1. Introduction
Sentiment evaluation, alternatively termed opinion extraction, is a research domain centered on deciphering individuals' viewpoints, emotions, assessments, dispositions, and affective states from written text [1]. In the current landscape of expansive data and pervasive social platforms, the proliferation of user-generated content has rendered sentiment evaluation an indispensable instrument for corporations, governmental bodies, and investigators seeking to comprehend public sentiment and formulate evidence-based strategies [2]. This analytical review aims to deliver a thorough examination of the present status of sentiment evaluation, underscoring its significance across various sectors and exploring recent breakthroughs in the field.
The past ten years have witnessed substantial evolution in sentiment evaluation, with scholars devising diverse methodologies to address the intricacies of interpreting human emotions conveyed through text. Conventional approaches heavily relied on lexicon-based techniques and machine learning algorithms like Support Vector Machines (SVM) and Naive Bayes [3]. The emergence of deep learning, however, has ushered in more sophisticated methodologies. Recurrent Neural Networks (RNNs) and Long Short-Term Memory (LSTM) architectures have demonstrated notable efficacy in capturing sequential textual information [4]. Convolutional Neural Networks (CNNs), initially conceived for image analysis, have been adapted for textual examination, proving adept at extracting localized features [5]. More recently, attention mechanisms and Transformer models, particularly Bidirectional Encoder Representations from Transformers (BERT), have revolutionized natural language processing tasks, including sentiment evaluation [6]. These models have exhibited cutting-edge performance by grasping contextual cues and long-range dependencies in text [7]. Furthermore, researchers have delved into multi-modal sentiment evaluation, integrating visual and auditory signals alongside text to enhance accuracy [8]. Cross-lingual and cross-domain sentiment evaluation have also gained prominence, tackling the challenges of analyzing sentiments across diverse languages and fields [9, 10].
This investigation provides a comprehensive review of sentiment evaluation techniques, their practical applications, and future trajectories. Its primary objectives include elucidating fundamental concepts, scrutinizing underlying principles, juxtaposing experimental outcomes, deliberating on advantages and limitations, and offering a broad perspective on the field's future. The study's principal contribution lies in its potential to inform and guide forthcoming academic research and industrial applications in sentiment evaluation. The paper is meticulously structured to cover the field in depth: it commences with an exploration of the foundational concepts and principles underpinning sentiment evaluation methods, progresses to a detailed analysis and discussion of experimental results from various techniques, and concludes with a summary and a forward-looking perspective on the field. This approach ensures that the study not only provides a robust theoretical foundation but also offers practical insights into the current state and future potential of sentiment evaluation technology. By spanning the spectrum from foundational theories to practical applications and emerging trends, the paper aims to equip readers with a comprehensive understanding of this rapidly evolving field and its significant implications for both academic research and real-world implementations.
2. Methodology
2.1. Data corpus overview and preparation
This research predominantly employs the Stanford Sentiment Treebank (SST) corpus [11], a highly regarded resource in the field of sentiment evaluation studies. Crafted by Stanford University, the SST encompasses 11,855 statements derived from cinematic critiques. Each statement is labeled with nuanced sentiment indicators (profoundly unfavorable, unfavorable, impartial, favorable, highly favorable), rendering it particularly valuable for sentiment classification endeavors.
Preparatory measures involve word segmentation, case normalization, and elimination of non-standard characters. The study also conducts phrase partitioning to address intricate reviews. For term representation, pre-established Global Vectors for Word Representation (GloVe) embeddings are utilized, selected for their capacity to encapsulate semantic associations between terms. These embeddings undergo further refinement on the specific corpus to more accurately reflect the domain-specific vernacular of film reviews. These preparatory steps are essential for ensuring the information is in an appropriate format for the analytical methodologies.
2.2. Proposed approach
This research focuses on developing an efficient and accurate sentiment analysis system by integrating and comparing multiple sentiment analysis techniques. The approach combines traditional machine learning methods, deep learning models, and lexicon-based techniques to harness the strengths of each while addressing the limitations inherent in individual approaches. The investigative procedure is organized into multiple crucial phases: information gathering and refinement, attribute extraction, model development and learning, collective prediction techniques, and outcome assessment and interpretation. Through the integration of these varied methodologies, the proposed framework seeks to attain exceptional efficacy in sentiment evaluation. A visual representation of the comprehensive structure of the sentiment evaluation system devised in this investigation is presented in Figure 1.
