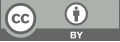
Enhancing DF-GAN for Text-to-Image Synthesis: Improved Text-Encoding and Network Structure
- 1 School of Statistics and Mathematics, Shandong University of Finance and Economics, Shandong, China
- 2 School of Electronic Engineering and Computer Science, Queen Marry University of London, London, United Kingdom
* Author to whom correspondence should be addressed.
Abstract
Text-to-image synthesis is one of the most challenging and popular tasks in machine learning, with many models developed to improve performance in this area. Deep Fusion Generative Adversarial Networks (DF-GAN) is a straightforward but efficient model for image generation, but it has three key limitations. First, it only supports sentence-level textual descriptions, restricting its ability to extract fine-grained features from word-level inputs. Second, the structure of the residual layers and blocks, along with key parameters, could be optimized for better performance. Third, existing evaluation metrics, such as Fréchet Inception Distance (FID), tend to place undue emphasis on irrelevant features like background, which is problematic when the focus is on generating specific objects. To address these issues, we introduced a new text encoder that enhances the model having capacity to process word-level descriptions, leading to more precise and text-consistent image generation. Additionally, we optimized key parameters and redesigned the convolutional and residual network structures, resulting in higher-quality images and reduced running time. Lastly, we proposed a new evaluation theory tailored to assess the quality of specific objects within the generated images. These improvements make the enhanced DF-GAN more effective in generating high-quality, text-aligned images efficiently.
Keywords
Text-to-Image, DF-GAN, Generative Adversarial Networks, Fréchet Inception Distance.
[1]. Ramesh A Pavlov M Goh G Gray S 2021 Zero-shot text-to-image generation. In International conference on machine learning pp 8821-8831
[2]. Goodfellow I Pouget-Abadie J Mehdi M Xu B Warde-Farley D Sherjil O Aaron C and Yoshua B 2014 Generative adversarial nets In Advances in Neural Information Processing Systems pp 2672–2680.
[3]. Chen Y Liu J Peng L Wu Y 2024 Auto-encoding variational bayes Cambridge Explorations in Arts and Sciences 2(1)
[4]. Ho J Jain A Abbeel P 2020 Denoising diffusion probabilistic models Advances in neural information processing systems 33 pp 6840-6851
[5]. Takeru M Masanori K 2018 cGANs with projection discriminator arXiv Preprint:1802.05637
[6]. Tao X Pengchuan Z Qiuyuan H Han Z Zhe G Huang X L and He X D 2018 Attngan: Fine-grained text to image generation with attentional generative adversarial networks In Proceedings of the IEEE conference on computer vision and pattern recognition pp 1316-1324
[7]. Qiao T T Zhang J Xu D Q and Tao D C 2019 Mirrorgan: Learning text-to-image generation by redescription. In Proceedings of the IEEE Conference on Computer Vision and Pattern Recognition pp 1505–1514
[8]. Zhu M F Pan P B Chen W and Yang Y 2019 Dm-gan: Dynamic memory generative adversarial networks for text-to-image synthesis In Proceedings of the IEEE Conference on Computer Vision and Pattern Recognition pp 5802-5810
[9]. Tao M Tang H Wu F Jing X Y 2022 Df-gan: A simple and effective baseline for text-to-image synthesis In Proceedings of the IEEE/CVF conference on computer vision and pattern recognition pp 16515-16525
[10]. Catherine W Branson S Welinder P Perona P Belongie S 2011 The Caltech-UCSD Birds-200-2011 Dataset California Institute of Technology
[11]. Heusel M Ramsauer H Unterthiner T 2017 Gans trained by a two time-scale update rule converge to a local nash equilibrium Advances in neural information processing systems 30
[12]. Dinh T M Nguyen R Hua B S 2022 Tise: Bag of metrics for text-to-image synthesis evaluation European Conference on Computer Vision. Cham: Springer Nature Switzerland pp 594-609
Cite this article
Wu,Y.;Zhou,Z. (2024). Enhancing DF-GAN for Text-to-Image Synthesis: Improved Text-Encoding and Network Structure. Applied and Computational Engineering,107,83-91.
Data availability
The datasets used and/or analyzed during the current study will be available from the authors upon reasonable request.
Disclaimer/Publisher's Note
The statements, opinions and data contained in all publications are solely those of the individual author(s) and contributor(s) and not of EWA Publishing and/or the editor(s). EWA Publishing and/or the editor(s) disclaim responsibility for any injury to people or property resulting from any ideas, methods, instructions or products referred to in the content.
About volume
Volume title: Proceedings of the 2nd International Conference on Machine Learning and Automation
© 2024 by the author(s). Licensee EWA Publishing, Oxford, UK. This article is an open access article distributed under the terms and
conditions of the Creative Commons Attribution (CC BY) license. Authors who
publish this series agree to the following terms:
1. Authors retain copyright and grant the series right of first publication with the work simultaneously licensed under a Creative Commons
Attribution License that allows others to share the work with an acknowledgment of the work's authorship and initial publication in this
series.
2. Authors are able to enter into separate, additional contractual arrangements for the non-exclusive distribution of the series's published
version of the work (e.g., post it to an institutional repository or publish it in a book), with an acknowledgment of its initial
publication in this series.
3. Authors are permitted and encouraged to post their work online (e.g., in institutional repositories or on their website) prior to and
during the submission process, as it can lead to productive exchanges, as well as earlier and greater citation of published work (See
Open access policy for details).