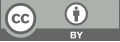
Research on Noise Robustness of Chinese Speech Recognition based on Bidirectional LSTM
- 1 School of Electronics Engineering and Computer Science, Peking University, Beijing, 100871, China
* Author to whom correspondence should be addressed.
Abstract
Automatic speech recognition (ASR) techniques are becoming more and more important in people’s daily life as a vital method of human-computer interaction. The ability to maintain a high recognition accuracy in noisy environments is the key for a model to be widely used in ASR. This article researches on the robustness against different kinds of background noise in Mandarin Chinese speech recognition and the baseline model used here is Bidirectional Long Short-Term Memory (BiLSTM). To compare the effects of different kinds of data augmentation method and different model structure, four common used data augmentation methods are applied in the process of training respectively and together, and two model methods, the CNNs and the attention mechanism, are combined with the baseline model, using the method of controlled experiment. After the model is trained, it will be tested within three kinds of background noise (car noise, café noise, white noise) to evaluate the anti-noise ability of different methods applied to the model. Among the four data augmentation methods, the method of random increased/decreased volume performed best in improving recognition accuracy, while the method of time-frequency masking increased Character Error Rate (CER) unexpectedly. As for the model methods, the CNNs performed better than the attention mechanism.
Keywords
deep learning, automatic speech recognition, Mandarin Chinese
[1]. Fan, H. H. (2017). Analysis and application of speech recognition technology. Electronics World, (17), 140.
[2]. Zhang, J. (2024). Application of artificial intelligence in speech recognition. Computer Knowledge and Technology, 20(17), 46–48.
[3]. Zhang, X. D., Zhang, Y. Y., Hu, H. D., et al. (2024). Research on speech recognition technology based on deep learning. Electronic Production, 32(16), 63–65.
[4]. Liu, Y., & Lian, M. M. (2024). Construction method of speech recognition system based on 1D convolutional neural network. Audio Engineering, 48(10), 77–79.
[5]. Wang, D., & Zhang, X. (2015). THCHS-30: A free Chinese speech corpus. arXiv.
[6]. Abdel-Hamid, O., Mohamed, A. R., Jiang, H., Deng, L., Penn, G., & Yu, D. (2014). Convolutional neural networks for speech recognition. IEEE/ACM Transactions on Audio, Speech, and Language Processing, 22(10), 1533–1545.
[7]. Bahdanau, D., Cho, K., & Bengio, Y. (2015). Neural machine translation by jointly learning to align and translate. Proceedings of the International Conference on Learning Representations (ICLR).
[8]. Park, D. S., Chan, W., Zhang, Y., Chiu, C.-C., Zoph, B., Cubuk, E. D., & Le, Q. V. (2019). SpecAugment: A simple data augmentation method for automatic speech recognition. Proceedings of the Interspeech 2019, 2613–2617.
Cite this article
Ji,Y. (2025). Research on Noise Robustness of Chinese Speech Recognition based on Bidirectional LSTM. Applied and Computational Engineering,145,57-63.
Data availability
The datasets used and/or analyzed during the current study will be available from the authors upon reasonable request.
Disclaimer/Publisher's Note
The statements, opinions and data contained in all publications are solely those of the individual author(s) and contributor(s) and not of EWA Publishing and/or the editor(s). EWA Publishing and/or the editor(s) disclaim responsibility for any injury to people or property resulting from any ideas, methods, instructions or products referred to in the content.
About volume
Volume title: Proceedings of the 3rd International Conference on Software Engineering and Machine Learning
© 2024 by the author(s). Licensee EWA Publishing, Oxford, UK. This article is an open access article distributed under the terms and
conditions of the Creative Commons Attribution (CC BY) license. Authors who
publish this series agree to the following terms:
1. Authors retain copyright and grant the series right of first publication with the work simultaneously licensed under a Creative Commons
Attribution License that allows others to share the work with an acknowledgment of the work's authorship and initial publication in this
series.
2. Authors are able to enter into separate, additional contractual arrangements for the non-exclusive distribution of the series's published
version of the work (e.g., post it to an institutional repository or publish it in a book), with an acknowledgment of its initial
publication in this series.
3. Authors are permitted and encouraged to post their work online (e.g., in institutional repositories or on their website) prior to and
during the submission process, as it can lead to productive exchanges, as well as earlier and greater citation of published work (See
Open access policy for details).