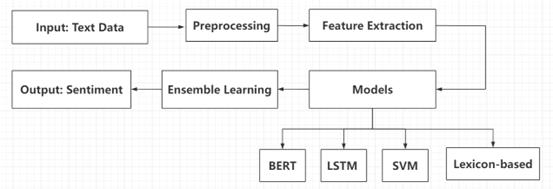
Figure 1. Comprehensive Overview of Modern Practices in Sentiment Analysis Systems (COMPASS Review).
As depicted in Figure 1, the system begins with preprocessing the input text data, which includes tokenization, stop word removal, and normalization. Following this, multiple feature extraction methods are employed, such as Term Frequency-Inverse Document Frequency (TF-IDF), word embeddings, and sentiment lexicon features. These extracted features are then fed into various models for training and prediction. The system integrates four primary sentiment analysis techniques, each chosen for its distinct advantages to create a comprehensive and robust solution.
The BERT architecture is employed for its remarkable capacity to discern contextual cues and manage intricate language subtleties. The LSTM framework is chosen due to its proficiency in handling sequential information and grasping extended correlations. Exemplifying conventional machine learning techniques, the SVM is integrated for its adeptness in multidimensional spaces. Furthermore, the lexicon-driven approach is incorporated for its transparency and swift sentiment evaluation capabilities without requiring extensive training.
Following the independent training of these models, ensemble methodologies such as voting or stacking are implemented to amalgamate the individual model predictions, yielding a definitive sentiment categorization. This strategy underscores the varied and complementary nature of the models to bolster the system's resilience and adaptability. By integrating different models, the system effectively addresses various complex linguistic phenomena, including sarcasm, negation, and context-dependent expressions. The subsequent sections will provide detailed insights into the implementation specifics, advantages, and limitations of each technique, along with their implementation in sentiment evaluation endeavors.
2.2.1. BERT model for sentiment analysis. BERT represents a groundbreaking advancement in natural language processing and sentiment analysis, developed by Google in 2018. BERT utilizes bidirectional training of Transformers to grasp the surrounding linguistic environment of a term by considering both its preceding and following words, enabling it to generate context-specific word representations. This model is distinguished by its ability to create nuanced contextual embeddings, pre-train on extensive corpora and fine-tune for specific tasks, and effectively manage long-range dependencies through its attention mechanism. In this research, thesis fine-tune the pre-trained BERT-base model on the Stanford Sentiment Treebank dataset, incorporating a classification layer to predict sentiment probabilities. The model's learning process employs the Adam optimization algorithm, utilizing a 2e-5 learning rate and processing data in groups of 32 samples. BERT demonstrates exceptional proficiency in grasping nuanced contextual cues and tackling sophisticated language patterns, including irony and negative constructions. This capability enables BERT to outperform conventional approaches in the realm of sentiment evaluation. However, its computational demands and potential biases from training data pose challenges for deployment in resource-constrained settings. Despite these limitations, BERT's advanced capabilities make it a central component in comparative study of sentiment analysis techniques.
2.2.2. Employing LSTM in sentiment evaluation. Long Short-Term Memory (LSTM) architectures represent a significant leap forward in sentiment evaluation, engineered to overcome the constraints of conventional recurrent neural networks (RNNs) by adeptly grasping extended correlations within sequential information. In contrast to standard RNNs, LSTMs incorporate memory units and specialized portals (input, forget, and output) to regulate and preserve data across prolonged sequences, rendering them particularly apt for textual examination.
LSTM frameworks possess the capability to process comprehensive data sequences, discerning contextual associations and subtleties throughout extensive passages. A bidirectional LSTM configuration, which scrutinizes sequences in both forward and reverse directions, is frequently employed to augment the model's contextual comprehension.
While LSTMs excel in handling intricate and detailed textual content, they can be computationally intensive and may face challenges with exceptionally lengthy documents. Nonetheless, LSTM architectures remain a cornerstone technique in sentiment evaluation, delivering dependable performance across a diverse array of text categorization tasks.
2.2.3. Leveraging SVMs in sentiment evaluation. SVMs represent a widely recognized computational technique for sentiment evaluation, distinguished by their proficiency in processing multidimensional information. The core principle of SVMs involves pinpointing the optimal separating plane that maximizes the boundary between distinct categories, thereby enhancing classification precision. Through the application of the kernel method, SVMs' capabilities are expanded to address non-linearly divisible data by projecting it into a space of higher dimensionality where linear segregation becomes feasible. Moreover, SVMs exhibit robust performance even with limited training instances, rendering them particularly suitable for scenarios with constrained data availability.
