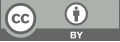
Recent Advances in Visual SLAM Algorithms in Dynamic Environments
- 1 Shien-Ming Wu School of Intelligent Engineering, South China University of Technology, Guangzhou, China
* Author to whom correspondence should be addressed.
Abstract
This paper reviews the latest research progress of visual SLAM in dynamic scenes. The improved algorithms dependent on deep learning and multi-sensor fusion are discussed. First of all, the challenges of traditional visual SLAM in dynamic environments are stated, especially the interference of high dynamic objects on system feature extraction, pose estimation and mapping. Then, the application of several object detection technologies such as YOLOv5 and YOLOv7 in dynamic visual SLAM was analyzed in detail. These methods significantly improve the positioning precision and robustness of the system by identifying and eliminating dynamic feature points in the scene. In addition, this paper explores the visual SLAM system combining semantic segmentation and geometric constraint optimization, and finally introduces the multi-sensor SLAM method combining IMU data, point cloud data and visual information. Experimental results show that these improved algorithms have a significant improvement in positioning accuracy and system robustness compared with traditional visual SLAM algorithms under TUM and other dynamic data sets.
Keywords
Visual SLAM, dynamic scenes, object detection, semantic segmentation, multi-sensor fusion.
[1]. Huang Y. R., Wang Z. F., Han T., & Song H. P. (2024). Dynamic visual SLAM algorithm combining lightweight YOLOv5s. Electronic Measurement Technology, 1-12.
[2]. Wang H. Y., Wu Y. Z., Chen L. J., & Chen X. (2024). Visual SLAM algorithm based on YOLOv5 and geometric constraints in dynamic scenes. Packaging Engineering, (03), 208-217. https://doi.org/10.19554/j.cnki.1001-3563.2024.03.024.
[3]. Wang Y. (2023). Research on indoor dynamic visual SLAM and loop detection based on semantic segmentation (Master’s thesis, Xi'an University of Technology). https://doi.org/10.27398/d.cnki.gxalu.2023.000286.
[4]. Wang H. X. (2021). Research on visual SLAM-IMU integrated navigation system (Master’s thesis, Harbin Engineering University). https://doi.org/10.27060/d.cnki.ghbcu.2021.000594.
[5]. Shi T., Xiao N. Z., Ding Y., & Xu J. D. (2024). Visual SLAM algorithm integrating improved YOLOv7 in dynamic scenes. Foreign Electronic Measurement Technology, (07), 90-96. https://doi.org/10.19652/j.cnki.femt.2406101.
[6]. Liu Y. Z., Xu X. R., Zhang H., & Yu Q. S. (2024). Visual SLAM algorithm based on semantic and geometric constraints in dynamic scenes. Information and Control, (03), 388-399. https://doi.org/10.13976/j.cnki.xk.2024.3089.
[7]. Fu H., Xu H. G., Zhang Z. M., & Qi S. H. (2021). Visual simultaneous localization and mapping in dynamic scenes based on semantic and optical flow constraints. Computer Applications, (11), 3337-3344.
[8]. Jiang H. C., Liu Y. Q., Peng J. Q., Li J. M., Zhu D. C., & Zhang X. L. (2021). RGB-D SLAM algorithm based on semantic prior and depth constraints in indoor dynamic scenes. Information and Control, (03), 275-286. https://doi.org/10.13976/j.cnki.xk.2021.0167.
[9]. Bao B. Z., Zhan X. B., Yu D., Si Y., Duan J., & Shi T. L. (2024). Dynamic SLAM algorithm based on radar and camera fusion. Instrumentation Technology and Sensors, (07), 105-109.
[10]. Wang Z., & Xiu C. D. (2023). A visual/IMU fusion localization method in dynamic environments. In Proceedings of the 17th National Conference on Signal and Intelligent Information Processing and Applications (pp. 180-184). Beijing University of Aeronautics and Astronautics.
Cite this article
Li,M. (2024). Recent Advances in Visual SLAM Algorithms in Dynamic Environments. Applied and Computational Engineering,80,128-135.
Data availability
The datasets used and/or analyzed during the current study will be available from the authors upon reasonable request.
Disclaimer/Publisher's Note
The statements, opinions and data contained in all publications are solely those of the individual author(s) and contributor(s) and not of EWA Publishing and/or the editor(s). EWA Publishing and/or the editor(s) disclaim responsibility for any injury to people or property resulting from any ideas, methods, instructions or products referred to in the content.
About volume
Volume title: Proceedings of CONF-MLA Workshop: Mastering the Art of GANs: Unleashing Creativity with Generative Adversarial Networks
© 2024 by the author(s). Licensee EWA Publishing, Oxford, UK. This article is an open access article distributed under the terms and
conditions of the Creative Commons Attribution (CC BY) license. Authors who
publish this series agree to the following terms:
1. Authors retain copyright and grant the series right of first publication with the work simultaneously licensed under a Creative Commons
Attribution License that allows others to share the work with an acknowledgment of the work's authorship and initial publication in this
series.
2. Authors are able to enter into separate, additional contractual arrangements for the non-exclusive distribution of the series's published
version of the work (e.g., post it to an institutional repository or publish it in a book), with an acknowledgment of its initial
publication in this series.
3. Authors are permitted and encouraged to post their work online (e.g., in institutional repositories or on their website) prior to and
during the submission process, as it can lead to productive exchanges, as well as earlier and greater citation of published work (See
Open access policy for details).