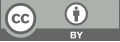
Comparison Impacts of Iteration Quantities on Loss Function for Different Data Sets
- 1 School of Physics, Sichuan University, Chengdu, China
* Author to whom correspondence should be addressed.
Abstract
As machine learning is applied to more and more high-tech fields, optimizing tasks has become a key step in the pursuit of more efficient and accurate artificial intelligence. Thes study will present an overview for machine learning and address the implementation of distributed machine learning, including cleaning the collected datasets, selecting different models, and discussing the influence of loss function types (L-smooth and non-L-smooth) on error convergence under the premise of increasing the number of iterations. Experiments show that the two types of loss functions have opposite results. For the L-smooth loss function, larger iterations reduce the error convergence. Regarding to the non-L-Smooth loss function, larger iterations increase the error convergence. This research also adjusted other model parameters and analyzed them, which has more meaningful implications for the parameter selection of machine learning model training in the future, and one can optimize error convergence by changing the type of loss function, so that improve the efficiency of scientific research.
Keywords
Distributed machine learning, artificial intelligence, loss function.
[1]. Dehghani M and Yazdanparast Z 2023 From distributed machine to distributed deep learning: a comprehensive survey Journal of Big Data vol 10(1) p 158
[2]. Bojarski M 2016 End to end learning for self-driving cars arXiv preprint arXiv:160407316
[3]. Khandani A E, Kim A J and Lo A W 2010 Consumer credit-risk models via machine-learning algorithms Journal of Banking & Finance vol 34(11) pp 2767-2787
[4]. Verbraeken J, Wolting M, Katzy J, Kloppenburg J, Verbelen T and Rellermeyer J S 2020 A survey on distributed machine learning Acm computing surveys (csur) vol 53(2) pp 1-33
[5]. Peteiro-Barral D and Guijarro-Berdiñas B 2013 A survey of methods for distributed machine learning Progress in Artificial Intelligence vol 2 pp 1-11
[6]. Xing E P, Ho Q, Xie P and Wei D 2016 Strategies and principles of distributed machine learning on big data Engineering vol 2(2) pp 179-195
[7]. Khromov G and Singh S P 2023 Some intriguing aspects about lipschitz continuity of neural networks arXiv preprint arXiv:230210886
[8]. Pan L and Song S 2023 Local SGD accelerates convergence by exploiting second order information of the loss function arXiv preprint arXiv:230515013
[9]. Bian W and Chen X 2012 Smoothing neural network for constrained non-Lipschitz optimization with applications IEEE transactions on neural networks and learning systems vol 23(3) pp 399-411
[10]. Osowski S, Siwek K and Markiewicz T 2004 June MLP and SVM networks-a comparative study Proceedings of the 6th Nordic Signal Processing Symposium 2004 NORSIG 2004 pp 37-40
[11]. Desai M and Shah M 2021 An anatomization on breast cancer detection and diagnosis employing multi-layer perceptron neural network (MLP) and Convolutional neural network (CNN Clinical eHealth vol 4 pp 1-11
[12]. Ramraj S and Uzir N Sunil R Banerjee S 2016 Experimenting XGBoost algorithm for prediction and classification of different datasets International Journal of Control Theory and Applications vol 9(40) pp 651-662
[13]. Torlay L, Perrone-Bertolotti M, Thomas E and Baciu M 2017 Machine learning–XGBoost analysis of language networks to classify patients with epilepsy Brain informatics vol 4 pp 159-169
[14]. Dhaliwal S S, Nahid A A and Abbas R 2018 Effective intrusion detection system using XGBoost Information vol 9(7) p 149
Cite this article
Wu,D. (2024). Comparison Impacts of Iteration Quantities on Loss Function for Different Data Sets. Applied and Computational Engineering,96,68-78.
Data availability
The datasets used and/or analyzed during the current study will be available from the authors upon reasonable request.
Disclaimer/Publisher's Note
The statements, opinions and data contained in all publications are solely those of the individual author(s) and contributor(s) and not of EWA Publishing and/or the editor(s). EWA Publishing and/or the editor(s) disclaim responsibility for any injury to people or property resulting from any ideas, methods, instructions or products referred to in the content.
About volume
Volume title: Proceedings of the 2nd International Conference on Machine Learning and Automation
© 2024 by the author(s). Licensee EWA Publishing, Oxford, UK. This article is an open access article distributed under the terms and
conditions of the Creative Commons Attribution (CC BY) license. Authors who
publish this series agree to the following terms:
1. Authors retain copyright and grant the series right of first publication with the work simultaneously licensed under a Creative Commons
Attribution License that allows others to share the work with an acknowledgment of the work's authorship and initial publication in this
series.
2. Authors are able to enter into separate, additional contractual arrangements for the non-exclusive distribution of the series's published
version of the work (e.g., post it to an institutional repository or publish it in a book), with an acknowledgment of its initial
publication in this series.
3. Authors are permitted and encouraged to post their work online (e.g., in institutional repositories or on their website) prior to and
during the submission process, as it can lead to productive exchanges, as well as earlier and greater citation of published work (See
Open access policy for details).