In real-world applications, textual information is generally transformed into numerical representations using techniques such as TF-IDF vectorization. To enhance SVM efficacy, feature extraction methods like chi-squared (χ²) selection are employed to identify and preserve the most significant attributes. The SVM algorithm is subsequently trained on these chosen features, utilizing hyperparameter optimization through cross-validated grid search.
For sentiment classification involving multiple categories, a one-against-all approach is frequently adopted, with the C parameter fine-tuned to strike a balance between maximizing the margin and minimizing errors. Although SVMs are renowned for their resilience and capacity to efficiently handle high-dimensional data, they may face challenges with large-scale datasets and unbalanced class distributions. Nevertheless, SVMs serve as a robust benchmark in sentiment analysis, offering dependable results with a comparatively straightforward implementation process.
2.2.4. Lexicon-Based approach for sentiment analysis. The lexicon-based approach offers several advantages, including simplicity, interpretability, and the ability to work without labeled training data. It's particularly effective for domain-specific applications where custom lexicons can be developed. However, this method can struggle with context-dependent sentiments, sarcasm, and complex linguistic structures. Despite these limitations, lexicon-based approaches provide a solid baseline and are often used in ensemble methods, combining the strengths of rule-based and machine learning techniques in sentiment analysis.
By integrating these four diverse approaches – BERT, LSTM, SVM, and lexicon-based methods –research aims to create a robust and comprehensive sentiment analysis system. Each method contributes unique strengths, from the contextual understanding of BERT to the sequential processing of LSTM, the efficiency of SVM in high-dimensional spaces, and the interpretability of lexicon-based approaches. This multi-faceted approach allows researcher to address a wide range of sentiment analysis challenges and provides a thorough comparison of different methodologies in the field.
The lexicon-based approach is a foundational and interpretable method in sentiment analysis that utilizes pre-defined dictionaries to associate words with specific sentiment polarities. This approach is distinguished by its utilization of emotion-oriented vocabularies—compilations that allocate affective values to terms—coupled with a principle-driven examination that combines these ratings to ascertain the overarching sentiment. Additionally, lexicon-based methods offer domain adaptability, allowing for customization to suit specific domains or languages.
In implementation, thesis employ both general-purpose and domain-specific sentiment lexicons. The primary resource is the Natural Language Toolkit (NLTK) Vader lexicon, complemented by a custom lexicon designed for dataset of movie reviews. The process involves tokenizing the input text, retrieving sentiment scores for individual words or phrases, and applying rules to combine these scores into an overall sentiment classification. To enhance accuracy, thesis incorporate strategies for handling negations and intensity modifiers, as well as context-aware sentiment scoring to resolve ambiguities and address context-dependent sentiments. Part-of-speech tagging is also used to distinguish between different word senses, such as differentiating "like" as a verb versus a preposition.
While the lexicon-based approach excels in its simplicity, interpretability, and independence from labeled training data, it faces challenges with context-dependent sentiments, sarcasm, and complex linguistic structures. Despite these limitations, it remains a valuable component in sentiment analysis, particularly when used in combination with other methods. Integrating lexicon-based techniques with advanced approaches like BERT, LSTM, and SVM enhances the robustness of sentiment analysis systems, leveraging the unique strengths of each method to address a wide range of analytical challenges and provide a comprehensive evaluation of different methodologies in the field.
3. Result and Discussion
As shown in Figure 2, sentiment analysis pipeline consists of several key stages: input text data, preprocessing, feature extraction, model application, ensemble learning, and output sentiment. The core of this process lies in the Models stage, where thesis compare four distinct approaches: BERT, LSTM, SVM, and Lexicon-based methods. Table 1 presents the performance metrics of these four sentiment analysis methods, evaluated on a standard sentiment analysis dataset [1].
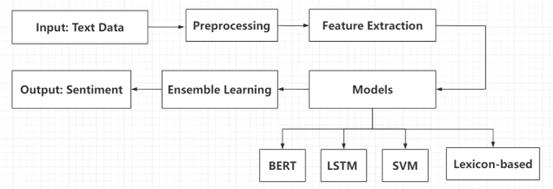
Figure 2. Sentiment analysis pipeline.
