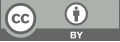
Research on Sensor Technology in Mobile Robot Navigation
- 1 The Stony Brook School, New York, USA
- 2 Beijing No.2 Middle school International Department, Beijing, China
- 3 Yiwu International Academy, Jinhua, China
* Author to whom correspondence should be addressed.
Abstract
As mobile robots are widely used in daily life, industrial manufacturing and the military, their ability to autonomous navigation in unmanned platforms and a wide range of environments is increasingly demanding. Therefore, the selection of sensors is a necessary process to improve the efficiency of navigation. The paper will introduce the principles and advantages of monocular vision, LIDAR and ultrasonic sensors etc. in detail, and then explore the advantages and disadvantages of various algorithms, and finally conclude optimal fusion of sensor solutions. The comparison results present that for the monocular vision, acquiring an image from a single camera setup, then using a YOLOv5 and mosaic technology to form a single image and finally using the improved RRT and the Frenet coordinate system to model the path is an efficient solution. 3D LIDAR technology can use the SLAM framework of graph optimization to create the map for obstacle avoidance and path planning. At last, this paper provides suggestions and optimizations for mobile robot navigation solutions, which are integrating multiple sensors and combining navigation solutions with machine learning.
Keywords
Mobile robot navigation, monocular vision, LiDAR 3D SLAM
[1]. Guo He. (2024). Obstacle distance estimation and obstacle avoidance path planning based on monocular vision (Master's degree thesis, Tianjin Vocational and Technical Normal University).https://link.cnki.net/doi/10.27711/d.cnki.gtjgc.2024.000141doi:10.27711/d.cnki.gtjgc.2024.000141.https://link.cnki.net/doi/10.27711/d.cnki.gtjgc.2024.000141doi:10.27711/d.cnki.gtjgc.2024.00014
[2]. Ji Siyu, Wang Yongsheng.(2022). Research on distance measurement method based on monocular vision and lidar. Ship Electronic Engineering (02),180-184.
[3]. Zhou Zhiguo, Cao Jiangwei, Di Shunfan. (2021). Overview of 3D Lidar SLAM algorithms. Chinese Journal of Scientific Instrument (09),13-27.doi:10.19650/j.cnki.cjsi.J2107897.
[4]. Li Z Y, Liu Q W and Pang Y. 2016. Review on forest parameters inversion using LiDAR. Journal of Remote Sensing, 20(5): 1138–1150 [DOI:10.11834/jrs.20165130]
[5]. Liu B, Yu Y, Jiang S. Review of advances in LiDAR detection and 3D imaging[J]. Opto-Electronic Engineering, 2019, 46(7): 190167
[6]. Yeong, D. J., Velasco-Hernandez, G., Barry, J., & Walsh, J. (2021). Sensor and sensor fusion technology in autonomous vehicles: A review. Sensors, 21(6), 2140.
[7]. A. O. Amole, M. O. Oyediran, O. O. Olusanya, W. A. Elegbede, A. T. Olusesi, A. O. Adeleye. 2020. Design and implementation of a prototype active infrared sensor controlled automatic sliding door for mitigation of coronavirus disease 2019 (COVID-19)
[8]. Mahdi, A. E., Azouz, A., Abdalla, A. E., & Abosekeen, A. (2022). A machine learning approach for an improved inertial navigation system solution. Sensors, 22(4), 1687.
Cite this article
Chen,Y.;Fan,Y.;Jin,M. (2024). Research on Sensor Technology in Mobile Robot Navigation. Applied and Computational Engineering,93,50-55.
Data availability
The datasets used and/or analyzed during the current study will be available from the authors upon reasonable request.
Disclaimer/Publisher's Note
The statements, opinions and data contained in all publications are solely those of the individual author(s) and contributor(s) and not of EWA Publishing and/or the editor(s). EWA Publishing and/or the editor(s) disclaim responsibility for any injury to people or property resulting from any ideas, methods, instructions or products referred to in the content.
About volume
Volume title: Proceedings of the 2nd International Conference on Machine Learning and Automation
© 2024 by the author(s). Licensee EWA Publishing, Oxford, UK. This article is an open access article distributed under the terms and
conditions of the Creative Commons Attribution (CC BY) license. Authors who
publish this series agree to the following terms:
1. Authors retain copyright and grant the series right of first publication with the work simultaneously licensed under a Creative Commons
Attribution License that allows others to share the work with an acknowledgment of the work's authorship and initial publication in this
series.
2. Authors are able to enter into separate, additional contractual arrangements for the non-exclusive distribution of the series's published
version of the work (e.g., post it to an institutional repository or publish it in a book), with an acknowledgment of its initial
publication in this series.
3. Authors are permitted and encouraged to post their work online (e.g., in institutional repositories or on their website) prior to and
during the submission process, as it can lead to productive exchanges, as well as earlier and greater citation of published work (See
Open access policy for details).