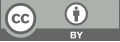
Learning Analytics Based on Big Data: Student Behavior Prediction and Personalized Educational Strategy Formulation
- 1 Hunan Institute of Technology, Hunan, China
* Author to whom correspondence should be addressed.
Abstract
Learning analytics have become a game-changer in education by using big data to analyse student behaviours, predict student outcomes and provide personalised interventions. This paper outlines the main components in learning analytics including data collection, predictive modelling and personalised educational strategies. It demonstrates how predictive models can be used to identify at-risk students and why real-time feedback can keep students engaged and motivated. Two case studies and examples of the data are used to illustrate how institutions can shift from reactive to proactive mode using learning analytics to track engagement, performance, and personalise the learning path. The study also shows that learning technologies are becoming adaptive to personalise learning experience, which results in a more learner-centric approach to education catering for the individual needs of students. Overall, the study demonstrates the role of learning analytics in creating a data-driven environment, which improves the student learning success and retention by addressing the challenges ahead of time.
Keywords
Learning analytics, big data, predictive modeling, student engagement, at-risk students.
[1]. Caspari-Sadeghi, Sima. "Learning assessment in the age of big data: Learning analytics in higher education." Cogent Education 10.1 (2023): 2162697.
[2]. Yassine, S., S. Kadry, and M. A. Sicilia. "Learning analytics and learning objects repositories: Overview and future directions." Learning, Design, and Technology: an international compendium of theory, research, practice, and policy (2023): 3503-3532.
[3]. Freire, Manuel, et al. "Game learning analytics: Learning analytics for serious games." Learning, design, and technology: An international compendium of theory, research, practice, and policy. Cham: Springer International Publishing, 2023. 3475-3502.
[4]. Nguyen, Andy, et al. "Examining socially shared regulation and shared physiological arousal events with multimodal learning analytics." British Journal of Educational Technology 54.1 (2023): 293-312.
[5]. Ijeh, Scholastica, et al. "Predictive modeling for disease outbreaks: a review of data sources and accuracy." International Medical Science Research Journal 4.4 (2024): 406-419.
[6]. Khodadadi, Ehsaneh, and S. K. Towfek. "Internet of Things Enabled Disease Outbreak Detection: A Predictive Modeling System." Journal of Intelligent Systems & Internet of Things 10.1 (2023).
[7]. Igwama, Geneva Tamunobarafiri, et al. "Big data analytics for epidemic forecasting: Policy Frameworks and technical approaches." International Journal of Applied Research in Social Sciences 6.7 (2024): 1449-1460.
[8]. Jain, Arushi, et al. "Optimized levy flight model for heart disease prediction using CNN framework in big data application." Expert Systems with Applications 223 (2023): 119859.
[9]. Farayola, Oluwatoyin Ajoke, et al. "Advancements in predictive analytics: A philosophical and practical overview." World Journal of Advanced Research and Reviews 21.3 (2024): 240-252.
[10]. Egwim, Christian Nnaemeka, et al. "Comparison of machine learning algorithms for evaluating building energy efficiency using big data analytics." Journal of Engineering, Design and Technology 22.4 (2024): 1325-1350.
[11]. Yuan, Shuai, Brigitte Kroon, and Astrid Kramer. "Building prediction models with grouped data: A case study on the prediction of turnover intention." Human Resource Management Journal 34.1 (2024): 20-38.
Cite this article
Luo,X. (2024). Learning Analytics Based on Big Data: Student Behavior Prediction and Personalized Educational Strategy Formulation. Applied and Computational Engineering,116,7-13.
Data availability
The datasets used and/or analyzed during the current study will be available from the authors upon reasonable request.
Disclaimer/Publisher's Note
The statements, opinions and data contained in all publications are solely those of the individual author(s) and contributor(s) and not of EWA Publishing and/or the editor(s). EWA Publishing and/or the editor(s) disclaim responsibility for any injury to people or property resulting from any ideas, methods, instructions or products referred to in the content.
About volume
Volume title: Proceedings of the 5th International Conference on Signal Processing and Machine Learning
© 2024 by the author(s). Licensee EWA Publishing, Oxford, UK. This article is an open access article distributed under the terms and
conditions of the Creative Commons Attribution (CC BY) license. Authors who
publish this series agree to the following terms:
1. Authors retain copyright and grant the series right of first publication with the work simultaneously licensed under a Creative Commons
Attribution License that allows others to share the work with an acknowledgment of the work's authorship and initial publication in this
series.
2. Authors are able to enter into separate, additional contractual arrangements for the non-exclusive distribution of the series's published
version of the work (e.g., post it to an institutional repository or publish it in a book), with an acknowledgment of its initial
publication in this series.
3. Authors are permitted and encouraged to post their work online (e.g., in institutional repositories or on their website) prior to and
during the submission process, as it can lead to productive exchanges, as well as earlier and greater citation of published work (See
Open access policy for details).