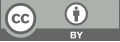
Big Data-Driven Analysis of Music Learning Behavior: Personalized Teaching Recommendations and Learning Strategy Optimization
- 1 Sichuan Conservatory of Music, Sichuan, China
* Author to whom correspondence should be addressed.
Abstract
This paper discusses how big data analytics could revolutionize music education by suggesting pedagogic ideas and shaping learning experiences based on student profiles. Through a combination of practice records, teacher-student dialogue and qualitative feedback, this study builds detailed portraits of music students to inform adaptive, data-informed teaching. It includes collecting, processing and analyzing the data, and applying clustering to classify the learner into Fast Learners, Methodical Learners and Emotionally Driven Learners. Each profile gets personalized practice schedules, feedback, and incentives for improved engagement and skill acquisition. Findings demonstrate that personalized methods deliver considerable improvements in learning and achievement. It also uses adaptive learning pathways and gamification to enhance student progress. Adapting to the new realities through feedback loops and constantly updated adjustments, this big data approach holds the promise of a fresh and flexible music education model. This study not only suggests how big data can drive personalized learning but offers a template for future educational data mining in music and beyond.
Keywords
Big Data, Music Learning, Personalized Teaching, Learning Strategy, Education Technology
[1]. Rajakumar, Keerthik Dhivya, Jagannath Mohan, and Adalarasu Kanagasabai. "An IoT-based emotion analysis and music therapy." Big Data, Cloud Computing and IoT. Chapman and Hall/CRC, 2023. 215-228.
[2]. Gorgoglione, Michele, et al. "Information Retrieval Technologies and Big Data Analytics to Analyze Product Innovation in the Music Industry." Sustainability 15.1 (2023): 828.
[3]. Le Coze, Jean-Christophe, and Stian Antonsen. "Safety in a digital age: Old and new problems—algorithms, machine learning, Big Data and artificial intelligence." Safety in the Digital Age: Sociotechnical Perspectives on Algorithms and Machine Learning. Cham: Springer Nature Switzerland, 2023. 1-9.
[4]. Shen, Xiuli. "Rapid Integration Algorithm for Music Education Information Resources Based on Data Mining." International Journal of High Speed Electronics and Systems (2024): 2540031.
[5]. Dewi, Christine, Abbott Po Shun Chen, and Henoch Juli Christanto. "Recognizing similar musical instruments with YOLO models." Big Data and Cognitive Computing 7.2 (2023): 94.
[6]. Arastoopour Irgens, Golnaz, et al. "Bop or flop?: Integrating music and data science in an elementary classroom." The Journal of Experimental Education 92.2 (2024): 262-286.
[7]. Obaid, Omar Ibrahim. "From machine learning to artificial general intelligence: A roadmap and implications." Mesopotamian Journal of Big Data 2023 (2023): 81-91.
[8]. Khonzoda, Ibragimova. "Methods of oriental scientists in forming music-rhythmic competencies in future music teachers." International Scientific and Current Research Conferences. 2024.
[9]. Hasan, Ferdous. "Pervasive Computing in Music Education: Creating an IoT-Connected Interactive Music Learning and Practice Platform."
[10]. Kommisetty, P. D. N. K., and Avula Nishanth. "AI-Driven Enhancements in Cloud Computing: Exploring the Synergies of Machine Learning and Generative AI." (2024).
[11]. Zeng, Dajun. "Intelligent Music Wireless Signal Multi-feature Evaluation System Based on ABE Algorithm." 2023 International Conference on Computer Simulation and Modeling, Information Security (CSMIS). IEEE, 2023.
Cite this article
Hou,X. (2024). Big Data-Driven Analysis of Music Learning Behavior: Personalized Teaching Recommendations and Learning Strategy Optimization. Applied and Computational Engineering,118,8-13.
Data availability
The datasets used and/or analyzed during the current study will be available from the authors upon reasonable request.
Disclaimer/Publisher's Note
The statements, opinions and data contained in all publications are solely those of the individual author(s) and contributor(s) and not of EWA Publishing and/or the editor(s). EWA Publishing and/or the editor(s) disclaim responsibility for any injury to people or property resulting from any ideas, methods, instructions or products referred to in the content.
About volume
Volume title: Proceedings of the 3rd International Conference on Software Engineering and Machine Learning
© 2024 by the author(s). Licensee EWA Publishing, Oxford, UK. This article is an open access article distributed under the terms and
conditions of the Creative Commons Attribution (CC BY) license. Authors who
publish this series agree to the following terms:
1. Authors retain copyright and grant the series right of first publication with the work simultaneously licensed under a Creative Commons
Attribution License that allows others to share the work with an acknowledgment of the work's authorship and initial publication in this
series.
2. Authors are able to enter into separate, additional contractual arrangements for the non-exclusive distribution of the series's published
version of the work (e.g., post it to an institutional repository or publish it in a book), with an acknowledgment of its initial
publication in this series.
3. Authors are permitted and encouraged to post their work online (e.g., in institutional repositories or on their website) prior to and
during the submission process, as it can lead to productive exchanges, as well as earlier and greater citation of published work (See
Open access policy for details).