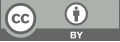
The Advantage and Disadvantage of Development of Quantum Computing in Machine Learning
- 1 College of Science, Mathematics and Technology, Wenzhou-Kean University, Wenzhou, Zhejiang Province, China, 325000
* Author to whom correspondence should be addressed.
Abstract
Quantum computing is an emergent technology with great promise to reshape machine learning. It enables computations intractable by any classical system. This paper discusses the basic concepts of quantum computing, its advantages, and their limitations regarding ML. It can be concluded that quantum computers leverage phenomena in quantum mechanics, such as superposition, entanglement, and interference execute those computations that are beyond the capability of classical systems. A few of the most important fancies in QSVM, QPCA, and QNN perform such tasks as computing kernel matrices, high-dimensional data processing, and solving optimization problems in some key points. In contrast, several formidable challenges persist, beginning with the scalability and coherence of qubits as hardware-related issues, and extending to software-centric problems such as data encoding and the efficiency of specific algorithmic applications. This paper also underscores potential future avenues for advancement, including the development of hybrid quantum-classical algorithms, quantum error correction techniques, and quantum-native machine learning models, aimed at surmounting these limitations. By addressing these challenges and directing focus towards prospective research, quantum computing holds the promise of unlocking transformative breakthroughs in various domains such as big data processing, drug development, financial modeling, and natural language processing. Such advancements could firmly establish quantum computing as the cornerstone of next-generation artificial intelligence technologies.
Keywords
quantum computing, machine learning, quantum machine learning, quantum algorithms
[1]. Long Haiquan, Liu Zhuoyue, and Wang Xiangtuan (2024). Research on the impact of the rapid development of artificial intelligence on computing power and energy China Informatization (11), 7-8
[2]. The physical implementation of quantum computation. (2000b). ADS. https://doi.org/10.1002/1521-3978(200009)48:9/11
[3]. Wang, Q. (2022, June). Support vector machine algorithm in machine learning. In 2022 IEEE international conference on artificial intelligence and computer applications (ICAICA) (pp. 750-756). IEEE.
[4]. Zhou, X., Yu, J., Tan, J., & Jiang, T. (2024). Quantum kernel estimation-based quantum support vector regression. Quantum Information Processing, 23(1). https://doi.org/10.1007/s11128-023-04231-7
[5]. Mandelbaum, R., Corcoles, A. D., & Gambetta, J. (2024, September 11). IBM’s Big Bet on the Quantum-Centric Supercomputer. IEEE Spectrum. https://spectrum.ieee.org/ibm-quantum-computer-2668978269
[6]. Zaman, A., Morrell, H. J., & Wong, H. Y. (2023). A Step-by-Step HHL algorithm walkthrough to enhance understanding of critical quantum computing concepts. IEEE Access, 11, 77117–77131. https://doi.org/10.1109/access.2023.3297658
Cite this article
Zhao,R. (2025). The Advantage and Disadvantage of Development of Quantum Computing in Machine Learning. Applied and Computational Engineering,119,167-172.
Data availability
The datasets used and/or analyzed during the current study will be available from the authors upon reasonable request.
Disclaimer/Publisher's Note
The statements, opinions and data contained in all publications are solely those of the individual author(s) and contributor(s) and not of EWA Publishing and/or the editor(s). EWA Publishing and/or the editor(s) disclaim responsibility for any injury to people or property resulting from any ideas, methods, instructions or products referred to in the content.
About volume
Volume title: Proceedings of the 3rd International Conference on Software Engineering and Machine Learning
© 2024 by the author(s). Licensee EWA Publishing, Oxford, UK. This article is an open access article distributed under the terms and
conditions of the Creative Commons Attribution (CC BY) license. Authors who
publish this series agree to the following terms:
1. Authors retain copyright and grant the series right of first publication with the work simultaneously licensed under a Creative Commons
Attribution License that allows others to share the work with an acknowledgment of the work's authorship and initial publication in this
series.
2. Authors are able to enter into separate, additional contractual arrangements for the non-exclusive distribution of the series's published
version of the work (e.g., post it to an institutional repository or publish it in a book), with an acknowledgment of its initial
publication in this series.
3. Authors are permitted and encouraged to post their work online (e.g., in institutional repositories or on their website) prior to and
during the submission process, as it can lead to productive exchanges, as well as earlier and greater citation of published work (See
Open access policy for details).