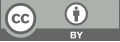
Exploring the Effectiveness of Big Data in Profitability of Construction Projects
- 1 University College London
* Author to whom correspondence should be addressed.
Abstract
The complexity of construction projects is generally high, requiring the collection and processing of data from diverse sources. Big data-based analytics and technologies can help such a project in different ways to improve profitability. However, their use comes with inherent risks, particularly concerning data security and accuracy. Data accuracy is a concern, as it directly impacts the credibility and reliability of Big Data analytics outcomes. The research examines the effectiveness of Big Data in the profitability of construction projects through extensive literature review and case analysis. Findings highlight that data accuracy is a key determinant of profitability in this context. Construction firms need to enforce policies and procedures to ensure data quality, credibility and accuracy while performing data collection. With accurate data, firms can leverage analytics to make informed, data-driven decisions that drive project success.
Keywords
Big Data analytics, construction projects, BIM, construction project profitability
[1]. Zhang, X. (2005). Financial viability analysis and capital structure optimization in privatized public infrastructure projects. Journal of construction engineering and management, 131(6), 656-668.
[2]. Xia, W., Zheng, Y., Huang, L., & Liu, Z. (2023). Integration of building information modeling (BIM) and big data in China: recent application and future perspective. Buildings, 13(10), 2435.
[3]. Castro Miranda, S. L., Del Rey Castillo, E., Gonzalez, V., & Adafin, J. (2022). Predictive analytics for early-stage construction cost estimation. Buildings, 12(7), 1043.
[4]. Zabala-Vargas, S., Jaimes-Quintanilla, M., & Jimenez-Barrera, M. H. (2023). Big data, data science, and artificial intelligence for project management in the architecture, engineering, and construction industry: a systematic review. Buildings, 13(12), 2944.
[5]. Munawar, H. S., Qayyum, S., Ullah, F., & Sepasgozar, S. (2020). Big data and its applications in smart real estate and the disaster management life cycle: A systematic analysis. Big Data and Cognitive Computing, 4(2), 4.
[6]. ilal, M., Oyedele, L. O., Kusimo, H. O., Owolabi, H. A., Akanbi, L. A., Ajayi, A. O., Akinade, O. O., & Delgado, J. M. D. (2019). Investigating profitability performance of construction projects using big data: A project analytics approach. Journal of Building Engineering, 26, 100850.
[7]. Yu, X., & Zuo, H. (2022). Research on Construction Cost Control Technology of Construction Project Based on Big Data Analysis Theory. Mobile Information Systems, 2022(1), 8739097.
[8]. Mandičák, T., Mésároš, P., Kanáliková, A., & Špak, M. (2021). Supply chain management and big data concept effects on economic sustainability of building design and project planning. Applied Sciences, 11(23), 11512.
[9]. Narayan, S., & Tan, H. C. (2019). Adopting big data to forecast success of construction projects: A review. Malaysian Construction Research Journal, 6(1), 132-143.
[10]. Munawar, H. S., Ullah, F., Qayyum, S., & Shahzad, D. (2022). Big data in construction: current applications and future opportunities. Big Data and Cognitive Computing, 6(1), 18.
[11]. Obiuto, N. C., Adebayo, R. A., Olajiga, O. K., & Festus-Ikhuoria, I. (2024). Integrating artificial intelligence in construction management: Improving project efficiency and cost-effectiveness. Int. J. Adv. Multidisc. Res. Stud, 4(2), 639-647.
[12]. Yang, J., Zhao, Y., Han, C., Liu, Y., & Yang, M. (2022). Big data, big challenges: risk management of financial market in the digital economy. Journal of Enterprise Information Management, 35(4/5), 1288-1304.
Cite this article
Yang,H. (2025). Exploring the Effectiveness of Big Data in Profitability of Construction Projects. Applied and Computational Engineering,120,209-213.
Data availability
The datasets used and/or analyzed during the current study will be available from the authors upon reasonable request.
Disclaimer/Publisher's Note
The statements, opinions and data contained in all publications are solely those of the individual author(s) and contributor(s) and not of EWA Publishing and/or the editor(s). EWA Publishing and/or the editor(s) disclaim responsibility for any injury to people or property resulting from any ideas, methods, instructions or products referred to in the content.
About volume
Volume title: Proceedings of the 5th International Conference on Signal Processing and Machine Learning
© 2024 by the author(s). Licensee EWA Publishing, Oxford, UK. This article is an open access article distributed under the terms and
conditions of the Creative Commons Attribution (CC BY) license. Authors who
publish this series agree to the following terms:
1. Authors retain copyright and grant the series right of first publication with the work simultaneously licensed under a Creative Commons
Attribution License that allows others to share the work with an acknowledgment of the work's authorship and initial publication in this
series.
2. Authors are able to enter into separate, additional contractual arrangements for the non-exclusive distribution of the series's published
version of the work (e.g., post it to an institutional repository or publish it in a book), with an acknowledgment of its initial
publication in this series.
3. Authors are permitted and encouraged to post their work online (e.g., in institutional repositories or on their website) prior to and
during the submission process, as it can lead to productive exchanges, as well as earlier and greater citation of published work (See
Open access policy for details).