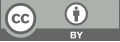
The Application of BCI Based on Deep Learning in Stroke Treatment
- 1 Department of Electronic and Electrical Engineering, Nouthern University of Science and Technology, Shenzhen, 518055, China
- 2 School of Electronic & Electrical Engineering, University of Leeds, Leeds, LS2 9JT, United Kingdom
- 3 Faculty of Natural, Mathematical & Engineering Sciences, Kings College London, London, WC2R 2LS, United Kingdom
- 4 Hangzhou World Foreign Language School, Hangzhou, 310000, China
* Author to whom correspondence should be addressed.
Abstract
Stroke is considered a global disease that leads to death and certain neural disabilities. Deep learning techniques have revolutionized EEG-based Brain-Computer Interface(BCI), enhancing reliability and promising a transformative future for BCI applications in stroke rehabilitation. However, BCI's implementation in clinical practice has been restricted due to their low accuracy performance. The objective of this review is to summarize how the integration of deep learning and BCI technologies can contribute to the rehabilitation of stroke patients. This paper compiles studies that evaluated deep learning and BCI intersection for stroke subjects, analyses the methodological quality of these studies, and verifies the relationship between the effects of the interventions and performance achieved in rehabilitation. The deficiencies and the future development direction of stroke rehabilitation in BCI with deep learning are also discussed. The various deep learning techniques combined with BCI technology, will improve people's ability to cope with stroke and provide a way to recover from stroke.
Keywords
brain computer interface, deep learning, stroke rehabilitation, motor imagery, stroke diagnoses
[1]. R.G. Nogueira, A.P. Jadhav, D.C. Haussen, et al., Thrombectomy 6 to 24 hours after stroke with a mismatch between deficit and infarct, N. Engl. J. Med. 378 (1) (2018) 11–21, https://doi.o rg/10.1056/NEJMoa1706442.
[2]. G.W. Albers, M.P. Marks, S. Kemp, et al., Thrombectomy for stroke at 6 to 16 hours with selection by perfusion imaging, N. Engl. J. Med. 378 (8) (2018) 708–718, https://doi.org/10.1056/NEJMoa1713973.
[3]. S. Al-Shoukry, T. H. Rassem and N. M. Makbol, “Alzheimer’s diseases detection by using deep learning algorithms: A mini-review,” IEEE Access, vol. 8, pp. 77131–77141, 2020.
[4]. Y. K. Cetinoglu, I. O. Koska, M. E. Uluc, and M. F. Gelal, "Detection and vascular territorial classification of stroke on diffusion-weighted MRI by deep learning," European Journal of Radiology, vol. 145, 2021, Art. no. 110050.
[5]. B. Tasci, "Automated ischemic acute infarction detection using pre-trained CNN models' deep features," Biomedical Signal Processing and Control, vol. 82, 2023, Art. no. 104603.
[6]. Z. G. Al-Mekhlafi, E. M. Senan, T. H. Rassem, B. A. Mohammed, N. M. Makbol, A. A. Alanazi, et al., "Deep learning and machine learning for early detection of stroke and haemorrhage," Computers, Materials and Continua, vol. 72, no. 1, pp. 775-796, 2022.
[7]. S. Singh, D. Dawar, E. Mehmood, J. D. Pandian, R. Sahonta, S. Singla, et al., "Determining Diagnostic Utility of EEG for Assessing Stroke Severity using Deep Learning Models," Biomedical Engineering Advances, vol. 7, 2024, Art. no. 100121.
[8]. J. Ma, et al., "Stroke Identification Based on EEG Convolutional Neural Network," in Intelligent Life System Modelling, Image Processing and Analysis (LSMSIS ICSEE 2021), M. Fei, L. Chen, S. Ma, and X. Li, eds., vol. 1467, Singapore: Springer, 2021, pp. 266-275.
[9]. R. Foong et al., "Assessment of the Efficacy of EEG-Based MI-BCI With Visual Feedback and EEG Correlates of Mental Fatigue for Upper-Limb Stroke Rehabilitation," IEEE Transactions on Biomedical Engineering, vol. 67, no. 3, pp. 786-794, March 2020.
