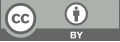
RS on video games based on item-based collaborative filtering algorithm
- 1 Chengdu University of Technology, Department of Software Engineering
* Author to whom correspondence should be addressed.
Abstract
With the rapid development of the Internet and e-commerce, recommender systems have received great attention and wide application in this environment. Because it is difficult for people to choose the one that they like in the face of the dazzling array of items on the Internet, and these e-commerce sites also need to consider how to improve efficiency, the recommendation system is an excellent solution. This paper mainly reviewed the development of recommender systems, focusing on the research and experiments of a recommender system based on an item-based collaborative filtering algorithm. According to the experimental results and some previous studies, summarizing the advantages and disadvantages of this method, proposing some solutions, and pointing out some problems that will be faced by future researches on recommendation systems.
Keywords
Recommendation system, Collaborative filtering, Item-based Collaborative filtering
[1]. Ren X L and Liu L 2008 Research progress and prospect of recommender system 2008 China Journal of Information Systems 2 p 82-90.
[2]. Wang Y F, Chuang Y L, Hsu M H and Keh H C 2004 A personalized recommender system for the cosmetic business Expert Systems with Applications 26 p 427-434
[3]. Liu M C 2016 Research on Content-Based Recommendation Technology Modern Marketing 6 p 243
[4]. Huang S L 2011 Designing utility-based recommender systems for e-commerce: Evaluation of preference-elicitation methods - ScienceDirect Electronic Commerce Research and Applications 10 p 398-407
[5]. Ma H G, Zhang G W and Li P 2009 Survey of Collaborative Filtering Algorithms Journal of Chinese Computer Systems 30(7) p 1282-1288.
[6]. Liu Z B 2016 Application of Machine Learning Methods in Personalized Recommender Systems Information Research 4
[7]. Huang L W, Jiang B T, Lv S Y, Liu Y B and Li D Y 2018 Survey on Deep Learning Based Recommender Systems Chinese Journal of Computers 41(7)
[8]. Sun X H 2005 Research on Sparsity and Cold Start Problem of Collaborative Filtering System (Doctoral dissertation: Zhejiang University)
[9]. Kim B M, Li Q, Kim J W and Kim J 2004 A new collaborative recommender system addressing three problems In Pacific Rim International Conference on Artificial Intelligence. (Berlin, Heidelberg: Springer) p 495-504
Cite this article
Zhu,H. (2023). RS on video games based on item-based collaborative filtering algorithm. Applied and Computational Engineering,5,11-17.
Data availability
The datasets used and/or analyzed during the current study will be available from the authors upon reasonable request.
Disclaimer/Publisher's Note
The statements, opinions and data contained in all publications are solely those of the individual author(s) and contributor(s) and not of EWA Publishing and/or the editor(s). EWA Publishing and/or the editor(s) disclaim responsibility for any injury to people or property resulting from any ideas, methods, instructions or products referred to in the content.
About volume
Volume title: Proceedings of the 3rd International Conference on Signal Processing and Machine Learning
© 2024 by the author(s). Licensee EWA Publishing, Oxford, UK. This article is an open access article distributed under the terms and
conditions of the Creative Commons Attribution (CC BY) license. Authors who
publish this series agree to the following terms:
1. Authors retain copyright and grant the series right of first publication with the work simultaneously licensed under a Creative Commons
Attribution License that allows others to share the work with an acknowledgment of the work's authorship and initial publication in this
series.
2. Authors are able to enter into separate, additional contractual arrangements for the non-exclusive distribution of the series's published
version of the work (e.g., post it to an institutional repository or publish it in a book), with an acknowledgment of its initial
publication in this series.
3. Authors are permitted and encouraged to post their work online (e.g., in institutional repositories or on their website) prior to and
during the submission process, as it can lead to productive exchanges, as well as earlier and greater citation of published work (See
Open access policy for details).