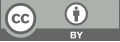
Reviews on Transformer-based Models for Financial Time Series Forecasting
- 1 School of Science, The Hong Kong University of Science and Technology, Hong Kong, China
* Author to whom correspondence should be addressed.
Abstract
The emergence of competitive deep learning models has increasing attached attention from both the academia and industry. Thus, as one of the fields that tend to chase the state-of-art and fashion technological trend, some previous work in financial time series forecasting has turned to deep learning models, including transformer-based models. While an examination work questioning the effectiveness of transformers for general time series forecasting (TSF) in 2022, researchers are keen to work on the creative design of transformer-based neural network architectures and related improvements. On the other hand, since the success of ChatGPT in 2023 as the milestone of transformers and Large Language Models (LLMs), an alternative method is put forward that implements domain-specific LLM in financial text to obtain sentiment information or generate trading signals, which does not solve the forecasting problem but provide support in decision making in investment. This review will scan through the history of the above models and methodologies in financial time series forecasting.
Keywords
Transformer, Time Series Forecasting, Financial Time Series
[1]. Liu, Y., Han, T., Ma, S., Zhang, J., Yang, Y., Tian, J., ... & Ge, B. (2023). Summary of ChatGPT- related research and perspective towards the future of large language models. Meta-Radiology, 100017. https://doi.org/10.1016/j.metrad.2023.100017
[2]. Vaswani, A. (2017). Attention is all you need. Advances in Neural Information Processing Systems. https://doi.org/10.48550/arXiv.1706.03762
[3]. Lim, B., & Zohren, S. (2021). Time-series forecasting with deep learning: a survey. Philosophical Transactions of the Royal Society A, 379(2194), 20200209. https://doi.org/10.1098/rsta.2020. 0209
[4]. Wen, Q., Zhou, T., Zhang, C., Chen, W., Ma, Z., Yan, J., & Sun, L. (2022). Transformers in time series: A survey. arXiv preprint arXiv:2202.07125. https://doi.org/10.48550/arXiv.2202. 07125
[5]. Bishop, C. M., & Bishop, H. (2023). Deep learning: Foundations and concepts. Springer Nature. 357-74. https://doi.org/10.1007/978-3-031-45468-4
[6]. Zou, J., Zhao, Q., Jiao, Y., Cao, H., Liu, Y., Yan, Q., ... & Shi, J. Q. (2022). Stock market prediction via deep learning techniques: A survey. arXiv preprint arXiv:2212.12717. https:// doi.org/10.48550/arXiv.2212.12717
[7]. Yang, H., Liu, X. Y., & Wang, C. D. (2023). Fingpt: Open-source financial large language models. arXiv preprint arXiv:2306.06031. https://doi.org/10.48550/arXiv.2306.06031
[8]. Wu, S., Irsoy, O., Lu, S., Dabravolski, V., Dredze, M., Gehrmann, S., ... & Mann, G. (2023). Bloomberggpt: A large language model for finance. arXiv preprint arXiv:2303.17564. https:/ /doi.org/10.48550/arXiv.2303.17564
[9]. Ba, J. L. (2016). Layer normalization. arXiv preprint arXiv:1607.06450. https://doi.org/10.48550/ arXiv.1607.06450
[10]. Li, S., Jin, X., Xuan, Y., Zhou, X., Chen, W., Wang, Y. X., & Yan, X. (2019). Enhancing the locality and breaking the memory bottleneck of transformer on time series forecasting. Advances in neural information processing systems, 32. https://doi.org/10.48550/arXiv.1907. 00235
[11]. Zeng, A., Chen, M., Zhang, L., & Xu, Q. (2023, June). Are transformers effective for time series forecasting? In Proceedings of the AAAI conference on artificial intelligence (Vol. 37, No. 9, pp. 11121-11128). https://doi.org/10.48550/arXiv.2205.13504
[12]. Zhou, H., Zhang, S., Peng, J., Zhang, S., Li, J., Xiong, H., & Zhang, W. (2021, May). Informer: Beyond efficient transformer for long sequence time-series forecasting. In Proceedings of the AAAI conference on artificial intelligence (Vol. 35, No. 12, pp. 11106-11115). https://doi.org/ 10.48550/arXiv.2012.07436
[13]. Nie, Y., Nguyen, N. H., Sinthong, P., & Kalagnanam, J. (2022). A time series is worth 64 words: Long-term forecasting with transformers. arXiv preprint arXiv:2211.14730. https://doi.org/10. 48550/arXiv.2211.14730
[14]. Liu, Y., Hu, T., Zhang, H., Wu, H., Wang, S., Ma, L., & Long, M. (2023). itransformer: Inverted transformers are effective for time series forecasting. arXiv preprint arXiv:2310.06625. https: //doi.org/10.48550/arXiv.2310.06625
[15]. Han, C., He, Z., & Toh, A. J. W. (2023). Pairs trading via unsupervised learning. European Journal of Operational Research, 307(2), 929-947. https://doi.org/10.1016/j.ejor.2022.09.041
[16]. Devlin, J. (2018). Bert: Pre-training of deep bidirectional transformers for language understanding. arXiv preprint arXiv:1810.04805. https://doi.org/10.48550/arXiv.1810.04805
[17]. Yang, Y., Uy, M. C. S., & Huang, A. (2020). Finbert: A pretrained language model for financial communications. arXiv preprint arXiv:2006.08097. https://doi.org/10.48550/arXiv.2006. 08097
Cite this article
Lin,H. (2024). Reviews on Transformer-based Models for Financial Time Series Forecasting. Applied and Computational Engineering,96,130-133.
Data availability
The datasets used and/or analyzed during the current study will be available from the authors upon reasonable request.
Disclaimer/Publisher's Note
The statements, opinions and data contained in all publications are solely those of the individual author(s) and contributor(s) and not of EWA Publishing and/or the editor(s). EWA Publishing and/or the editor(s) disclaim responsibility for any injury to people or property resulting from any ideas, methods, instructions or products referred to in the content.
About volume
Volume title: Proceedings of the 2nd International Conference on Machine Learning and Automation
© 2024 by the author(s). Licensee EWA Publishing, Oxford, UK. This article is an open access article distributed under the terms and
conditions of the Creative Commons Attribution (CC BY) license. Authors who
publish this series agree to the following terms:
1. Authors retain copyright and grant the series right of first publication with the work simultaneously licensed under a Creative Commons
Attribution License that allows others to share the work with an acknowledgment of the work's authorship and initial publication in this
series.
2. Authors are able to enter into separate, additional contractual arrangements for the non-exclusive distribution of the series's published
version of the work (e.g., post it to an institutional repository or publish it in a book), with an acknowledgment of its initial
publication in this series.
3. Authors are permitted and encouraged to post their work online (e.g., in institutional repositories or on their website) prior to and
during the submission process, as it can lead to productive exchanges, as well as earlier and greater citation of published work (See
Open access policy for details).