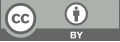
Performance comparison of representative chatbots based on deep learning
- 1 356 Alexandra Road, ALEXIS, 159949, Singapore
* Author to whom correspondence should be addressed.
Abstract
Chatbots have always been a hot topic in the field of natural language processing, which aims to train models to understand input content and give cognitively logical answers. With the continuous development of artificial intelligence technology, the performance of chat robots has continuously made breakthroughs, and has been widely used in many fields such as smart home, e-commerce, and automatic consultation. In this article, we introduce the basic techniques and methods of deep learning-based chatbots, including recurrent neural network (RNN), long short-term memory (LSTM), Seq2Seq model, attention mechanism, etc. We also review a chatbot designed using RNN and quality testing result. We finally summarize the existing research issues of the chatbot and discuss its possible future works.
Keywords
Deep learning, Chatbot, Natural language processing, Recurrent Neural Network, Long Short-Term Memory
[1]. Jurafsky, D., & Martin, J. H. Speech and Language Processing: An Introduction to Natural Language Processing, Computational Linguistics, and Speech Recognition.
[2]. Joshi, C. K., Mi, F., & Faltings, B. (2017). Personalization in goal-oriented dialog. arXiv preprint arXiv:1706.07503.
[3]. Weizenbaum, J. (1966). ELIZA—a computer program for the study of natural language communication between man and machine. Communications of the ACM, 9(1), 36-45.
[4]. Gilbert, R. L., & Forney, A. (2015). Can avatars pass the Turing test? Intelligent agent perception in a 3D virtual environment. International Journal of Human-Computer Studies, 73, 30-36.
[5]. Csaky, R. (2019). Deep learning based chatbot models. arXiv preprint arXiv:1908.08835.
[6]. Hochreiter, S., & Schmidhuber, J. (1997). Long short-term memory. Neural computation, 9(8), 1735-1780.
[7]. Sutskever, I., Vinyals, O., & Le, Q. V. (2014). Sequence to sequence learning with neural networks. Advances in neural information processing systems, 27.
[8]. Bahdanau, D., Cho, K., & Bengio, Y. (2014). Neural machine translation by jointly learning to align and translate. arXiv preprint arXiv:1409.0473.
[9]. Top. [Online] Available: http://file.rszhang.top/nlp/chat.zip
[10]. Radziwill, N. M., & Benton, M. C. (2017). Evaluating quality of chatbots and intelligent conversational agents. arXiv preprint arXiv:1704.04579.
[11]. ChatBot. [Online]. Available: https://github.com/1033020837/ChatBot
[12]. Why GitHub? [Online]. Available: https://github.com/wudejian789/Attention-Seq2Seq-Chatbot-by-Pytorch1.0.1
Cite this article
Song,C. (2023). Performance comparison of representative chatbots based on deep learning. Applied and Computational Engineering,6,710-718.
Data availability
The datasets used and/or analyzed during the current study will be available from the authors upon reasonable request.
Disclaimer/Publisher's Note
The statements, opinions and data contained in all publications are solely those of the individual author(s) and contributor(s) and not of EWA Publishing and/or the editor(s). EWA Publishing and/or the editor(s) disclaim responsibility for any injury to people or property resulting from any ideas, methods, instructions or products referred to in the content.
About volume
Volume title: Proceedings of the 3rd International Conference on Signal Processing and Machine Learning
© 2024 by the author(s). Licensee EWA Publishing, Oxford, UK. This article is an open access article distributed under the terms and
conditions of the Creative Commons Attribution (CC BY) license. Authors who
publish this series agree to the following terms:
1. Authors retain copyright and grant the series right of first publication with the work simultaneously licensed under a Creative Commons
Attribution License that allows others to share the work with an acknowledgment of the work's authorship and initial publication in this
series.
2. Authors are able to enter into separate, additional contractual arrangements for the non-exclusive distribution of the series's published
version of the work (e.g., post it to an institutional repository or publish it in a book), with an acknowledgment of its initial
publication in this series.
3. Authors are permitted and encouraged to post their work online (e.g., in institutional repositories or on their website) prior to and
during the submission process, as it can lead to productive exchanges, as well as earlier and greater citation of published work (See
Open access policy for details).