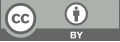
Cognitive Biases in Large Language Model based Decision Making: Insights and Mitigation Strategies
- 1 Emory University, 801 Emory St, Oxford, GA 30054, U.S.A
* Author to whom correspondence should be addressed.
Abstract
Recent development of Artificial Intelligence (AI) has led to an increasing integration of Large Language Models (LLMs) for real-world decision-making. While these models can enhance decision-making processes, they remain susceptible to the same cognitive biases found in humans. Our study examines two well-documented biases found in LLMs–Anchoring and Framing effect–and evaluates their presence in OpenAI’s GPT-3.5 turbo, GPT-4o, and GPT-4o-mini. Using a structured framework, we assess the degree of these biases across different models and proposed four bias mitigation prompting strategies: Chain-of-Thought (CoT), Thoughts of Principles (ToP), ignoring anchor hints, and reflection. We evaluated the bias reduction effectiveness of each prompting strategy using both our proposed prompts and GPT-generated prompts. Our findings reveal significant differences in bias levels across models (p < 0.01), with stronger models exhibiting a greater susceptibility to bias. However, none of the tested prompting strategies indicated statistically significant effectiveness in bias reduction. Our results highlight the challenges of debiasing LLMs and underscore the need for caution when deploying them in real-world decision-making scenarios. Further research is necessary to explore broader cognitive biases and develop more effective mitigation strategies.
Keywords
Cognitive biases, Anchoring bias, Framing Effect, Large Language Models, Bias Mitigation Strategies
[1]. Y. Yao, J. Duan, K. Xu, Y. Cai, Z. Sun, and Y. Zhang, “A survey on large language model (LLM) security and privacy: The Good, The Bad, and The Ugly,” High-Confid. Comput., vol. 4, no. 2, p. 100211, Jun. 2024, doi: 10.1016/j.hcc.2024.100211.
[2]. J. Kaddour, J. Harris, M. Mozes, H. Bradley, R. Raileanu, and R. McHardy, “Challenges and Applications of Large Language Models,” Jul. 19, 2023, arXiv: arXiv:2307.10169. doi: 10.48550/arXiv.2307.10169.
[3]. A. N. Talboy, and E. Fuller. “Challenging the Appearance of Machine Intelligence: Cognitive Bias in LLMs and Best Practices for Adoption.” Aug. 28, 2023. arXiv:2304.01358.
[4]. S. Malberg, R. Poletukhin, C. M. Schuster, and G. Groh, “A Comprehensive Evaluation of Cognitive Biases in LLMs,” Oct. 20, 2024, arXiv: arXiv:2410.15413. doi: 10.48550/arXiv.2410.15413.
[5]. Y. Zhang et al., “Siren’s Song in the AI Ocean: A Survey on Hallucination in Large Language Models,” Sep. 24, 2023, arXiv: arXiv:2309.01219. doi: 10.48550/arXiv.2309.01219.
[6]. “Measuring and Mitigating Racial Disparities in Large Language Model Mortgage Underwriting”.
[7]. J. Lou and Y. Sun, “Anchoring Bias in Large Language Models: An Experimental Study,” Dec. 18, 2024, arXiv: arXiv:2412.06593. doi: 10.48550/arXiv.2412.06593.
[8]. A. Tversky and D. Kahneman, “Judgment under Uncertainty: Heuristics and Biases,” vol. 185, 1974.
[9]. G. Suri, L. R. Slater, A. Ziaee, and M. Nguyen, “Do Large Language Models Show Decision Heuristics Similar to Humans?”.
[10]. J. K. Nguyen, “Human bias in AI models? Anchoring effects and mitigation strategies in large language models,” J. Behav. Exp. Finance, vol. 43, p. 100971, Sep. 2024, doi: 10.1016/j.jbef.2024.100971.
[11]. A. Tversky and D. Kahneman, “The Framing of Decisions and the Psychology of Choice,” vol. 211, 1981.
[12]. J. Gong, Y. Zhang, Z. Yang, Y. Huang, J. Feng, and W. Zhang, “The framing effect in medical decision-making: a review of the literature,” Psychol. Health Med., vol. 18, no. 6, pp. 645–653, 2013, doi: 10.1080/13548506.2013.766352.
[13]. B. Keysar, S. L. Hayakawa, and S. G. An, “The Foreign-Language Effect: Thinking in a Foreign Tongue Reduces Decision Biases,” Psychol. Sci., vol. 23, no. 6, pp. 661–668, Jun. 2012, doi: 10.1177/0956797611432178.
[14]. J. M. Echterhoff, Y. Liu, A. Alessa, J. McAuley, and Z. He, “Cognitive Bias in Decision-Making with LLMs,” in Findings of the Association for Computational Linguistics: EMNLP 2024, Miami, Florida, USA: Association for Computational Linguistics, 2024, pp. 12640–12653. doi: 10.18653/v1/2024.findings-emnlp.739.
Cite this article
Chen,S. (2025). Cognitive Biases in Large Language Model based Decision Making: Insights and Mitigation Strategies. Applied and Computational Engineering,138,167-174.
Data availability
The datasets used and/or analyzed during the current study will be available from the authors upon reasonable request.
Disclaimer/Publisher's Note
The statements, opinions and data contained in all publications are solely those of the individual author(s) and contributor(s) and not of EWA Publishing and/or the editor(s). EWA Publishing and/or the editor(s) disclaim responsibility for any injury to people or property resulting from any ideas, methods, instructions or products referred to in the content.
About volume
Volume title: Proceedings of the 3rd International Conference on Software Engineering and Machine Learning
© 2024 by the author(s). Licensee EWA Publishing, Oxford, UK. This article is an open access article distributed under the terms and
conditions of the Creative Commons Attribution (CC BY) license. Authors who
publish this series agree to the following terms:
1. Authors retain copyright and grant the series right of first publication with the work simultaneously licensed under a Creative Commons
Attribution License that allows others to share the work with an acknowledgment of the work's authorship and initial publication in this
series.
2. Authors are able to enter into separate, additional contractual arrangements for the non-exclusive distribution of the series's published
version of the work (e.g., post it to an institutional repository or publish it in a book), with an acknowledgment of its initial
publication in this series.
3. Authors are permitted and encouraged to post their work online (e.g., in institutional repositories or on their website) prior to and
during the submission process, as it can lead to productive exchanges, as well as earlier and greater citation of published work (See
Open access policy for details).