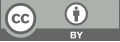
Optimizing E-commerce Recommender Systems: A Comprehensive Review of Techniques and Future Directions
- 1 School of Engineering, University of Connecticut, CT, USA
* Author to whom correspondence should be addressed.
Abstract
This paper examines recommender systems in e-commerce by reviewing technologies and real-world applications and identifying the importance of big data analytics in recommender systems. In the study, three kinds of recommendation algorithms are discussed: collaborative filtering, content-based recommendation, and hybrid models. Collaborative filtering methods did well in the case of large-scale user data, but had cold-start and sparsity problems. Based on the content, recommended methods have strong personalized suggestion functions, but the “information cocoon” phenomenon is a risk, which decreases the contents’ diversity. Hybrid models are a combination approach between the two techniques, providing a flexible and robustness solution, but only a more complex computationally. This article also looks at the technology trends that have emerged lately, for example, the use of deep learning models, as well as the privacy-preserving techniques utilized in recommender systems. By analyzing and summarizing the existing research, this paper provides a reference basis for future optimization and application of recommender systems and points out potential research directions.
Keywords
Data Analytics, E-Commerce, Recommender Systems, Collaborative Filtering.
[1]. Roy, D., & Dutta, M. (2022). A systematic review and research perspective on recommender systems. Journal of Big Data, 9(1), 1–36. https://doi.org/10.1186/s40537-022-00592-5
[2]. Huy, P. Q., & Phuc, V. K. (2023). Big data in relation with business intelligence capabilities and e-commerce during COVID-19 pandemic in accountant’s perspective. Future Business Journal, 9(1), 40–21. https://doi.org/10.1186/s43093-023-00221-4
[3]. Wang, J., Lee, L. K., Wu, N. I. (2023). A Systematic Review of Recommendation System Based on Deep Learning Methods. In: Nedjah, N., Martínez Pérez, G., Gupta, B.B. (eds) International Conference on Cyber Security, Privacy and Networking (ICSPN 2022). ICSPN 2021. Lecture Notes in Networks and Systems, vol 599. Springer, Cham. https://doi.org/10.1007/978-3-031-22018-0_12
[4]. Akter, S., & Wamba, S. F. (2016). Big data analytics in E-commerce: a systematic review and agenda for future research. Electronic Markets, 26(2), 173–194. https://doi.org/10.1007/s12525-016-0219-0
[5]. Cirqueira, D., Hofer, M., Nedbal, D., Helfert, M., & Bezbradica, M. (2020). Customer Purchase Behavior Prediction in E-commerce: A Conceptual Framework and Research Agenda. In New Frontiers in Mining Complex Patterns (pp. 119–136). Springer International Publishing. https://doi.org/10.1007/978-3-030-48861-1_8
[6]. Alsmadi, A. A., Shuhaiber, A., Al-Okaily, M., Al-Gasaymeh, A., & Alrawashdeh, N. (2023). Big data analytics and innovation in e-commerce: current insights and future directions. Journal of Financial Services Marketing, 1–18. https://doi.org/10.1057/s41264-023-00235-7
[7]. Chen, Y., Li, C., Wang H. Big Data and Predictive Analytics for Business Intelligence: A Bibliographic Study (2000–2021). Forecasting. 2022; 4(4):767-786. https://doi.org/10.3390/forecast4040042
[8]. Nguyen, L. V., Vo, Q.-T., & Nguyen, T.-H. (2023). Adaptive KNN-Based Extended Collaborative Filtering Recommendation Services. Big Data and Cognitive Computing, 7(2), 106-. https://doi.org/10.3390/bdcc7020106
[9]. Chakraborty, S., Hoque, Md. S., Rahman Jeem, N., Biswas, M. C., Bardhan, D., & Lobaton, E. (2021). Fashion Recommendation Systems, Models and Methods: A Review. Informatics (Basel), 8(3), 49-. https://doi.org/10.3390/informatics8030049
Cite this article
Wu,Z. (2024). Optimizing E-commerce Recommender Systems: A Comprehensive Review of Techniques and Future Directions. Applied and Computational Engineering,97,96-101.
Data availability
The datasets used and/or analyzed during the current study will be available from the authors upon reasonable request.
Disclaimer/Publisher's Note
The statements, opinions and data contained in all publications are solely those of the individual author(s) and contributor(s) and not of EWA Publishing and/or the editor(s). EWA Publishing and/or the editor(s) disclaim responsibility for any injury to people or property resulting from any ideas, methods, instructions or products referred to in the content.
About volume
Volume title: Proceedings of the 2nd International Conference on Machine Learning and Automation
© 2024 by the author(s). Licensee EWA Publishing, Oxford, UK. This article is an open access article distributed under the terms and
conditions of the Creative Commons Attribution (CC BY) license. Authors who
publish this series agree to the following terms:
1. Authors retain copyright and grant the series right of first publication with the work simultaneously licensed under a Creative Commons
Attribution License that allows others to share the work with an acknowledgment of the work's authorship and initial publication in this
series.
2. Authors are able to enter into separate, additional contractual arrangements for the non-exclusive distribution of the series's published
version of the work (e.g., post it to an institutional repository or publish it in a book), with an acknowledgment of its initial
publication in this series.
3. Authors are permitted and encouraged to post their work online (e.g., in institutional repositories or on their website) prior to and
during the submission process, as it can lead to productive exchanges, as well as earlier and greater citation of published work (See
Open access policy for details).