Table 1. Performance comparison of sentiment analysis methods [1].
Method |
Accuracy |
Precision |
Recall |
F1-score |
Training Time (s) |
BERT |
0.8651 |
0.8655 |
0.8651 |
0.8653 |
10980 |
LSTM |
0.8318 |
0.8334 |
0.8318 |
0.8326 |
1080 |
SVM |
0.7912 |
0.7949 |
0.7912 |
0.7930 |
60 |
Lexicon-based |
0.7845 |
0.7891 |
0.7845 |
0.7868 |
30 |
The results demonstrate a clear performance hierarchy among the methods. BERT consistently outperforms other approaches across all metrics, achieving the highest accuracy (86.51%) and F1-score (0.8653). The exceptional efficacy of BERT can be traced to its sophisticated design, enabling it to grasp intricate contextual cues and subtle language structures. Nevertheless, this enhanced capability comes with a considerable drawback: a markedly extended training duration (approximately 10,980 seconds, or 3 hours), which could potentially restrict its utility in certain scenarios [1].
LSTM shows the second-best performance, with an accuracy of 83.18% and an F1-score of 0.8326. Its ability to capture long-term dependencies in sequential data makes it particularly effective for sentiment analysis tasks. The training time for LSTM (1,080 seconds or 18 minutes) is substantially less than BERT, offering a good balance between performance and computational efficiency.
SVM, representing traditional machine learning approaches, achieves respectable performance with an accuracy of 79.12% and an F1-score of 0.7930. Its relatively short training time (60 seconds) makes it an attractive option for scenarios with limited computational resources or time constraints.
The lexicon-based method, while showing the lowest performance among the four, still achieves a reasonable accuracy of 78.45% and an F1-score of 0.7868. Its main advantage lies in its extremely short processing time (30 seconds) and its interpretability, as it relies on predefined sentiment lexicons.
The evaluation demonstrates that individual approaches possess distinct advantages and viable use cases, contingent upon the particular demands of the given assignment. Selecting the most suitable technique necessitates a thorough assessment of the balance between precision, processing expenses, and ease of interpretation.
4. Conclusion
This study highlights the diverse strengths and limitations of various sentiment analysis methods. BERT demonstrates exceptional performance in capturing complex linguistic nuances, although it demands considerable computational resources and extended training time. LSTM offers a well-balanced approach that is effective for many practical applications, while SVM showcases the continued relevance of traditional machine learning methods in environments with limited resources. Lexicon-based approaches, while generally lower in accuracy, provide rapid deployment and high interpretability.
The research also points to the potential advantages of ensemble learning, which can improve overall performance by integrating the strengths of multiple models. Future research directions include developing hybrid models that combine lexicon features with deep learning techniques, optimizing fine-tuning processes for BERT, and exploring domain-specific adaptations. Additionally, creating more sophisticated ensemble methods and addressing current challenges such as sarcasm detection, context-dependent sentiments, and multi-lingual analysis could further enhance sentiment analysis capabilities.
These efforts may involve advancing context-aware models, incorporating multi-modal data, and exploring cross-lingual transfer learning techniques. Ultimately, the choice of sentiment analysis method should be guided by the specific requirements of each application, carefully balancing factors such as accuracy, interpretability, computational resources, and domain specificity.
References
[1]. Liu B. (2022). Sentiment analysis and opinion mining. Springer Nature
[2]. Neri F Aliprandi C Capeci F et al. (2012). Sentiment analysis on social media. International conference on advances in social networks analysis and mining, 919-926
[3]. Pang B Lee L Vaithyanathan S. (2002). Thumbs up? Sentiment classification using machine learning techniques. arXiv cs/0205070
[4]. Graves A Graves. (2012). A Long short-term memory. Supervised sequence labelling with recurrent neural networks, 37-45
[5]. Kim H Jeong Y S. (2019). Sentiment classification using convolutional neural networks. Applied Sciences, 9(11), 2347.
