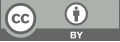
Elo in MOBA: Algorithm Comparison and Application Discussion
- 1 University of California Davis
- 2 Minzu University of China, Beijing, China
- 3 University of California Berkeley, Berkeley, United States
* Author to whom correspondence should be addressed.
Abstract
Online multiplayer games are supposed to match single players or squads with similar abilities. They depend on different kinds of rating systems to ensure a fair and competitive gaming environment. Traditional rating systems generally focus on single evaluation criteria, such as Elo scores or number of killings. However, games like Overwatch, which have more rating aspects, such as the usage of items and skills and the corporation of a whole team, need some more complex rating systems. Because of these, those traditional rating systems cannot satisfy the needs of modern multiplayer online games. Thus, it is urgent to develop new rating systems with new modes. In this paper, we are going to explore the newly designed Elo system with new models, especially its application in MOBA games. We will also talk about the TrueSkill and TrueSkill2 rating systems. These are two rating systems with high accuracy in win rate prediction, which can match fair games for players and teams.
Keywords
Elo, MOBA, Algorithm Comparison. Online multiplayer games
[1]. Ralf Herbrich, Tom Minka, and Thore Graepel. “TrueSkill™: a Bayesian skill rating system”. In: Advances in neural information processing systems 19 (2006).
[2]. Stephanie Kovalchik. “Extension of the Elo rating system to margin of victory”. In: International Journal of Forecasting 36.4 (2020), pp. 1329–1341.
[3]. Tom Minka, Ryan Cleven, and Yordan Zaykov. “True skill 2: An improved Bayesian skill rating system”. In: Technical Report (2018).
[4]. Yuhan Song. “Analysis of ELO rating scheme in MOBA games”. In: arXiv preprint arXiv:2310.13719 (2023).
[5]. Kai Wang et al. “EnMatch: Matchmaking for Better Player Engagement via Neural Combinatorial Optimization.” In: Proceedings of the AAAI Conference on Artificial Intelligence. Vol. 38. 8. 2024, pp. 9098–9106.
Cite this article
Dong,J.;Jiao,H.;Liu,Y. (2025). Elo in MOBA: Algorithm Comparison and Application Discussion. Applied and Computational Engineering,132,292-300.
Data availability
The datasets used and/or analyzed during the current study will be available from the authors upon reasonable request.
Disclaimer/Publisher's Note
The statements, opinions and data contained in all publications are solely those of the individual author(s) and contributor(s) and not of EWA Publishing and/or the editor(s). EWA Publishing and/or the editor(s) disclaim responsibility for any injury to people or property resulting from any ideas, methods, instructions or products referred to in the content.
About volume
Volume title: Proceedings of the 2nd International Conference on Machine Learning and Automation
© 2024 by the author(s). Licensee EWA Publishing, Oxford, UK. This article is an open access article distributed under the terms and
conditions of the Creative Commons Attribution (CC BY) license. Authors who
publish this series agree to the following terms:
1. Authors retain copyright and grant the series right of first publication with the work simultaneously licensed under a Creative Commons
Attribution License that allows others to share the work with an acknowledgment of the work's authorship and initial publication in this
series.
2. Authors are able to enter into separate, additional contractual arrangements for the non-exclusive distribution of the series's published
version of the work (e.g., post it to an institutional repository or publish it in a book), with an acknowledgment of its initial
publication in this series.
3. Authors are permitted and encouraged to post their work online (e.g., in institutional repositories or on their website) prior to and
during the submission process, as it can lead to productive exchanges, as well as earlier and greater citation of published work (See
Open access policy for details).