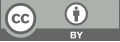
MFCC-based Classification of Carotid Artery Doppler Audio Signals Using LSTM Network
- 1 Department of Biomedical Engineering, Indian Institute of Technology, Hyderabad, India
- 2 Kamineni Hospital, King Koti, Hyderabad, India
- 3 Department of Biomedical Engineering, Indian Institute of Technology, Hyderabad, India
- 4 Department of Biomedical Engineering, Indian Institute of Technology, Hyderabad, India
* Author to whom correspondence should be addressed.
Abstract
The carotid artery assessment is essential for detecting stenosis and vascular abnormalities. Traditional Doppler ultrasound, while effective, requires specialized equipment and trained operators, limiting its accessibility in primary care. This study investigates Doppler audio signal analysis as a non-invasive, cost-effective alternative for assessing carotid artery hemodynamics. Using advanced signal processing techniques like mel-frequency cepstral coefficients (MFCCs) and deep learning models such as Long Short-Term Memory (LSTM) networks, we analyze Doppler audio signals from the common carotid artery (CCA) in 216 individuals. Our findings reveal significant age-related variations in blood flow dynamics and distinct signal patterns, highlighting the potential of Doppler audio analysis for early vascular screening. The changes in MFCCs indicate their usefulness in identifying hemodynamic alterations associated with aging and disease, supporting their role in non-invasive carotid artery health assessment. We also evaluate the deep learning framework, utilizing RNNs to capture long-term dependencies in the signals and providing a comprehensive comparison of network configurations and performance relative to state-of-the-art algorithms.
Keywords
Common Carotid Artery, Doppler Ultrasound Audio, Recurrent Neural Network, MFCC Features, Age related variations
[1]. Dabbaghchian, S., Ghaemmaghami, M.P., & Esmailpour, M. (2010). Feature extraction using MFCC. Proceedings of the 2010 International Conference on Multimedia Computing and Information Technology (MCIT), 17–20.
[2]. Huynh, H.T., & Ha, Q.M. (2020). Voice disorder classification based on MFCC and SVM. Journal of Computer Science and Cybernetics, 36(3), 201–210.
[3]. Abdar, M., Pourpanah, F., Hussain, S., Rezazadegan, D., Liu, L., Ghavamzadeh, M., ... Nahavandi, S. (2021). A review of deep learning techniques for medical image segmentation. Artificial Intelligence in Medicine, 114, 102066. https://doi.org/10.1016/j.artmed.2021.102066
[4]. Zhang, Z., Cui, P., & Liu, W. (2020). Deep learning for healthcare: Review, opportunities, and challenges. Briefings in Bioinformatics, 22(2), 805–818. https://doi.org/10.1093/bib/bbz092
[5]. Suhn, T., et al. (2019). Design of an auscultation system for phonoangiography and monitoring of carotid artery diseases. Proceedings of the Annual International Conference of the IEEE Engineering in Medicine and Biology Society (EMBS), 1776–1779.
[6]. Iverson, B.L., & Dervan, P.B. (2006). The cervical bruit: sound spectral analysis related to severity of carotid arterial disease. Clinical Cardiology, 29(10), 462–465.
[7]. Boussaa, M., Atouf, I., Atibi, M., & Bennis, A. (2016). ECG signals classification using MFCC coefficients and ANN classifier. Proceedings of the 2nd International Conference on Electrical and Information Technologies (ICEIT).
[8]. Farzam, B., & Shirazi, J. (2014). The diagnosis of heart diseases based on PCG signals using MFCC coefficients and SVM classifier. IJISET - International Journal of Innovative Science, Engineering & Technology, 1(10).
[9]. Deng, M., Meng, T., Cao, J., Wang, S., Zhang, J., & Fan, H. (2020). Heart sound classification based on improved MFCC features and convolutional recurrent neural networks. Neural Networks, 130, 22–32.
[10]. Mu, X., & Min, C.H. (2023). MFCC as features for speaker classification using machine learning. Proceedings of the IEEE Conference on Machine Learning and Applications, 79-8-3503-3761-7/23/$31.00 ©2023 IEEE.
[11]. Abbas, S.N., Abo-Zahhad, M., Ahmed, S.M., & Farrag, M. (2016). Heart-ID: human identity recognition using heart sounds based on modifying mel-frequency cepstral features. IET Biometrics, 5(4), 284–296. https://doi.org/10.1049/iet-bmt.2015.0033
Cite this article
V,V.G.T.;Ghori,I.;Eranki,A.;John,R. (2024). MFCC-based Classification of Carotid Artery Doppler Audio Signals Using LSTM Network. Applied and Computational Engineering,100,36-42.
Data availability
The datasets used and/or analyzed during the current study will be available from the authors upon reasonable request.
Disclaimer/Publisher's Note
The statements, opinions and data contained in all publications are solely those of the individual author(s) and contributor(s) and not of EWA Publishing and/or the editor(s). EWA Publishing and/or the editor(s) disclaim responsibility for any injury to people or property resulting from any ideas, methods, instructions or products referred to in the content.
About volume
Volume title: Proceedings of the 5th International Conference on Signal Processing and Machine Learning
© 2024 by the author(s). Licensee EWA Publishing, Oxford, UK. This article is an open access article distributed under the terms and
conditions of the Creative Commons Attribution (CC BY) license. Authors who
publish this series agree to the following terms:
1. Authors retain copyright and grant the series right of first publication with the work simultaneously licensed under a Creative Commons
Attribution License that allows others to share the work with an acknowledgment of the work's authorship and initial publication in this
series.
2. Authors are able to enter into separate, additional contractual arrangements for the non-exclusive distribution of the series's published
version of the work (e.g., post it to an institutional repository or publish it in a book), with an acknowledgment of its initial
publication in this series.
3. Authors are permitted and encouraged to post their work online (e.g., in institutional repositories or on their website) prior to and
during the submission process, as it can lead to productive exchanges, as well as earlier and greater citation of published work (See
Open access policy for details).