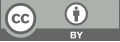
YOLOSCM: An Improved YOLO Algorithm for Cars Detection
- 1 School of Computer Science, Hubei University, Hubei, China
- 2 Columbia University in the City of New York, New York, USA
- 3 College of engineering, Northeastern University, Boston, USA
* Author to whom correspondence should be addressed.
Abstract
Detecting objects in urban traffic images presents considerable difficulties because of the following reasons: 1) These images are typically immense in size, encompassing millions or even hundreds of millions of pixels, yet computational resources are constrained. 2) The small size of vehicles in certain scenarios leads to insufficient information for accurate detection. 3) The uneven distribution of vehicles causes inefficient use of computational resources. To address these issues, we propose YOLOSCM (You Only Look Once with Segmentation Clustering Module), an efficient and effective framework. To address the challenges of large-scale images and the non-uniform distribution of vehicles, we propose a Segmentation Clustering Module (SCM). This module adaptively identifies clustered regions, enabling the model to focus on these areas for more precise detection. Additionally, we propose a new training strategy to optimize the detection of small vehicles and densely packed targets in complex urban traffic scenes. We perform extensive experiments on urban traffic datasets to demonstrate the effectiveness and superiority of our proposed approach.
Keywords
cars detection, small target, clustering
[1]. B. Singh and L. S. Davis, “An analysis of scale invariance in object detection snip,” in Proceedings of the IEEE conference on computer vision and pattern recognition, 2018, pp. 3578–3587.
[2]. C. Xu, J. Wang, W. Yang, H. Yu, L. Yu, and G.-S. Xia, “Detecting tiny objects in aerial images: A normalized wasserstein distance and a new benchmark,” ISPRS Journal of Photogrammetry and Remote Sensing, vol. 190, pp. 79–93, 2022.
[3]. F. Yang, H. Fan, P. Chu, E. Blasch, and H. Ling, “Clustered object detection in aerial images,” in Proceedings of the IEEE/CVF international conference on computer vision, 2019, pp. 8311–8320.
[4]. J. Gao, Y. Yuan, and Q. Wang, “Feature-aware adaptation and density alignment for crowd counting in video surveillance,”IEEE transactions on cybernetics, vol. 51, no. 10, pp. 4822–4833, 2020.
[5]. C. Li, T. Yang, S. Zhu, C. Chen, and S. Guan, “Density map guided object detection in aerial images,” in proceedings of the IEEE/CVF conference on computer vision and pattern recognition workshops, 2020, pp. 190–191.
[6]. H. Rezatofighi, N. Tsoi, J. Gwak, A. Sadeghian, I. Reid, and S. Savarese, “Generalized intersection over union: A metric and a loss for bounding box regression,” in Proceedings of the IEEE/CVF conference on computer vision and pattern recognition, 2019, pp. 658–666.
[7]. C. Liu, G. Gao, Z. Huang, Z. Hu, Q. Liu, and Y. Wang,“Yolc: You only look clusters for tiny object detection in aerial images,” IEEE Transactions on Intelligent Transportation Systems, 2024.
[8]. T.-Y. Lin, M. Maire, S. Belongie, J. Hays, P. Perona, D. Ra- manan, P. Doll´ar, and C. L. Zitnick, “Microsoft coco: Common objects in context,” in Computer Vision–ECCV 2014: 13th European Conference, Zurich, Switzerland, September 6-12, 2014, Proceedings, Part V 13. Springer, 2014, pp. 740–755.
[9]. D. Du, Y. Qi, H. Yu, Y. Yang, K. Duan, G. Li, W. Zhang, Q. Huang, and Q. Tian, “The unmanned aerial vehicle benchmark: Object detection and tracking,” in Proceedings of the European conference on computer vision (ECCV), 2018, pp. 370–386.
[10]. X. Zhou, D. Wang, and P. Kr¨ahenb¨uhl, “Objects as points,”arXiv preprint arXiv:1904.07850, 2019.
Cite this article
Deng,C.;Chen,L.;Liu,S. (2025). YOLOSCM: An Improved YOLO Algorithm for Cars Detection. Applied and Computational Engineering,108,182-187.
Data availability
The datasets used and/or analyzed during the current study will be available from the authors upon reasonable request.
Disclaimer/Publisher's Note
The statements, opinions and data contained in all publications are solely those of the individual author(s) and contributor(s) and not of EWA Publishing and/or the editor(s). EWA Publishing and/or the editor(s) disclaim responsibility for any injury to people or property resulting from any ideas, methods, instructions or products referred to in the content.
About volume
Volume title: Proceedings of the 5th International Conference on Signal Processing and Machine Learning
© 2024 by the author(s). Licensee EWA Publishing, Oxford, UK. This article is an open access article distributed under the terms and
conditions of the Creative Commons Attribution (CC BY) license. Authors who
publish this series agree to the following terms:
1. Authors retain copyright and grant the series right of first publication with the work simultaneously licensed under a Creative Commons
Attribution License that allows others to share the work with an acknowledgment of the work's authorship and initial publication in this
series.
2. Authors are able to enter into separate, additional contractual arrangements for the non-exclusive distribution of the series's published
version of the work (e.g., post it to an institutional repository or publish it in a book), with an acknowledgment of its initial
publication in this series.
3. Authors are permitted and encouraged to post their work online (e.g., in institutional repositories or on their website) prior to and
during the submission process, as it can lead to productive exchanges, as well as earlier and greater citation of published work (See
Open access policy for details).