2003.
[20]. JGJ 113-2015. (2015). Technical Regulations for the Application of Building Glass.
Cite this article
Huang,J.;Liu,J.;Shen,H. (2024). Optimization of Heat Reflective Triple Glazing Thickness Using the Artificial Fish Swarm Algorithm. Applied and Computational Engineering,94,40-48.
Data availability
The datasets used and/or analyzed during the current study will be available from the authors upon reasonable request.
Disclaimer/Publisher's Note
The statements, opinions and data contained in all publications are solely those of the individual author(s) and contributor(s) and not of EWA Publishing and/or the editor(s). EWA Publishing and/or the editor(s) disclaim responsibility for any injury to people or property resulting from any ideas, methods, instructions or products referred to in the content.
About volume
Volume title: Proceedings of CONF-MLA 2024 Workshop: Securing the Future: Empowering Cyber Defense with Machine Learning and Deep Learning
© 2024 by the author(s). Licensee EWA Publishing, Oxford, UK. This article is an open access article distributed under the terms and
conditions of the Creative Commons Attribution (CC BY) license. Authors who
publish this series agree to the following terms:
1. Authors retain copyright and grant the series right of first publication with the work simultaneously licensed under a Creative Commons
Attribution License that allows others to share the work with an acknowledgment of the work's authorship and initial publication in this
series.
2. Authors are able to enter into separate, additional contractual arrangements for the non-exclusive distribution of the series's published
version of the work (e.g., post it to an institutional repository or publish it in a book), with an acknowledgment of its initial
publication in this series.
3. Authors are permitted and encouraged to post their work online (e.g., in institutional repositories or on their website) prior to and
during the submission process, as it can lead to productive exchanges, as well as earlier and greater citation of published work (See
Open access policy for details).