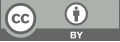
Computer vision model’s application in the current system on object detection tasks
- 1 Georgia Institute of Technology, North Ave NW, Atlanta, GA 30332
* Author to whom correspondence should be addressed.
Abstract
The implementation of object detection algorithms would be helpful to the various fields of the current time. When object detection is applied to the surveillance camera system, it will be more efficient to locate crimes or find lost kids. This paper will investigate the performance of different object detection algorithms in a real-world scenario. With experimentation, CenterNet++ outperforms YOLO and MaskRCNN, two traditional and classic object detection algorithms, on the MS COCO dataset, which concludes that CenterNet++ can ensure both accuracy and speed.
Keywords
Video Processing Systems, Computer Vision, Artificial Intelligence, Object Detection
[1]. Sreenu, G., Saleem Durai, M.A.2019 Intelligent video surveillance: a review through deep learning techniques for crowd analysis. J Big Data 6, 48.
[2]. Whittaker, Danielle.2021 “Why AI CCTV Is the Future of Security and Surveillance in Public Spaces.” Security, 14 Dec. 2021.
[3]. Tsakanikas, Vassilios, and Tasos Dagiuklas.2018 “Video Surveillance Systems-Current Status and Future Trends.” Computers & Electrical Engineering, vol. 70, pp. 736–753.
[4]. Arunnehru, J., et al. “Human Action Recognition Using 3D Convolutional Neural Networks with 3D Motion Cuboids in Surveillance Videos.” Procedia Computer Science, vol. 133, 2018, pp. 471–477.
[5]. D., Aishwarya, and Minu R.I. 2021 “Edge Computing Based Surveillance Framework for Real Time Activity Recognition.” ICT Express, vol. 7, no. 2, 2021, pp. 182–186.
[6]. Yuan, Yuan, et al. 2018“Action Recognition Using Spatial-Optical Data Organization and Sequential Learning Framework.” Neurocomputing, vol. 315, 2018, pp. 221–233.
[7]. Kardas, Karani, and Nihan Kesim Cicekli.2017 “SVAS: Surveillance Video Analysis System.” Expert Systems with Applications, vol. 89, 2017, pp. 343–361.
[8]. Wang, Dong, et al.2018 “Dairy Goat Detection Based on Faster R-CNN from Surveillance Video.” Computers and Electronics in Agriculture, vol. 154, 2018, pp. 443–449.
[9]. Beyer, Lucas & Zhai, Xiaohua & Royer, Amélie & Markeeva, Larisa & Anil, Rohan & Kolesnikov, Alexander. 2021. Knowledge distillation: A good teacher is patient and consistent.
[10]. Wei, Chen & Fan, Haoqi & Xie, Saining & Wu, Chao-Yuan & Yuille, Alan & Feichtenhofer, Christoph. 2021. Masked Feature Prediction for Self-Supervised Visual Pre-Training.
[11]. Lin, Tsung-Yi & Maire, Michael & Belongie, Serge & Hays, James & Perona, Pietro & Ramanan, Deva & Dollár, Piotr & Zitnick, C. 2014. Microsoft COCO: Common Objects in Context. 8693.
[12]. Lin TY, Maire M, Belongie S, et al. 2014 Microsoft coco: Common objects in context. In: European conference on computer vision, Springer, pp 740–755
[13]. Duan, Kaiwen & Bai, Song & Xie, Lingxi & Qi, Honggang & Tian, Qi. 2022 CenterNet++ for Object Detection.
[14]. Xingyi Zhou, Dequan Wang, Philipp Krähenbühl. Objects as Points arXiv preprint arXiv:1904.07850
[15]. Redmon, Joseph & Divvala, Santosh & Girshick, Ross & Farhadi, Ali. 2015 You Only Look Once: Unified, Real-Time Object Detection.
[16]. He, Kaiming & Gkioxari, Georgia & Dollar, Piotr & Girshick, Ross. 2017 Mask R-CNN. 2980-2988.
Cite this article
Huang,F. (2023). Computer vision model’s application in the current system on object detection tasks. Applied and Computational Engineering,4,1-6.
Data availability
The datasets used and/or analyzed during the current study will be available from the authors upon reasonable request.
Disclaimer/Publisher's Note
The statements, opinions and data contained in all publications are solely those of the individual author(s) and contributor(s) and not of EWA Publishing and/or the editor(s). EWA Publishing and/or the editor(s) disclaim responsibility for any injury to people or property resulting from any ideas, methods, instructions or products referred to in the content.
About volume
Volume title: Proceedings of the 3rd International Conference on Signal Processing and Machine Learning
© 2024 by the author(s). Licensee EWA Publishing, Oxford, UK. This article is an open access article distributed under the terms and
conditions of the Creative Commons Attribution (CC BY) license. Authors who
publish this series agree to the following terms:
1. Authors retain copyright and grant the series right of first publication with the work simultaneously licensed under a Creative Commons
Attribution License that allows others to share the work with an acknowledgment of the work's authorship and initial publication in this
series.
2. Authors are able to enter into separate, additional contractual arrangements for the non-exclusive distribution of the series's published
version of the work (e.g., post it to an institutional repository or publish it in a book), with an acknowledgment of its initial
publication in this series.
3. Authors are permitted and encouraged to post their work online (e.g., in institutional repositories or on their website) prior to and
during the submission process, as it can lead to productive exchanges, as well as earlier and greater citation of published work (See
Open access policy for details).