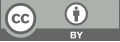
BERT and RoBERTa for Sarcasm Detection: Optimizing Performance through Advanced Fine-tuning
- 1 Jacobs School of Engineering, University of California, San Diego, CA, USA
* Author to whom correspondence should be addressed.
Abstract
This paper presents an advanced approach to sarcasm detection in online discourse using state-of-the-art language models. The study systematically evolves Bidirectional Encoder Representations from Transformers (BERT) and Robustly Optimized BERT Pretraining Approach (RoBERTa) architectures from baseline to optimized versions, demonstrating significant improvements in sarcasm detection accuracy. Utilizing a balanced subset of 30,000 samples from a Reddit sarcasm dataset, the research implements gradual unfreezing, adaptive learning rates, and sophisticated regularization techniques. The final RoBERTa model achieves 76.80% accuracy, outperforming BERT and showing balanced precision and recall across sarcastic and non-sarcastic classes. The comparative analysis reveals interesting learning dynamics between BERT and RoBERTa, with RoBERTa demonstrating superior performance in later training stages. The study highlights the importance of architectural innovations and advanced training strategies in capturing the nuanced linguistic cues of sarcasm. While computational constraints limited the dataset size, the research provides valuable insights into model behavior and sets a foundation for future work. The paper concludes by discussing potential avenues for advancement, including scaling to larger datasets, exploring multi-modal approaches, and developing more interpretable models, ultimately contributing to the broader field of natural language understanding and affective computing.
Keywords
Sarcasm Detection, Bidirectional Encoder Representations from Transformers, Robustly Optimized BERT Pretraining Approach, Text Classification, Sentiment Analysis.
[1]. Amir, S., Wallace, B. C., Lyu, H., Silva, P. C. (2016). Modelling context with user embeddings for sarcasm detection in social media. arXiv preprint arXiv:1607.00976.
[2]. Poria, S., Cambria, E., Hazarika, D., Vij, P. (2016). A deeper look into sarcastic tweets using deep convolutional neural networks. arXiv preprint arXiv:1610.08815.
[3]. Hazarika, D., Poria, S., Gorantla, S., Cambria, E., Zimmermann, R., Mihalcea, R. (2018). Cascade: Contextual sarcasm detection in online discussion forums. arXiv preprint arXiv:1805.06413..
[4]. Liu, Y. (2019). Roberta: A robustly optimized bert pretraining approach. arXiv preprint arXiv:1907.11692.
[5]. Kumar, P., Sarin, G. (2022). WELMSD–word embedding and language model based sarcasm detection. Online Information Review. 46(7):1242-56.
[6]. Onan, A., Toçoğlu, M. A. (2021). A term weighted neural language model and stacked bidirectional LSTM based framework for sarcasm identification. Ieee Access. 9:7701-22.
[7]. Zhou, J. (2023). An evaluation of state-of-the-art large language models for sarcasm detection. arXiv preprint arXiv:2312.03706.
[8]. Dadu, T., Pant, K. (2020). Sarcasm detection using context separators in online discourse. In Proceedings of the Second Workshop on Figurative Language Processing (pp. 51-55).
[9]. Mao, J., Liu, W. (2019). A BERT-based Approach for Automatic Humor Detection and Scoring. IberLEF@ SEPLN. 2421:197-202.
[10]. Howard, J., Ruder, S. (2018). Universal language model fine-tuning for text classification. arXiv preprint arXiv:1801.06146.
[11]. Dai, W., Yu, T., Liu, Z., Fung, P. (2020). Kungfupanda at semeval-2020 task 12: Bert-based multi-task learning for offensive language detection. arXiv preprint arXiv:2004.13432.
[12]. Sarsam, S. M., Al-Samarraie, H., Alzahrani, A. I., Wright, B. (2020). Sarcasm detection using machine learning algorithms in Twitter: A systematic review. International Journal of Market Research. 62(5):578-98.
Cite this article
Shu,X. (2024). BERT and RoBERTa for Sarcasm Detection: Optimizing Performance through Advanced Fine-tuning. Applied and Computational Engineering,97,1-11.
Data availability
The datasets used and/or analyzed during the current study will be available from the authors upon reasonable request.
Disclaimer/Publisher's Note
The statements, opinions and data contained in all publications are solely those of the individual author(s) and contributor(s) and not of EWA Publishing and/or the editor(s). EWA Publishing and/or the editor(s) disclaim responsibility for any injury to people or property resulting from any ideas, methods, instructions or products referred to in the content.
About volume
Volume title: Proceedings of the 2nd International Conference on Machine Learning and Automation
© 2024 by the author(s). Licensee EWA Publishing, Oxford, UK. This article is an open access article distributed under the terms and
conditions of the Creative Commons Attribution (CC BY) license. Authors who
publish this series agree to the following terms:
1. Authors retain copyright and grant the series right of first publication with the work simultaneously licensed under a Creative Commons
Attribution License that allows others to share the work with an acknowledgment of the work's authorship and initial publication in this
series.
2. Authors are able to enter into separate, additional contractual arrangements for the non-exclusive distribution of the series's published
version of the work (e.g., post it to an institutional repository or publish it in a book), with an acknowledgment of its initial
publication in this series.
3. Authors are permitted and encouraged to post their work online (e.g., in institutional repositories or on their website) prior to and
during the submission process, as it can lead to productive exchanges, as well as earlier and greater citation of published work (See
Open access policy for details).