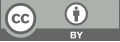
Traffic Flow Prediction Model Based on the Fusion of Timedomain Convolutional Network and Long- and Short-term Memory Network
- 1 School of Economics and Management, Chongqing Jiaotong University, Chongqing
* Author to whom correspondence should be addressed.
Abstract
This paper proposes a traffic flow prediction model based on the fusion of time-domain convolutional network (TCN) and long-short-term memory network (LSTM), and verifies its effectiveness through multi-dimensional experiments. To address the complexity of urban traffic flow prediction, the study constructs a TCN-LSTM hybrid model to fuse temporal feature extraction and long and short-term dependency capturing capabilities, and performs prediction validation on three types of traffic flow datasets, namely, cars, bicycles and trucks, respectively. The experimental results show that: in car traffic prediction, the training loss value of the model decreases significantly from the initial 0.8 to less than 0.1, and the R² of the training set and the test set reaches 0.73 and 0.75, respectively, which reflects good convergence and generalisation ability; bicycle traffic prediction shows that the R² of the training set reaches as high as 0.84 but the R² of the test set decreases to 0.31, which shows that there is a certain degree of overfitting phenomenon; truck Truck traffic prediction achieves a balanced performance of R² 0.73 for the training set and R² 0.50 for the test set, which verifies the model's ability to capture heavy vehicle traffic patterns robustly. By comparing the performance differences between TCN, LSTM and their hybrid models through ablation experiments, it is found that TCN-LSTM is superior in key indicators: the mean absolute error (MAE) is 0.11 lower than that of the pure TCN model, the mean squared error (MSE) is in between that of TCN and LSTM, and the relative prediction deviation (RPD) reaches the highest value of the three at 2.15, which is a good proof that the hybrid model combines both multi-scale capturing ability of time-series features and the advantage of accurate modelling of nonlinear relationships.
Keywords
Time-domain convolutional networks, Long and short-term memory networks, Traffic flow prediction
[1]. Pandey, Ashutosh, and DeLiang Wang. "TCNN: Temporal convolutional neural network for real-time speech enhancement in the time domain." ICASSP 2019-2019 IEEE International Conference on Acoustics, Speech and Signal Processing (ICASSP). IEEE, 2019.
[2]. Hou, Changbo, et al. "Multisignal modulation classification using sliding window detection and complex convolutional network in frequency domain." IEEE Internet of Things Journal 9.19 (2022): 19438-19449.
[3]. Teixeira, F. L., et al. "Finite-difference time-domain methods." Nature Reviews Methods Primers 3.1 (2023): 75.
[4]. Bai, Ruxue, et al. "Fractional Fourier and time domain recurrence plot fusion combining convolutional neural network for bearing fault diagnosis under variable working conditions." Reliability Engineering & System Safety 232 (2023): 109076.
[5]. Monsalves, N., et al. "Application of Convolutional Neural Networks to time domain astrophysics. 2D image analysis of OGLE light curves." Astronomy & Astrophysics 691 (2024): A106.
[6]. Liang, Pengfei, et al. "Unsupervised fault diagnosis of wind turbine bearing via a deep residual deformable convolution network based on subdomain adaptation under time-varying speeds." Engineering Applications of Artificial Intelligence 118 (2023): 105656.
[7]. Hou, Xiaoqi, and Yong Gao. "Single-channel blind separation of co-frequency signals based on convolutional network." Digital Signal Processing 129 (2022): 103654.
[8]. Shaaban, Ahmed, et al. "RT-SCNNs: real-time spiking convolutional neural networks for a novel hand gesture recognition using time-domain mm-wave radar data." International Journal of Microwave and Wireless Technologies 16.5 (2024): 783-795.
[9]. Qiu, Haobo, et al. "A piecewise method for bearing remaining useful life estimation using temporal convolutional networks." Journal of Manufacturing Systems 68 (2023): 227-241.
[10]. Tsaih, Rua-Huan, and Chih Chun Hsu. "Artificial intelligence in smart tourism: A conceptual framework." (2018)..
Cite this article
Liu,Y. (2025). Traffic Flow Prediction Model Based on the Fusion of Timedomain Convolutional Network and Long- and Short-term Memory Network. Applied and Computational Engineering,144,59-68.
Data availability
The datasets used and/or analyzed during the current study will be available from the authors upon reasonable request.
Disclaimer/Publisher's Note
The statements, opinions and data contained in all publications are solely those of the individual author(s) and contributor(s) and not of EWA Publishing and/or the editor(s). EWA Publishing and/or the editor(s) disclaim responsibility for any injury to people or property resulting from any ideas, methods, instructions or products referred to in the content.
About volume
Volume title: Proceedings of the 3rd International Conference on Functional Materials and Civil Engineering
© 2024 by the author(s). Licensee EWA Publishing, Oxford, UK. This article is an open access article distributed under the terms and
conditions of the Creative Commons Attribution (CC BY) license. Authors who
publish this series agree to the following terms:
1. Authors retain copyright and grant the series right of first publication with the work simultaneously licensed under a Creative Commons
Attribution License that allows others to share the work with an acknowledgment of the work's authorship and initial publication in this
series.
2. Authors are able to enter into separate, additional contractual arrangements for the non-exclusive distribution of the series's published
version of the work (e.g., post it to an institutional repository or publish it in a book), with an acknowledgment of its initial
publication in this series.
3. Authors are permitted and encouraged to post their work online (e.g., in institutional repositories or on their website) prior to and
during the submission process, as it can lead to productive exchanges, as well as earlier and greater citation of published work (See
Open access policy for details).