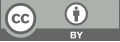
Leveraging LSTM Neural Networks for Stock Price Prediction and Trading Strategy Optimization in Financial Markets
- 1 Master of Public Administration, Columbia University, New York City, USA
- 2 Master of Business Administration, Columbia University, NY, USA
- 3 Business Analytics and Project Management, University of Connecticut, CT, USA
* Author to whom correspondence should be addressed.
Abstract
In the Artificial Intelligence development market in recent years, the atmosphere felt by the financial field will be relatively strong, and some, especially in the deep learning model, widely exist in the performance of complex financial data, which has certain advantages. Therefore, as one of the most prominent models of deep learning, LSTM neural network models are just good at processing some complex financial data of the rest of the time series, such as stock price prediction and trading strategy, optimization, and so on. However, in the actual application process, the stock price prediction of such models still has certain data quality, historical data market fluctuations, complex, non-linear data and other related factors, so there are certain challenges and development space in the process of processing.Nevertheless, by properly addressing these issues and combining them with best practices, LSTM algorithms are a powerful tool to help uncover underlying patterns in financial markets and optimize trading decisions.
Keywords
Long short-term memory Network (LSTM), Stock price forecasting, Trading strategy optimization, Artificial Intelligence (AI)
[1]. Ta, V. D., Liu, C. M., & Tadesse, D. A. (2020). Portfolio optimization-based stock prediction using long-short term memory network in quantitative trading. Applied Sciences, 10(2), 437.
[2]. Namdari, A., & Li, Z. S. (2018). Integrating Fundamental and Technical Analysis of Stock Market through Multi-layer Perceptron. 2018 IEEE Technology and Engineering Management Conference (TEMSCON) .
[3]. Singh, P., Jha, M., Sharaf, M., El-Meligy, M. A., & Gadekallu, T. R. (2023). Harnessing a hybrid CNN-LSTM model for portfolio performance: A case study on stock selection and optimization. Ieee Access, 11, 104000-104015.
[4]. Koo, E., & Kim, G. (2022). A hybrid prediction model integrating garch models with a distribution manipulation strategy based on lstm networks for stock market volatility. IEEE Access, 10, 34743-34754.
[5]. Wu, J. M. T., Sun, L., Srivastava, G., & Lin, J. C. W. (2021). A long short-term memory network stock price prediction with leading indicators. Big Data, 9(5), 343-357.
[6]. Zhu, Y., Yu, K., Wei, M., Pu, Y., & Wang, Z. (2024). AI-Enhanced Administrative Prosecutorial Supervision in Financial Big Data: New Concepts and Functions for the Digital Era. Social Science Journal for Advanced Research, 4(5), 40-54.
[7]. Zhao, Fanyi, et al. "Application of Deep Reinforcement Learning for Cryptocurrency Market Trend Forecasting and Risk Management." Journal of Industrial Engineering and Applied Science 2.5 (2024): 48-55.
[8]. Wang, S., Zhu, Y., Lou, Q., & Wei, M. (2024). Utilizing Artificial Intelligence for Financial Risk Monitoring in Asset Management. Academic Journal of Sociology and Management, 2(5), 11-19.
[9]. Shen, Q., Wen, X., Xia, S., Zhou, S., & Zhang, H. (2024). AI-Based Analysis and Prediction of Synergistic Development Trends in US Photovoltaic and Energy Storage Systems. International Journal of Innovative Research in Computer Science & Technology, 12(5), 36-46.
[10]. Zhu, Y., Yu, K., Wei, M., Pu, Y., & Wang, Z. (2024). AI-Enhanced Administrative Prosecutorial Supervision in Financial Big Data: New Concepts and Functions for the Digital Era. Social Science Journal for Advanced Research, 4(5), 40-54.
[11]. Li, H., Zhou, S., Yuan, B., & Zhang, M. (2024). OPTIMIZING INTELLIGENT EDGE COMPUTING RESOURCE SCHEDULING BASED ON FEDERATED LEARNING. Journal of Knowledge Learning and Science Technology ISSN: 2959-6386 (online), 3(3), 235-260.
Cite this article
Ju,C.;Shen,Q.;Ni,X. (2024). Leveraging LSTM Neural Networks for Stock Price Prediction and Trading Strategy Optimization in Financial Markets. Applied and Computational Engineering,112,47-53.
Data availability
The datasets used and/or analyzed during the current study will be available from the authors upon reasonable request.
Disclaimer/Publisher's Note
The statements, opinions and data contained in all publications are solely those of the individual author(s) and contributor(s) and not of EWA Publishing and/or the editor(s). EWA Publishing and/or the editor(s) disclaim responsibility for any injury to people or property resulting from any ideas, methods, instructions or products referred to in the content.
About volume
Volume title: Proceedings of the 5th International Conference on Signal Processing and Machine Learning
© 2024 by the author(s). Licensee EWA Publishing, Oxford, UK. This article is an open access article distributed under the terms and
conditions of the Creative Commons Attribution (CC BY) license. Authors who
publish this series agree to the following terms:
1. Authors retain copyright and grant the series right of first publication with the work simultaneously licensed under a Creative Commons
Attribution License that allows others to share the work with an acknowledgment of the work's authorship and initial publication in this
series.
2. Authors are able to enter into separate, additional contractual arrangements for the non-exclusive distribution of the series's published
version of the work (e.g., post it to an institutional repository or publish it in a book), with an acknowledgment of its initial
publication in this series.
3. Authors are permitted and encouraged to post their work online (e.g., in institutional repositories or on their website) prior to and
during the submission process, as it can lead to productive exchanges, as well as earlier and greater citation of published work (See
Open access policy for details).