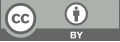
Movement Detection of Tennis Players Based on Yolo and Human Skeleton Recognition Technology
- 1 Maynooth International Engineering College, Fuzhou University
- 2 School of Beijing Foreign Study, University International Curriculum Center
- 3 School of Beijing Foreign Study, University International Curriculum Center
* Author to whom correspondence should be addressed.
Abstract
This study aims to develop a monitoring model for the action states of athletes (such as standing, moving, and striking) during tennis matches. The model is based on the YOLO (You Only Look Once) architecture and is trained with the 3dResNet50 to achieve automatic recognition of athletes’ actions. Additionally, we utilized the MediaPipe model to recognize the human skeletal structure, further enhancing the accuracy of action recognition. Tested on real tennis match video data, the model demonstrated efficient action recognition capabilities and good real-time performance, providing strong technical support for sports training and competition analysis. This research not only extends the application of computer vision in the field of sports but also lays a foundation for further advancements in motion analysis technology.
Keywords
Behavior Recognition, YOLO, 3DResNet50, Media-pipe, STGCN model, Frame Compare
[1]. Joseph Redmon, Santosh Divvala, Ross Girshick, and Ali Farhadi. You only look once: Unified, real-time object detection. In Proceedings of the IEEE Conference on Computer Vision and Pattern Recognition, pages 779–788, 2016.
[2]. Juan A. Vega-Márquez, Mikel Martínez-Otzeta, Aitor Gómez-Arriola, and Víctor Hernández-Herrero. Semisupervised deep learning for soccer ball detection and tracking. Sensors, 23(9):4688, 2023.
[3]. Xiaofei Li, Ronghua Luo, and Faiz Ul Islam. Tracking and detection of basketball movements using multi-feature data fusion and hybrid yolo-t2lstm network. Soft Computing, 28:1653–1667, 2024.
[4]. Kaiming He, Xiangyu Zhang, Shaoqing Ren, and Jian Sun. Deep residual learning for image recognition. Proceedings of the IEEE Conference on Computer Vision and Pattern Recognition, pages 770–778, 2016.
[5]. Jochen Görtler, Fred Hohman, Dominik Moritz, Kanit Wongsuphasawat, Donghao Ren, Rahul Nair, Marc Kirchner, and Kayur Patel. Neo: Generalizing confusion matrix visualization to hierarchical and multi-output labels. In CHI Conference on Human Factors in Computing Systems, pages 1–13. ACM, 2022.
[6]. Du Tran, Lubomir Bourdev, Rob Fergus, Lorenzo Torresani, and Manohar Paluri. Learning spatiotemporal features with 3d convolutional networks for action recognition in videos. In ICCV, 2015.
[7]. Andrew C Wilson, Rebecca Roelofs, Mitchell Stern, Nathan Srebro, and Benjamin Recht. On empirical comparisons of optimizers for deep learning. arXiv preprint arXiv:1710.06451, 2017.
[8]. Yu-Chuan Huang, I-No Liao, Ching-Hsuan Chen, Tsì-Uí Ik, and Wen-Chih Peng. Tracknet: A deep learning network for concurrent ball tracking and pose estimation during sports events. In Proceedings of the IEEE Conference on Computer Vision and Pattern Recognition Workshops, pages 4610–4619, 2018.
[9]. Xingkui Zhu, Shuchang Lyu, Xu Wang, and Qi Zhao. Tph-yolov5: Improved yolov5 based on transformer prediction head for object detection on drone-captured scenarios. arXiv preprint arXiv:2204.08621, 2022.
Cite this article
He,M.;Fu,M.;Cao,D. (2025). Movement Detection of Tennis Players Based on Yolo and Human Skeleton Recognition Technology. Applied and Computational Engineering,108,100-107.
Data availability
The datasets used and/or analyzed during the current study will be available from the authors upon reasonable request.
Disclaimer/Publisher's Note
The statements, opinions and data contained in all publications are solely those of the individual author(s) and contributor(s) and not of EWA Publishing and/or the editor(s). EWA Publishing and/or the editor(s) disclaim responsibility for any injury to people or property resulting from any ideas, methods, instructions or products referred to in the content.
About volume
Volume title: Proceedings of the 5th International Conference on Signal Processing and Machine Learning
© 2024 by the author(s). Licensee EWA Publishing, Oxford, UK. This article is an open access article distributed under the terms and
conditions of the Creative Commons Attribution (CC BY) license. Authors who
publish this series agree to the following terms:
1. Authors retain copyright and grant the series right of first publication with the work simultaneously licensed under a Creative Commons
Attribution License that allows others to share the work with an acknowledgment of the work's authorship and initial publication in this
series.
2. Authors are able to enter into separate, additional contractual arrangements for the non-exclusive distribution of the series's published
version of the work (e.g., post it to an institutional repository or publish it in a book), with an acknowledgment of its initial
publication in this series.
3. Authors are permitted and encouraged to post their work online (e.g., in institutional repositories or on their website) prior to and
during the submission process, as it can lead to productive exchanges, as well as earlier and greater citation of published work (See
Open access policy for details).