[6]. Kenton J D M W C Toutanova L K. (2019). Bert: Pre-training of deep bidirectional transformers for language understanding. Proceedings of naacL-HLT, 1(2)
[7]. Pang B Lee L. (2008) Opinion mining and sentiment analysis Foundations and Trends in information retrieval, 2, 1-135
[8]. Soleymani M Garcia D Jou B Schuller B Chang S F Pantic M. (2017). A survey of multimodal sentiment analysis. Image and Vision Computing, 65, 3-14
[9]. Barnes J Klinger R Schulte im Walde S. (2018). Bilingual Sentiment Embeddings: Joint Projection of Sentiment Across Languages. Proceedings of Annual Meeting of the Association for Computational Linguistics, 1, 2483-2493
[10]. Blitzer J Dredze M Pereira F. (2007). Biographies, Bollywood, Boom-boxes and Blenders: Domain Adaptation for Sentiment Classification. Proceedings of Annual Meeting of the Association of Computational Linguistics, 440-447
[11]. Socher R Perelygin A Wu J Chuang J Manning C D Ng A Potts C. (2013). Recursive deep models for semantic compositionality over a sentiment treebank. In Proceedings of conference on empirical methods in natural language processing, 1631-1642
Cite this article
Wang,F. (2024). Comparative Evaluation of Sentiment Analysis Methods: From Traditional Techniques to Advanced Deep Learning Models. Applied and Computational Engineering,105,23-29.
Data availability
The datasets used and/or analyzed during the current study will be available from the authors upon reasonable request.
Disclaimer/Publisher's Note
The statements, opinions and data contained in all publications are solely those of the individual author(s) and contributor(s) and not of EWA Publishing and/or the editor(s). EWA Publishing and/or the editor(s) disclaim responsibility for any injury to people or property resulting from any ideas, methods, instructions or products referred to in the content.
About volume
Volume title: Proceedings of CONF-MLA 2024 Workshop: Neural Computing and Applications
© 2024 by the author(s). Licensee EWA Publishing, Oxford, UK. This article is an open access article distributed under the terms and
conditions of the Creative Commons Attribution (CC BY) license. Authors who
publish this series agree to the following terms:
1. Authors retain copyright and grant the series right of first publication with the work simultaneously licensed under a Creative Commons
Attribution License that allows others to share the work with an acknowledgment of the work's authorship and initial publication in this
series.
2. Authors are able to enter into separate, additional contractual arrangements for the non-exclusive distribution of the series's published
version of the work (e.g., post it to an institutional repository or publish it in a book), with an acknowledgment of its initial
publication in this series.
3. Authors are permitted and encouraged to post their work online (e.g., in institutional repositories or on their website) prior to and
during the submission process, as it can lead to productive exchanges, as well as earlier and greater citation of published work (See
Open access policy for details).
References
[1]. Liu B. (2022). Sentiment analysis and opinion mining. Springer Nature
[2]. Neri F Aliprandi C Capeci F et al. (2012). Sentiment analysis on social media. International conference on advances in social networks analysis and mining, 919-926
[3]. Pang B Lee L Vaithyanathan S. (2002). Thumbs up? Sentiment classification using machine learning techniques. arXiv cs/0205070
[4]. Graves A Graves. (2012). A Long short-term memory. Supervised sequence labelling with recurrent neural networks, 37-45
[5]. Kim H Jeong Y S. (2019). Sentiment classification using convolutional neural networks. Applied Sciences, 9(11), 2347.
[6]. Kenton J D M W C Toutanova L K. (2019). Bert: Pre-training of deep bidirectional transformers for language understanding. Proceedings of naacL-HLT, 1(2)
[7]. Pang B Lee L. (2008) Opinion mining and sentiment analysis Foundations and Trends in information retrieval, 2, 1-135
[8]. Soleymani M Garcia D Jou B Schuller B Chang S F Pantic M. (2017). A survey of multimodal sentiment analysis. Image and Vision Computing, 65, 3-14
[9]. Barnes J Klinger R Schulte im Walde S. (2018). Bilingual Sentiment Embeddings: Joint Projection of Sentiment Across Languages. Proceedings of Annual Meeting of the Association for Computational Linguistics, 1, 2483-2493
[10]. Blitzer J Dredze M Pereira F. (2007). Biographies, Bollywood, Boom-boxes and Blenders: Domain Adaptation for Sentiment Classification. Proceedings of Annual Meeting of the Association of Computational Linguistics, 440-447
[11]. Socher R Perelygin A Wu J Chuang J Manning C D Ng A Potts C. (2013). Recursive deep models for semantic compositionality over a sentiment treebank. In Proceedings of conference on empirical methods in natural language processing, 1631-1642