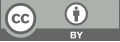
Rocket Recycling via Reinforcement Learning
- 1 Worcester Academy, Worcester, MA, USA
* Author to whom correspondence should be addressed.
Abstract
Rocket recycling offers significant potential to reduce launch costs, increase launch frequency, and reduce space debris, as evidenced by SpaceX’s success with rocket relandings. Traditional model-based control methods face challenges in handling the complex, non-linear dynamics inherent in the rocket recycling process. Reinforcement learning (RL), by contrast, provides a flexible, data-driven approach which is better suited to managing these complicate dynamic conditions. In this paper, we investigate two research problems in RL-based rocket recycling control: 1) how the granularity of control variables (e.g. thrust force levels) impacts the control performance; 2) how the environmental interference during training affects the robustness of RL controller. Our evaluation results demonstrate that increasing the granularity of control variables significantly slows the convergence of RL-based controller to a stable state. Moreover, introducing environmental interference during training—such as wind disturbances—improves both the efficiency and robustness of the RL-based controller. Our findings provide insights into how the RL-based controller can be more effectively used for rocket recycling tasks and highlight considerations that can enhance its applicability and robustness in dynamic environments.
Keywords
rocket recycling, reinforcement learning, convergence, robustness
[1]. Space Economy Institute (2023). The Role of Reusable Rockets in Reducing the Cost of Access to Space. https://spaceeconomyinstitute.com/2023/08/24/the-role-of-reusable-rockets-in-reducing-the-cost-of-access-to-space/
[2]. Dokumaci, Korhan, M. Timur Aydemir, and Metin U. Salamci. "Modeling, PID control and simulation of a rocket launcher system." 2014 16th International Power Electronics and Motion Control Conference and Exposition. IEEE, 2014.
[3]. Wang, Jinbo, Naigang Cui, and Changzhu Wei. "Optimal rocket landing guidance using convex optimization and model predictive control." Journal of guidance, control, and dynamics 42, no. 5 (2019): 1078-1092.
[4]. Xue, Shuai, Zhaolei Wang, Hongyang Bai, Chunmei Yu, and Zian Li. "Research on Self-Learning Control Method of Reusable Launch Vehicle Based on Neural Network Architecture Search." Aerospace 11, no. 9 (2024): 774.
[5]. Li, Wenting, Xiuhui Zhang, Yunfeng Dong, Yan Lin, and Hongjue Li. "Powered Landing Control of Reusable Rockets Based on Softmax Double DDPG." Aerospace 10, no. 7 (2023): 590.
[6]. Jin, Guangyin, Jincai Huang, Yanghe Feng, Guangquan Cheng, Zhong Liu, and Qi Wang. "Addressing the Task of Rocket Recycling with Deep Reinforcement Learning." In Proceedings of the 6th International Conference on Information Technology: IoT and Smart City, pp. 284-290. 2018.
[7]. Rodriguez, Juan. "Recyclable Rocket Modeling and Control in Complex Weather Conditions." (2023).
[8]. Zhengxia Zou (2021). Github Repository: Rocket-recycling with Reinforcement Learning. https://github.com/jiupinjia/rocket-recycling
[9]. Sebastian, R., Joshua, P., Corey, N., (2020). Machine Learning in Python: Main Developments and Technology Trends in Data Science, Machine Learning, and Artificial Intelligence. https://www.mdpi.com/2078-2489/11/4/193
[10]. Shin, Joohyun, Thomas A. Badgwell, Kuang-Hung Liu, and Jay H. Lee. "Reinforcement learning–overview of recent progress and implications for process control." Computers & Chemical Engineering 127 (2019): 282-294.
[11]. Li, Yuxi. "Deep reinforcement learning: An overview." arXiv preprint arXiv:1701.07274 (2017).
[12]. Han, Minghao, Lixian Zhang, Jun Wang, and Wei Pan. "Actor-critic reinforcement learning for control with stability guarantee." IEEE Robotics and Automation Letters 5, no. 4 (2020): 6217-6224.
Cite this article
Mou,A. (2024). Rocket Recycling via Reinforcement Learning. Applied and Computational Engineering,113,83-91.
Data availability
The datasets used and/or analyzed during the current study will be available from the authors upon reasonable request.
Disclaimer/Publisher's Note
The statements, opinions and data contained in all publications are solely those of the individual author(s) and contributor(s) and not of EWA Publishing and/or the editor(s). EWA Publishing and/or the editor(s) disclaim responsibility for any injury to people or property resulting from any ideas, methods, instructions or products referred to in the content.
About volume
Volume title: Proceedings of the 2nd International Conference on Machine Learning and Automation
© 2024 by the author(s). Licensee EWA Publishing, Oxford, UK. This article is an open access article distributed under the terms and
conditions of the Creative Commons Attribution (CC BY) license. Authors who
publish this series agree to the following terms:
1. Authors retain copyright and grant the series right of first publication with the work simultaneously licensed under a Creative Commons
Attribution License that allows others to share the work with an acknowledgment of the work's authorship and initial publication in this
series.
2. Authors are able to enter into separate, additional contractual arrangements for the non-exclusive distribution of the series's published
version of the work (e.g., post it to an institutional repository or publish it in a book), with an acknowledgment of its initial
publication in this series.
3. Authors are permitted and encouraged to post their work online (e.g., in institutional repositories or on their website) prior to and
during the submission process, as it can lead to productive exchanges, as well as earlier and greater citation of published work (See
Open access policy for details).