[10]. C. Zhao, R. Li, C. Wang, W. Huang, and Y. Zhang, "EEG-Based Brain Network Analysis in Stroke Patients During a Motor Execution Task," in Proc. 9th Int. IEEE EMBSConf. Neural Eng., San Francisco, CA, USA, March 20-23, 2019, pp. 887-890
[11]. H. R. Yasin, E. C. Djamal, and F. Nugraha, "Optimization of Multi-Channel EEG Signal Using Genetic Algorithm in Post-Stroke Classification," in 2020 3rd International Conference on Computer and Informatics Engineering (IC2IE), Bali, Indonesia, 2020, pp. 129-134,
[12]. A. S. Ananda, E. C. Djamal, and F. Nugraha, "Post-Stroke Recognition Based on EEG Using PCA and Recurrent Neural Networks," 2020 3rd International Conference on Computer and Informatics Engineering (IC2IE), 2020, pp. 1-7,
[13]. C. Tangwiriyasakul, V. Mocioiu, M. J. A. M. van Putten, and W. L. C. Rutten, "Classification of motor imagery performance in acute stroke," J. Neural Eng., vol. 11, no. 3, pp. 1-9, 2014,
[14]. K. K. Ang and C. Guan, "EEG-Based Strategies to Detect Motor Imagery for Control and Rehabilitation," IEEE Transactions on Neural Systems and Rehabilitation Engineering, vol. 25, no. 4, pp. 392-401, April 2017,
[15]. H. Raza, A. Chowdhury, and S. Bhattacharyya, "Deep Learning based Prediction of EEG Motor Imagery of Stroke Patients for Neuro-Rehabilitation Application," in Proceedings of the International Joint Conference on Neural Networks (IJCNN), 2020, pp. 1-8.
[16]. S. Tortora, S. Ghidoni, C. Chisari, S. Micera, and F. Artoni, "Deep learning-based BCI for gait decoding from EEG with LSTM recurrent neural network," Journal of Neural Engineering, vol. 17, no. 4, pp. 046011, Jul. 2020,
[17]. T. Karácsony, J. P. Hansen, H. K. Iversen, and S. Puthusserypady, "Brain Computer Interface for Neuro-rehabilitation With Deep Learning Classification and Virtual Reality Feedback," in Proceedings of the Augmented Human International Conference 2019 (AH2019), Reims, France, 2019, pp. 1-8,
Cite this article
Lin,X.;Yang,C.;Yuan,W.;Cai,Z. (2025). The Application of BCI Based on Deep Learning in Stroke Treatment. Applied and Computational Engineering,131,1-7.
Data availability
The datasets used and/or analyzed during the current study will be available from the authors upon reasonable request.
Disclaimer/Publisher's Note
The statements, opinions and data contained in all publications are solely those of the individual author(s) and contributor(s) and not of EWA Publishing and/or the editor(s). EWA Publishing and/or the editor(s) disclaim responsibility for any injury to people or property resulting from any ideas, methods, instructions or products referred to in the content.
About volume
Volume title: Proceedings of the 2nd International Conference on Machine Learning and Automation
© 2024 by the author(s). Licensee EWA Publishing, Oxford, UK. This article is an open access article distributed under the terms and
conditions of the Creative Commons Attribution (CC BY) license. Authors who
publish this series agree to the following terms:
1. Authors retain copyright and grant the series right of first publication with the work simultaneously licensed under a Creative Commons
Attribution License that allows others to share the work with an acknowledgment of the work's authorship and initial publication in this
series.
2. Authors are able to enter into separate, additional contractual arrangements for the non-exclusive distribution of the series's published
version of the work (e.g., post it to an institutional repository or publish it in a book), with an acknowledgment of its initial
publication in this series.
3. Authors are permitted and encouraged to post their work online (e.g., in institutional repositories or on their website) prior to and
during the submission process, as it can lead to productive exchanges, as well as earlier and greater citation of published work (See
Open access policy for details).