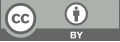
Optimization of XGBoost Bankruptcy Prediction Based on Four-Vector Optimization Algorithm
- 1 Department of Economics, University of California, Berkeley, State of California, Berkeley, 94704, USA
* Author to whom correspondence should be addressed.
Abstract
In this paper, the XGBoost model is optimized based on the four-vector optimization algorithm to realize the effective prediction of enterprise bankruptcy. In the experiment, we adopted this advanced algorithm to greatly improve the predictive performance of the model, with the goal of providing a reliable bankruptcy risk assessment tool for enterprises and investors. By training the model, we observed a gradual decline in the fitness curve from 0.029 to 0.026. This trend indicates that with the increase of the number of training iterations, the performance of the model is constantly improving. The gradual slowing of fitness decline suggests that the model may have approached the optimal solution or become stable, which provides confidence for subsequent applications. In terms of specific prediction effect, the confusion matrix of the training set shows that the accuracy of the model is as high as 100%, while the accuracy of the test set is as high as 97.33%. Such high accuracy not only reflects the model's excellent ability on the seen data, but also shows that the model has good generalization ability and can maintain efficient performance on the unseen data. In addition, the performance indicator chart shows the trend of different performance indicators, including FM, J, Q, CA, DAUC, SE, SP. From the figure, we can see that the values of FM and J remain around 1.5, indicating that the model performs well in some ways. However, DAUC and SE showed less than ideal results, close to zero or negative, which means that on some performance indicators, the model still needs further optimization and improvement. To sum up, the research in this paper not only provides an effective tool for corporate bankruptcy prediction, but also provides data support for investors and managers in the decision-making process. By accurately predicting the risk of bankruptcy, relevant parties can take preventive measures to effectively reduce investment risks and improve decision-making efficiency. Therefore, improving the accuracy of corporate bankruptcy prediction can not only promote the sustainable development of enterprises, but also provide protection for the healthy operation of the economy in a larger scope.
Keywords
Four-vector optimization algorithm, XGBoost, Enterprise Bankruptcy prediction
[1]. Charalambous, Chris, Spiros H. Martzoukos, and Zenon Taoushianis. "Estimating corporate bankruptcy forecasting models by maximizing discriminatory power." Review of Quantitative Finance and Accounting 58.1 (2022): 297-328.
[2]. Alam, Talha Mahboob, et al. "Corporate bankruptcy prediction: An approach towards better corporate world." The Computer Journal 64.11 (2021): 1731-1746.
[3]. Kim, Hyeongjun, Hoon Cho, and Doo** Ryu. "Corporate bankruptcy predi
[4]. Matenda, Frank Ranganai, et al. "Bankruptcy prediction for private firms in develo** economies: a sco** review and guidance for future research." Management Review Quarterly (2021): 1-40.
[5]. du Jardin, Philippe. "Designing topological data to forecast bankruptcy using convolutional neural networks." Annals of Operations Research 325.2 (2023): 1291-1332.
[6]. Cao, Yi, et al. "A two‐stage Bayesian network model for corporate bankruptcy prediction." International Journal of Finance & Economics 27.1 (2022): 455-472.
[7]. Chen, Tsung-Kang, et al. "Bankruptcy prediction using machine learning models with the text-based communicative value of annual reports." Expert Systems with Applications 233 (2023): 120714.
[8]. Jones, Stewart. "A literature survey of corporate failure prediction models." Journal of Accounting Literature 45.2 (2023): 364-405.
[9]. Vuković, Bojana, et al. "Corporate bankruptcy prediction: evidence from wholesale companies in the Western European countries." Ekonomicky casopis 68.5 (2020): 477-498.
[10]. Rajendran, Surendran, et al. "Automated segmentation of brain tumor MRI images using deep learning." IEEE Access 11 (2023): 64758-64768.
Cite this article
Jin,Y. (2024). Optimization of XGBoost Bankruptcy Prediction Based on Four-Vector Optimization Algorithm. Applied and Computational Engineering,120,42-49.
Data availability
The datasets used and/or analyzed during the current study will be available from the authors upon reasonable request.
Disclaimer/Publisher's Note
The statements, opinions and data contained in all publications are solely those of the individual author(s) and contributor(s) and not of EWA Publishing and/or the editor(s). EWA Publishing and/or the editor(s) disclaim responsibility for any injury to people or property resulting from any ideas, methods, instructions or products referred to in the content.
About volume
Volume title: Proceedings of the 5th International Conference on Signal Processing and Machine Learning
© 2024 by the author(s). Licensee EWA Publishing, Oxford, UK. This article is an open access article distributed under the terms and
conditions of the Creative Commons Attribution (CC BY) license. Authors who
publish this series agree to the following terms:
1. Authors retain copyright and grant the series right of first publication with the work simultaneously licensed under a Creative Commons
Attribution License that allows others to share the work with an acknowledgment of the work's authorship and initial publication in this
series.
2. Authors are able to enter into separate, additional contractual arrangements for the non-exclusive distribution of the series's published
version of the work (e.g., post it to an institutional repository or publish it in a book), with an acknowledgment of its initial
publication in this series.
3. Authors are permitted and encouraged to post their work online (e.g., in institutional repositories or on their website) prior to and
during the submission process, as it can lead to productive exchanges, as well as earlier and greater citation of published work (See
Open access policy for details).